B-229 Benchmarking of Bioinformatics and Molecular Tools for Copy Number Variants Calling From Human Genome: A Detailed Look on Single Exons
Clinical Chemistry(2023)
摘要
Background Copy Number Variants (CNV) are deletions, duplications or insertions larger than 50 base pairs. They account for a large percentage of the normal genome variation and play major roles in human pathology. NGS bears the promise to allow concomitant exploration of CNV and smaller variants. However, accurately calling CNV from NGS data, especially targeted-NGS, remains a difficult computational task, for which a consensus is still lacking. Here, we aim to compare two bioinformatics approaches for CNV calling based on reads count [Dragen CNV caller and Normalized Reads Counts algorithm (NRC)] with legacy molecular tools for CNV detection such as MLPA and qPCR on a set of 20 polymorphic CNV of human genome. Methods DNA extracted from whole blood of 20 participants were enrolled in this validation. All samples were submitted to a clinical exome NGS targeted sequencing performed with lotus DNA library prep, xGEN hybridization capture, xGEN inherited disease panel (all from Integrated DNA Technologies) and sequenced using NextSeq-500 (Illumina). Twenty polymorphic CNV sites of human genome which overlap the exons included in the clinical exome were selected from NCBI dbVar curated common structural variants. Their median (min-max) minor allele frequency was 5% (1–63%). When intersected with regions included in clinical exome these CNV matched regions with 1 to 17 consecutive exons. One exon per CNV was the most prevalent condition (n = 10), followed by 2 exons (n = 4), 3 exons (n = 1), 4 exons (n = 3), 6 exons (n = 1), 8 exons (n = 1), 11 exons (n = 1), and 17 exons (n = 1). A single exon (region) of each included CNV were selected to be genotyped by the four tested methods: MLPA, qPCR, CNV caller implemented on Dragen enrichment v.4.0.3 (Illumina) and a laboratory-developed algorithm which detects CNV based on reads depth count of single exons normalized between samples from the same batch. Dragen was the exception for analysis of only single exons; its segmentation algorithm was not disable. The agreement between each compared method was calculated using absolute and relative frequency and measured using kappa statistics. MLPA was considered the reference method because of its known higher performance over qPCR for CNV calling. MLPA results were presented as copy numbers (CN). Results MLPA and qPCR fail to return results from 1 region each. MLPA called 24 losses with CN = 0, 71 losses with CN = 1, 260 no-CNV (CN = 2), 25 gains with CN = 3, and 0 gains with CN = 4. The CNV calling agreement of MLPA with other tested methods where: MLPA vs Dragen—341/380 (89.7%), Kappa = 0.66 (substantial agreement); MLPA vs NRC 366/380 (96.3%), Kappa = 0.92 (almost perfect agreement); MLPA vs qPCR 329/360 (91.4%), Kappa = 0.82 (almost perfect agreement). Conclusion We observed an overall good concordance between all tested methods when applied to a large number CNV to be interrogated (360–380 CNV callings). qPCR could validate most MLPA calls. Dragen was challenged by the fact that only polymorphic CNV were considered and showed substantial agreement with MLPA. MLPA and NRC showed the highest agreement suggesting that NRC could reliability confirm single exons CNV callings, as MLPA is extensively used to.
更多查看译文
关键词
copy number variants,human genome,bioinformatics
AI 理解论文
溯源树
样例
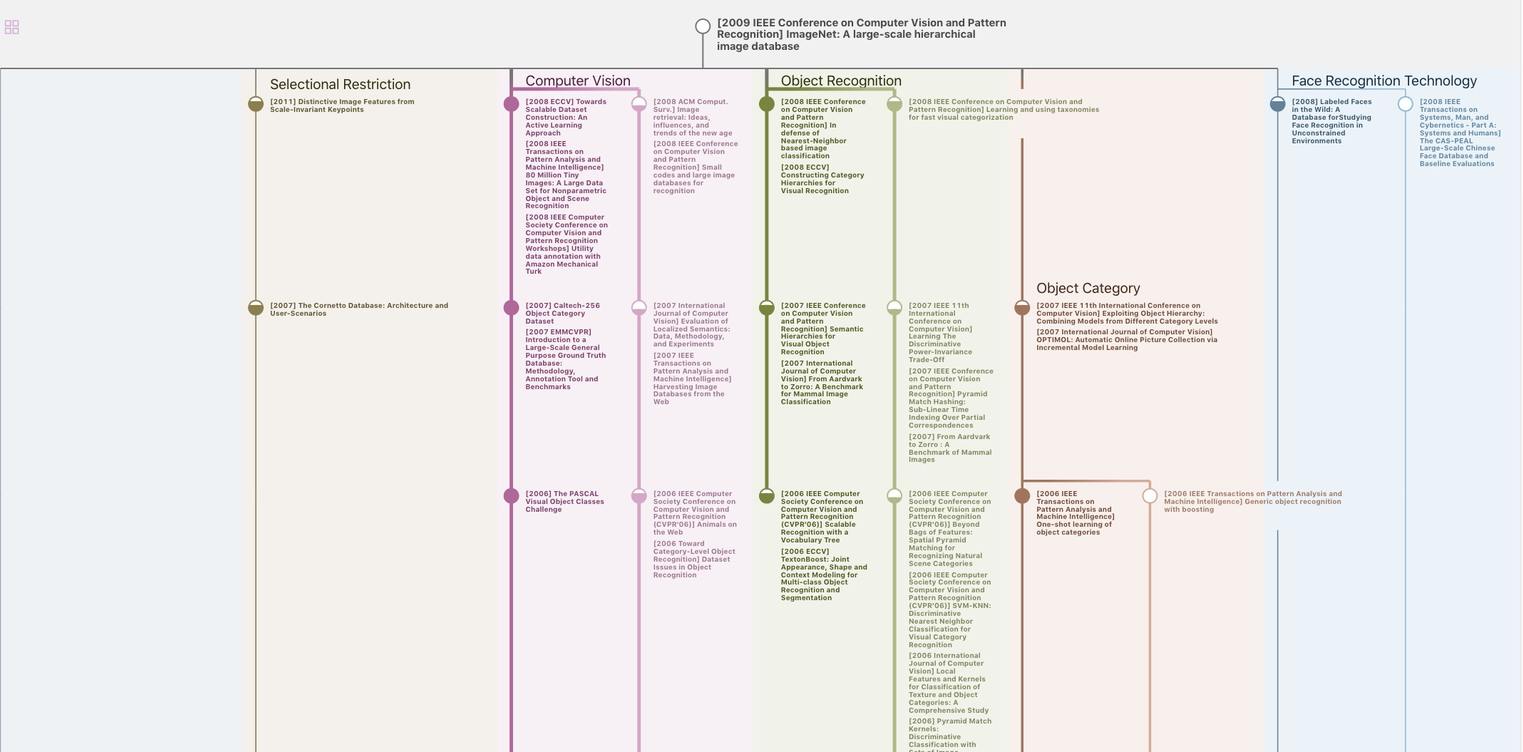
生成溯源树,研究论文发展脉络
Chat Paper
正在生成论文摘要