Genomic Selection for Meat Quality Traits Based on VIS/NIR Spectral Information1
Journal of Integrative Agriculture(2023)
摘要
The principle of genomic selection (GS) entails estimating breeding values (BVs) by summing all the SNP polygenic effects. The VIS/NIRS wavelength and abundance values can directly reflect the concentrations of chemical substances, and the measurement of meat traits by VIS/NIRS is similar to the processing of genomic selection data by summing all “polygenic effects” associated with spectral feature peaks. Therefore, it is meaningful to investigate the incorporation of VIS/NIRS information into GS models to establish an efficient and low-cost breeding model. In this study, we measured 6 meat quality traits in 359 Duroc×Landrace×Yorkshire pigs from Guangxi, China, and genotyped them with high-density SNP chips. According to the completeness of the information for the target population, we proposed four breeding strategies applied to different scenarios: I, only spectral and genotypic data exist for the target population; II, only spectral data exist for the target population; III, only spectral and genotypic data but with different prediction processes exist for the target population; and IV, only spectral and phenotypic data exist for the target population. The four scenarios were used to evaluate the GEBV accuracy by increasing the VIS/NIR spectral information. In the results of the 5-fold cross-validation, the genetic algorithm showed remarkable potential for preselection of feature wavelengths. The breeding efficiency of Strategies II, III, and IV was superior to that of traditional GS for most traits, and the GEBV prediction accuracy was improved by 32.2%, 40.8% and 15.5% respectively on average. Among them, the prediction accuracy of Strategy II for Fat (%) even improved by 50.7% compared to traditional GS. The GEBV prediction accuracy of Strategy I was nearly identical to that of traditional GS, and the fluctuation range was less than 7%. Moreover, the breeding cost of the four strategies was lower than that of traditional GS methods, with Strategy IV being the lowest as it ’did not require genotyping. Our findings demonstrate that GS methods based on VIS/NIRS data have significant predictive potential and are worthy of further research to provide a valuable reference for the development of effective and affordable breeding strategies.
更多查看译文
关键词
VIS/NIR,Genomic selection,GEBV,Machine learning,Pig,Meat quality
AI 理解论文
溯源树
样例
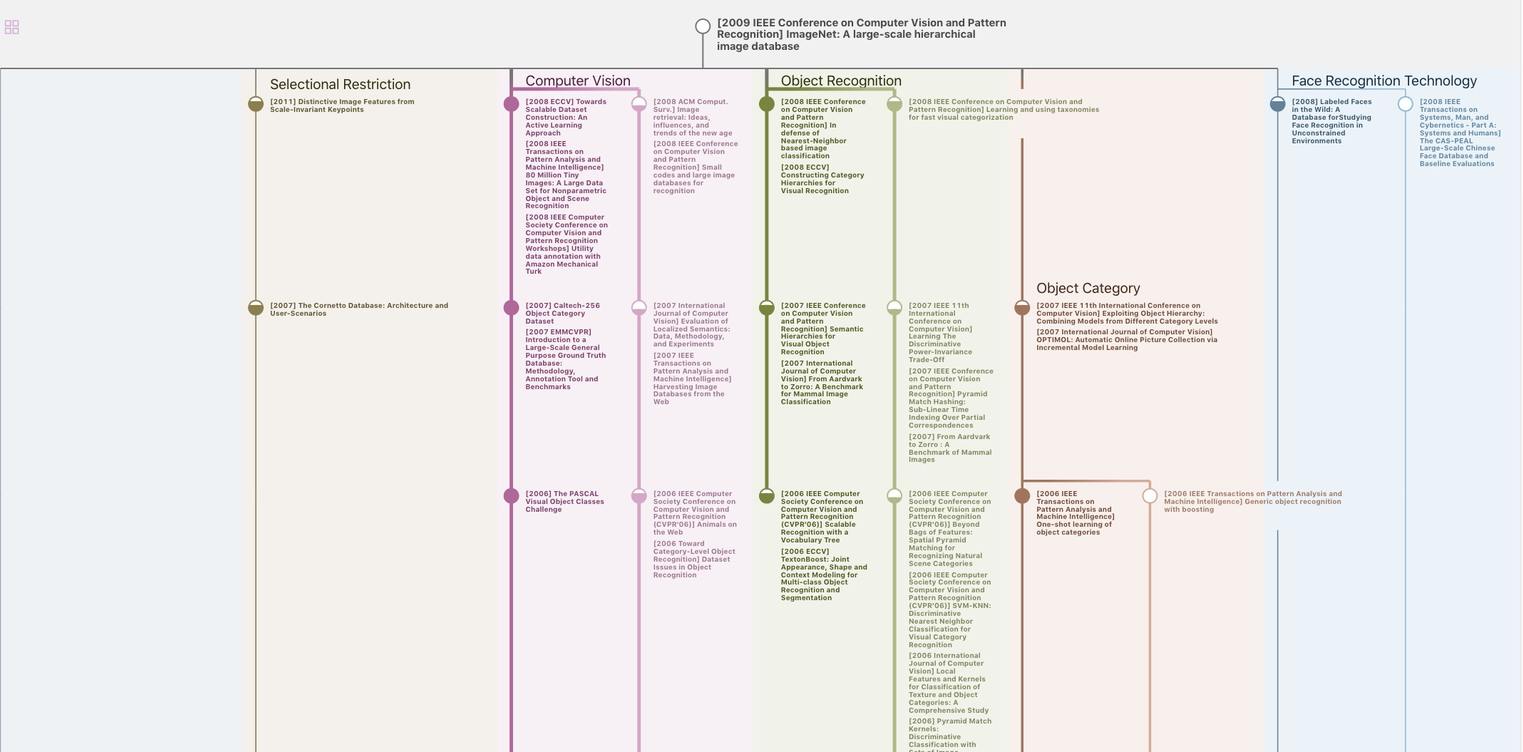
生成溯源树,研究论文发展脉络
Chat Paper
正在生成论文摘要