Unmasking AlphaFold: integration of experiments and predictions with a smarter template mechanism
bioRxiv (Cold Spring Harbor Laboratory)(2023)
摘要
Since the release of AlphaFold, large efforts have been undertaken to improve its predictions and integrate it in already existing pipelines for the determination of protein structures. Though a number of functionalities have been introduced, and results at the latest CASP edition showed a marked improvement in the prediction of multimers, AlphaFold’s capability of predicting large protein complexes is still limited, and integration with experimental data is not straightforward. In this study, we introduce AF_unmasked: a version of AlphaFold able to better take advantage of information from experimental structures to build high-quality protein assemblies, including very large protein complexes. We test AF_unmasked on difficult targets from the latest CASP edition as well as on large protein cryo-EM targets and show that this new approach enables generation of near-perfect structures even when little to no evolutionary information is available or when imperfect experimental structures are used as a starting point. We also show how incomplete structures can be filled in by AF_unmasked, a procedure called “structural inpainting”, and how this can give insights into protein dynamics. In summary, AF_unmasked represents an easy-to-use method with much improved capacity to efficiently integrate experiments and predictions to solve large protein complexes, without any need for computationally demanding re-training.
更多查看译文
关键词
predictions,experiments,template
AI 理解论文
溯源树
样例
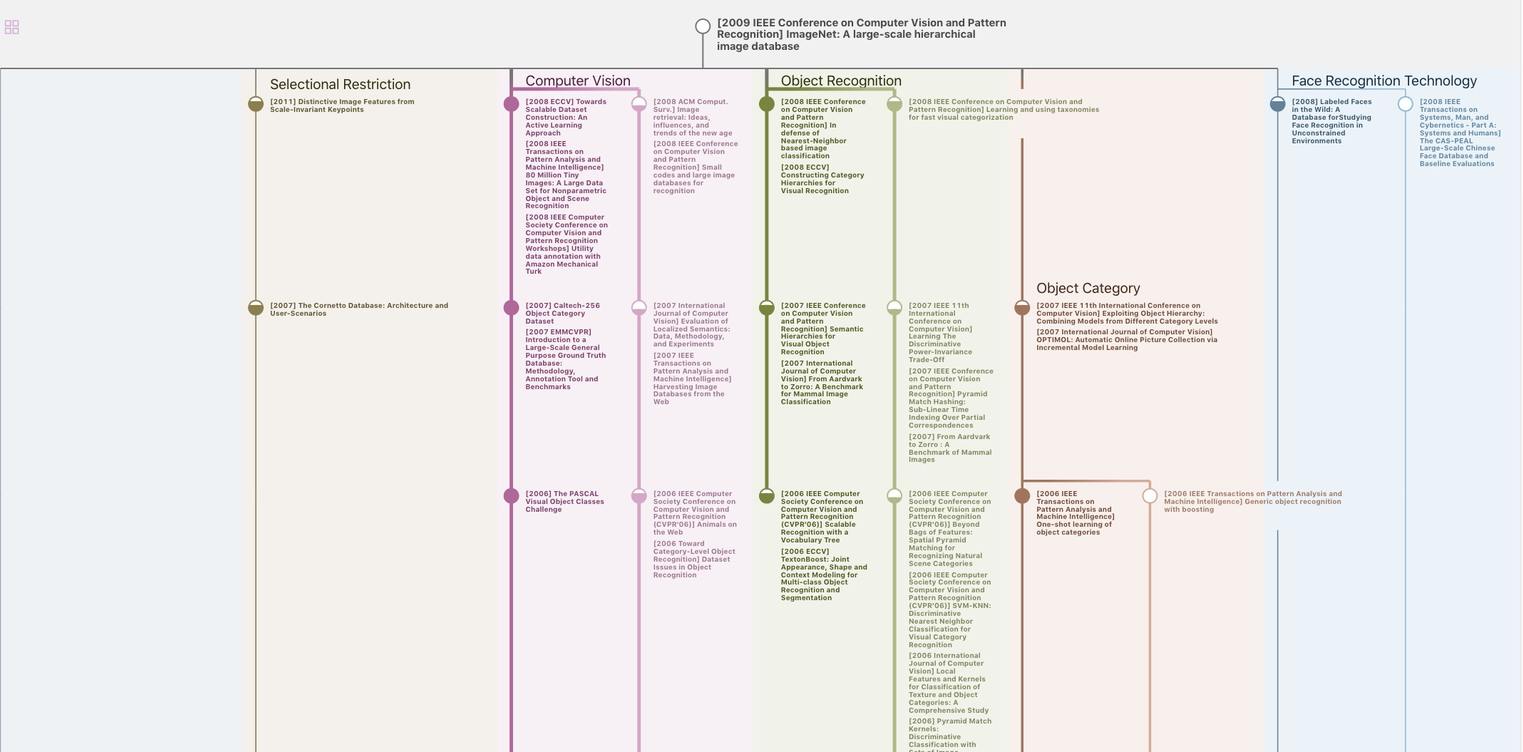
生成溯源树,研究论文发展脉络
Chat Paper
正在生成论文摘要