Design and Verification of Partial Power DC-DC Converter for Hydrogen Fuel Cell Locomotive
The Transactions of the Korean Institute of Electrical Engineers(2023)
摘要
Speech emotion recognition is a technology that identifies emotional states in human speech and plays a crucial role in enhancing Human Computer Interactions (HCI) more naturally and effectively. This technology supports the accurate understanding and appropriate response to human emotions through AI. In this study, we compare and analyze the performance of a speech emotion recognition model based on deep learning that utilizes the fusion of multiple features from speech signals. Various features such as bark-spectrum, mel-spectrum, Mel Frequency Cepstrum Coefficient (MFCC), and GammaTone Cepstrum Coefficient (GTCC) are extracted from the speech signal. Among these, two types of features are fused based on Canonical Correlation Analysis (CCA) methods to obtain a new single feature vector, which is then used as the input for the one-dimensional Convolutional Neural Network (1D-CNN) emotion recognition model. This fused feature contributes to enhancing the efficiency and accuracy of emotion recognition, and its performance is analyzed in comparison to the case of using only each feature. The performance of the model is evaluated using the AI-hub emotion classification dataset and the Korean speech emotion state classification dataset constructed by Chosun University. For both datasets, the multiple features level fusion through the CCA method improved the performance of voice emotion recognition compared to using single features.
更多查看译文
关键词
hydrogen,converter
AI 理解论文
溯源树
样例
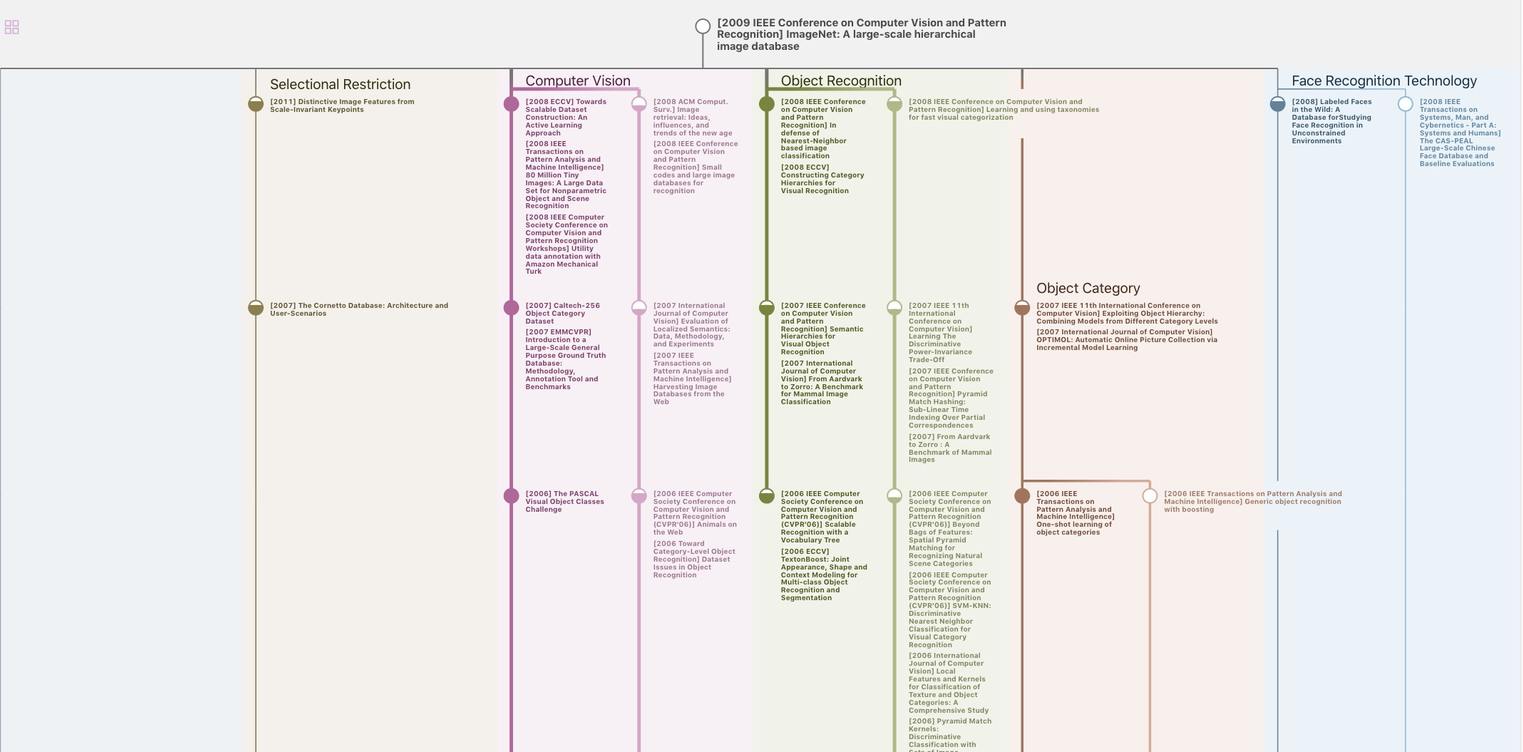
生成溯源树,研究论文发展脉络
Chat Paper
正在生成论文摘要