Machine Learning To Identify Heterogeneity Of Treatment Effect Of Intermediate-dose Vs Standard-dose Prophylactic Anticoagulation In The Inspiration Trial
Arteriosclerosis, Thrombosis, and Vascular Biology(2023)
摘要
Background: In the Intermediate vs Standard-Dose Prophylactic Anticoagulation in Critically-ill Patients With COVID-19: An Open Label Randomized Controlled Trial (INSPIRATION) trial, intermediate- compared with standard-dose prophylactic anticoagulation did not reduce a composite of venous/arterial thrombosis, treatment with extracorporeal membrane oxygenation or mortality. Whether machine learning (ML) implemented to predict individualized treatment effects (ITE) would identify variations in outcomes using baseline study variables is unknown. Methods: We used data from participants in the INSPIRATION trial. Patients were split into separate training (50%) and testing (50%) cohorts. In the training set, 24 different machine learning approaches to predict ITE were fit using baseline variables as predictors. The models were evaluated in the test set using the adjusted Qini index and the c-for-benefit, which illustrate how well the models identify patients deriving benefit or harm by the intervention. A value of 0 for the adjusted Qini index and 0.5 for c-for-benefit suggest the model did not identify any heterogeneous treatment effect. Results: A total of 562 patients were included. Baseline characteristics were balanced in the study groups. The single-model (s-learner) gradient boosted machine (GBM) model performed best, followed by the Tian transformation GBM model, and the causal forests model. Only the s-learner GBM model had an adjusted Qini >1, and only the s-learner GBM and Tian transformation GBM had c-for-benefit >0.50 ( Figure ). All other algorithms found no evidence of heterogeneous treatment effect. Conclusions: Of 24 machine learning models designed to predict ITE, only 2 found evidence of heterogeneous treatment effect. Considering multiple comparisons, these results should be considered as hypothesis-generating. The utility of ML models such as s-learner GBM for identification of ITE requires validation in additional trials.
更多查看译文
关键词
Thrombosis,Machine Learning,Clinical trials
AI 理解论文
溯源树
样例
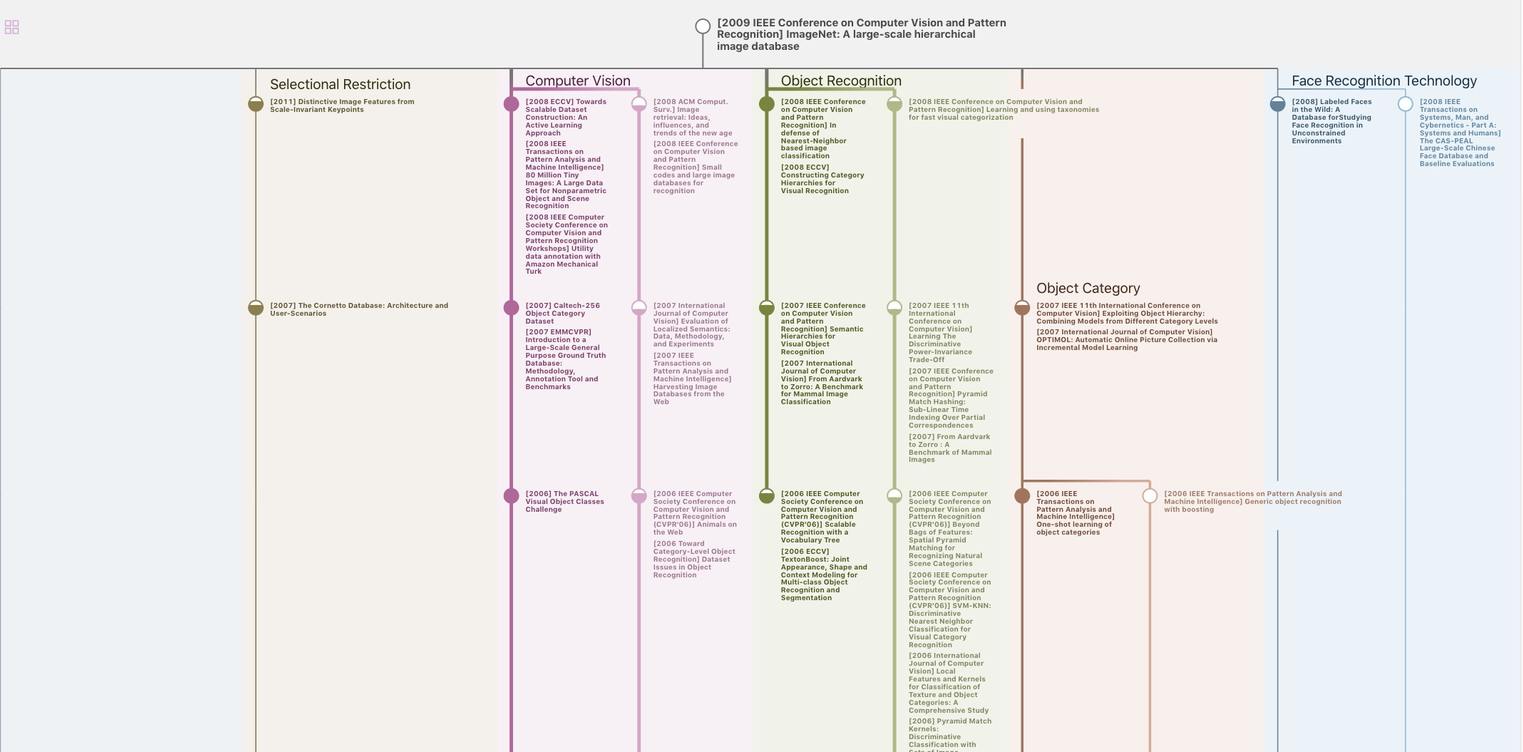
生成溯源树,研究论文发展脉络
Chat Paper
正在生成论文摘要