Time-Sensitive Index Future Trading Trend Prediction with Deep Learning methods
Research Square (Research Square)(2023)
摘要
Abstract Purpose- As the stock price’s fluctuation is nonlinear and greatly affected by environmental and industrial factors, stock prediction is extremely complicated and has various methods. This paper aims to find the optimal prediction model for predicting the Hang Seng stock market data from six main forecasting methods: ARIMA (Auto Regressive Integrated Moving Average), Artificial Neural Network (ANN), Convolutional Neural Network (CNN), Long short-term memory (LSTM), Gated Circulation Unit (GRU) and Conv1D-LSTM. Design/methodology/approach- In this project, the authors apply the open data of Hang Seng stock’s hour-by-hour closing prices to the above methods to establish forecasting models. Among them, hourly technical indicators (such as MA, EMA, and Momentum) are used in training neural network models as features. MSE and MAE methods are utilized to evaluate the discrepancy between the predicted and actual values. Finally, the accuracy of the buying decision (purchase or sell) is tested by the yield calculated by the actual closing price and the predicted closing price, respectively. Findings- The data collected from open data of the Hang Seng dataset from September 2019 to September 2020 contained hourly stock indexes, including closing price, opening price, high price, low price, volume, and other fundamental indicators. The total amount of data was 4608 (hours)× 26 (indexes) samples. The contribution of this paper to the existing knowledge is the evidence that the LSTM model has more advantages in forecasting and purchasing decisions compared with other models limited to the Hang Seng stock market. On this basis, the in-depth analysis demonstrates that the datasets multi-dimensionality and timeliness should be considered in the prediction model design. And the proposed architecture of deep learning models in this study could be modified to an expert system coupled with several models to explore the best approach to predicting a stock market's trading trend. Originality/value- The contribution of this paper to the existing knowledge is the evidence that the LSTM model has more advantages in forecasting and purchasing decisions compared with other models limited to the Hang Seng stock market. On this basis, the in-depth analysis demonstrates that the datasets multi-dimensionality and timeliness should be considered in the prediction model design. And the proposed architecture of deep learning models in this study could be modified to an expert system coupled with several models to explore the best approach to predicting a stock market's trading trend.
更多查看译文
关键词
deep learning,future,prediction,index,time-sensitive
AI 理解论文
溯源树
样例
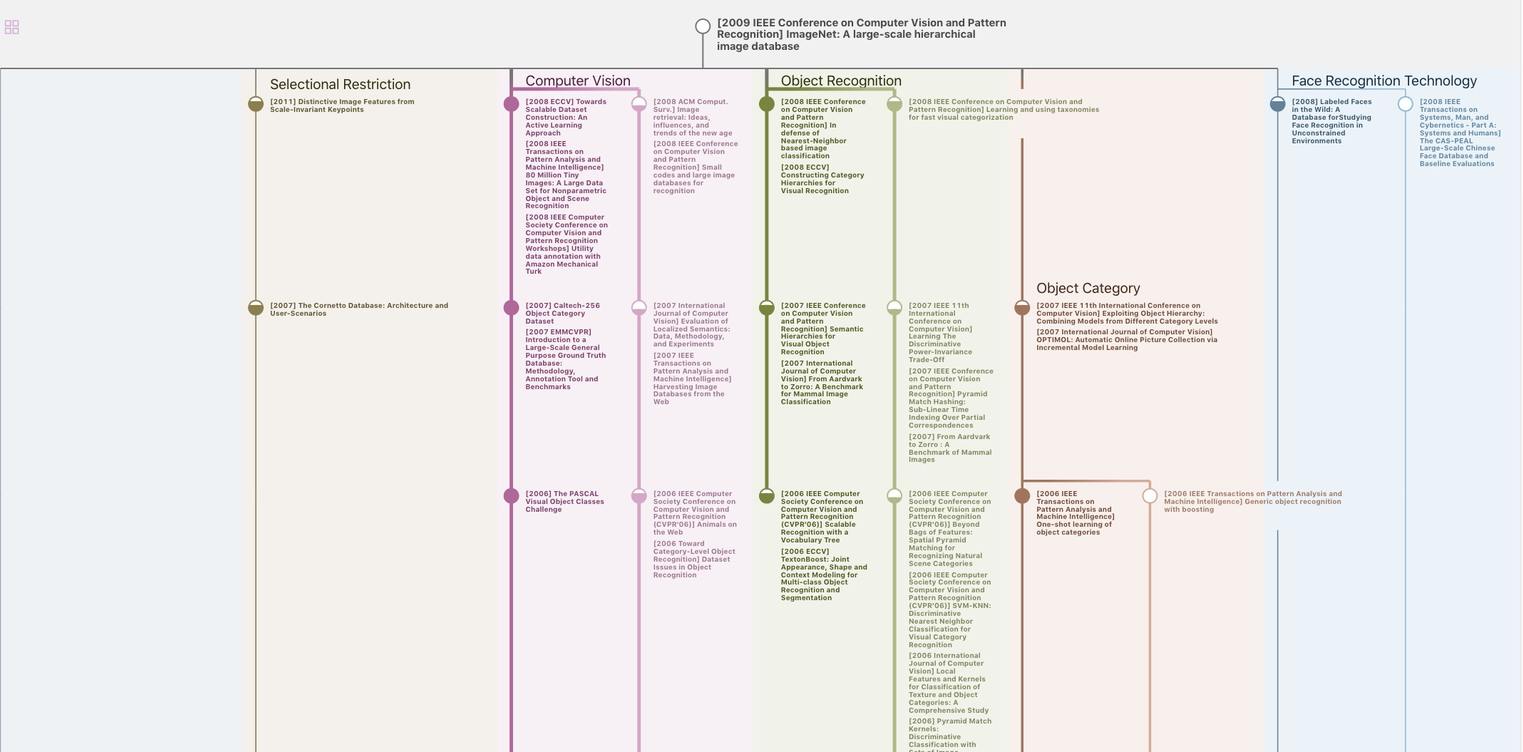
生成溯源树,研究论文发展脉络
Chat Paper
正在生成论文摘要