Self-Supervised Adversarial Learning for Domain Adaptation of Pavement Distress Classification
IEEE TRANSACTIONS ON INTELLIGENT TRANSPORTATION SYSTEMS(2024)
摘要
Pavement distress classification is crucial for the maintenance of highways. Although many methods for classifying pavement distress are available, they all assume that training and testing datasets are drawn from the same distribution. When we introduce a new unlabeled dataset with a different distribution, the performance of existing methods decreases considerably due to domain shift, motivating us to look beyond the supervised setting to utilize unlabeled datasets directly in training a model. Therefore, we develop a novel unsupervised domain adaptation (UDA) framework, namely, the Self-supervised Adversarial Network (SSAN) for the first time in this study to conduct multi-category pavement distress classification on an unlabeled target domain. In particular, SSAN leverages adversarial domain adaptation (ADA) thoughts to align the features of different domains. However, distress typically occupies a small section of high-resolution pavement images. Consequently, aligning features directly is unreasonable because the aligning procedure is still dominated by background features instead of foreground features, which are the most useful information for classification. Therefore, we design a pretext module, called Self-supervised Learning for the Target domain (SLT), to mine foreground information. To validate our method, we use two challenging pavement crack datasets, namely, the Chonqing University Bituminous Pavement Disease Detection (CQU-BPDD) and the Chongqing University Bituminous Pavement Multi-label Disease Detection (CQU-BPMDD) datasets. Moreover, extensive experiments demonstrate that SSAN outperforms state-of-the-art UDA methods.
更多查看译文
关键词
Pavement distress classification,adversarial domain adaptation,self-supervised learning,discriminative information
AI 理解论文
溯源树
样例
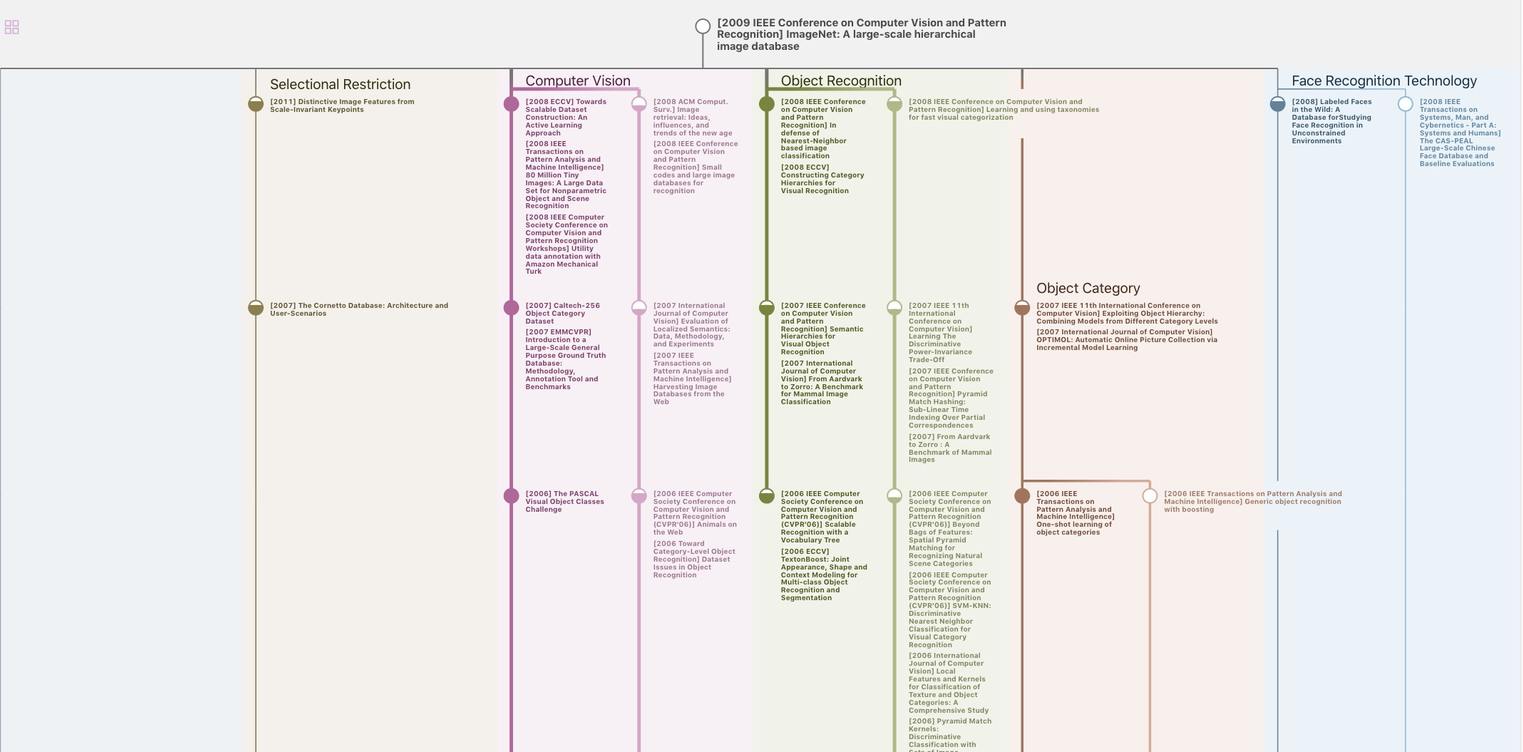
生成溯源树,研究论文发展脉络
Chat Paper
正在生成论文摘要