When is the next order? Nowcasting channel inventories with Point-of-Sales data to predict the timing of retail orders
EUROPEAN JOURNAL OF OPERATIONAL RESEARCH(2024)
摘要
Slow -moving goods are common in many retail settings and occupy a vast part of retail shelves. Since stores sell these products irregularly and in small quantities, the replenishing distribution center may only place batched orders with manufacturers every few weeks. While order quantities are often fixed, the challenge for manufacturers facing such intermittent demand is to forecast the order timing. In this paper, we explore the value of Point -of -Sales (PoS) data to improve a food manufacturer's order timing forecast for slow -moving goods. We propose an inventory modeling approach that uses the last order, PoS data from retail stores, and the expected lead time demand to estimate the retailer's channel inventory. With this dynamic estimate, we can 'nowcast' the retailer's inventory and predict his next order. To illustrate our methodology, we first conduct an experimental simulation and compare our results to a Croston variant and a moving average model. Next, we validate our approach with empirical data from a small German food manufacturer that serves a grocery retailer with a central distribution center and 53 hypermarkets. We find that, on average, our approach improves the accuracy of order -timing predictions by 10-20 percent points. We overcome a shrinkage -induced bias by incorporating an inventory correction factor. Our approach describes a new way of utilizing PoS data in multi -layered distribution networks and can complement established forecasting methods such as Croston. Particular applications arise when the order history is short (e.g., product launch) or represents a bad predictor for future demand (e.g., during COVID-19).
更多查看译文
关键词
Forecasting,Point-of-Sales,Information sharing,Intermittent demand,Food retailing
AI 理解论文
溯源树
样例
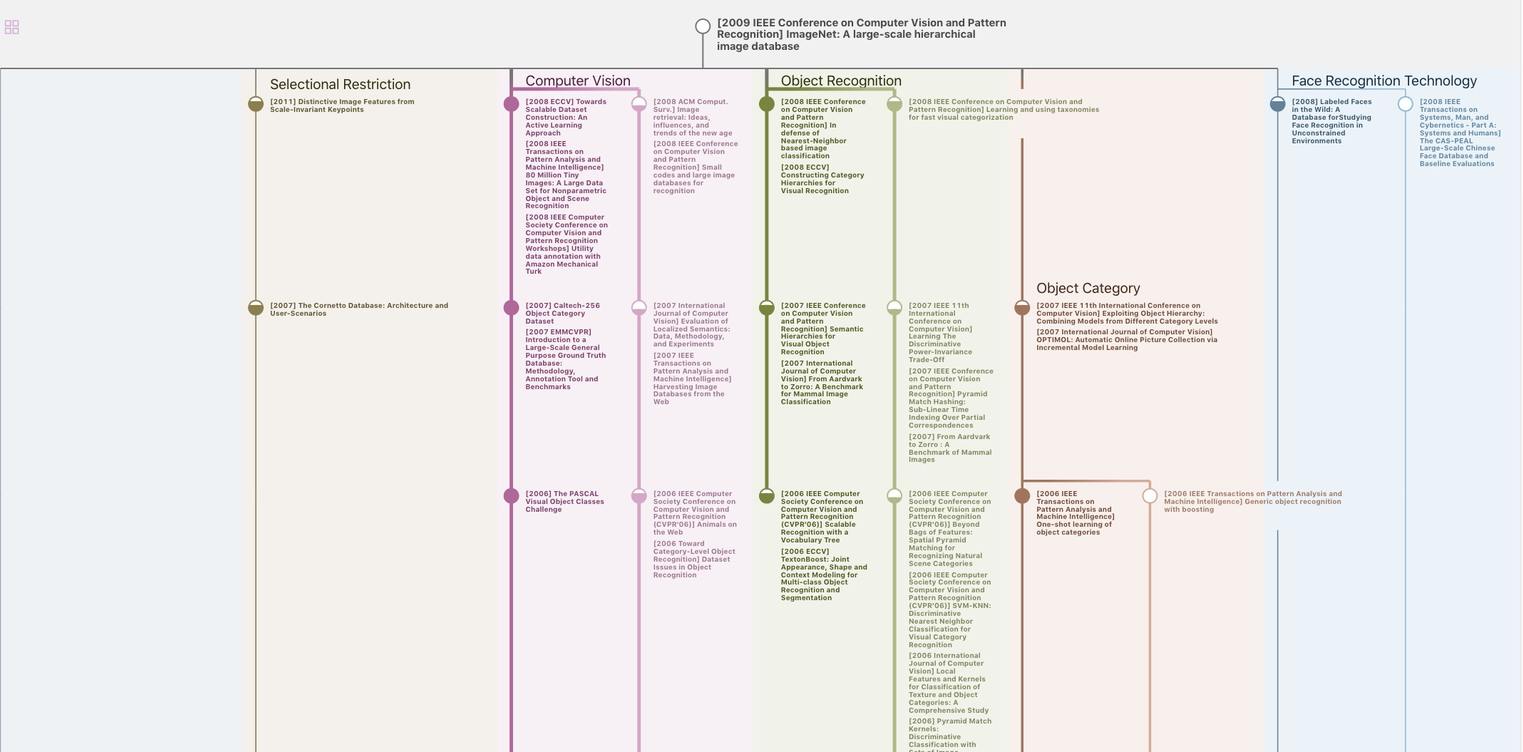
生成溯源树,研究论文发展脉络
Chat Paper
正在生成论文摘要