Can machine learning provide preoperative predictions of biological hemostasis after extracorporeal circulation for cardiac surgery?
The Journal of Thoracic and Cardiovascular Surgery(2023)
摘要
The goal of this study was to improve decision-making regarding the transfusion of patients at the end of extracorporeal circulation for cardiac surgery through machine learning predictions of the evolution of platelets counts, prothrombin ratio and fibrinogen assay.Prospective data with information about patient preoperative biology and surgery characteristics were collected at Institut Mutualiste Montsouris Hospital (Paris, France) for ten months (n = 598). For each outcome of interest, instead of arbitrarily choosing one machine learning algorithm, we trained and tested a variety of algorithms together with the super learning algorithm, a state-of-the-art ensemble method that aggregates all the predictions and selects the best performing algorithm (total: 137 algorithms). We considered the top performing algorithms and compared them to more standard and interpretable multivariable linear regression models. All algorithms were evaluated through their root mean squared error (RMSE), a measure of the average difference between true and predicted values.The RMSE of the top algorithms for predicting the difference between pre and postoperative platelet counts, prothrombin ratio and fibrinogen assay were 38.27 x10e9 g/l, 8.66% and 0.44 g/l respectively. The linear models had similar performances.Our machine learning algorithms accurately predicted prothrombin ratio and fibrinogen assay and less accurately platelet counts. As such, our models could provide an aid-decision tool for the anesthetist in the operating room; future clinical trials addressing this hypothesis are warranted.
更多查看译文
关键词
preoperative predictions,extracorporeal circulation,cardiac surgery,biological hemostasis
AI 理解论文
溯源树
样例
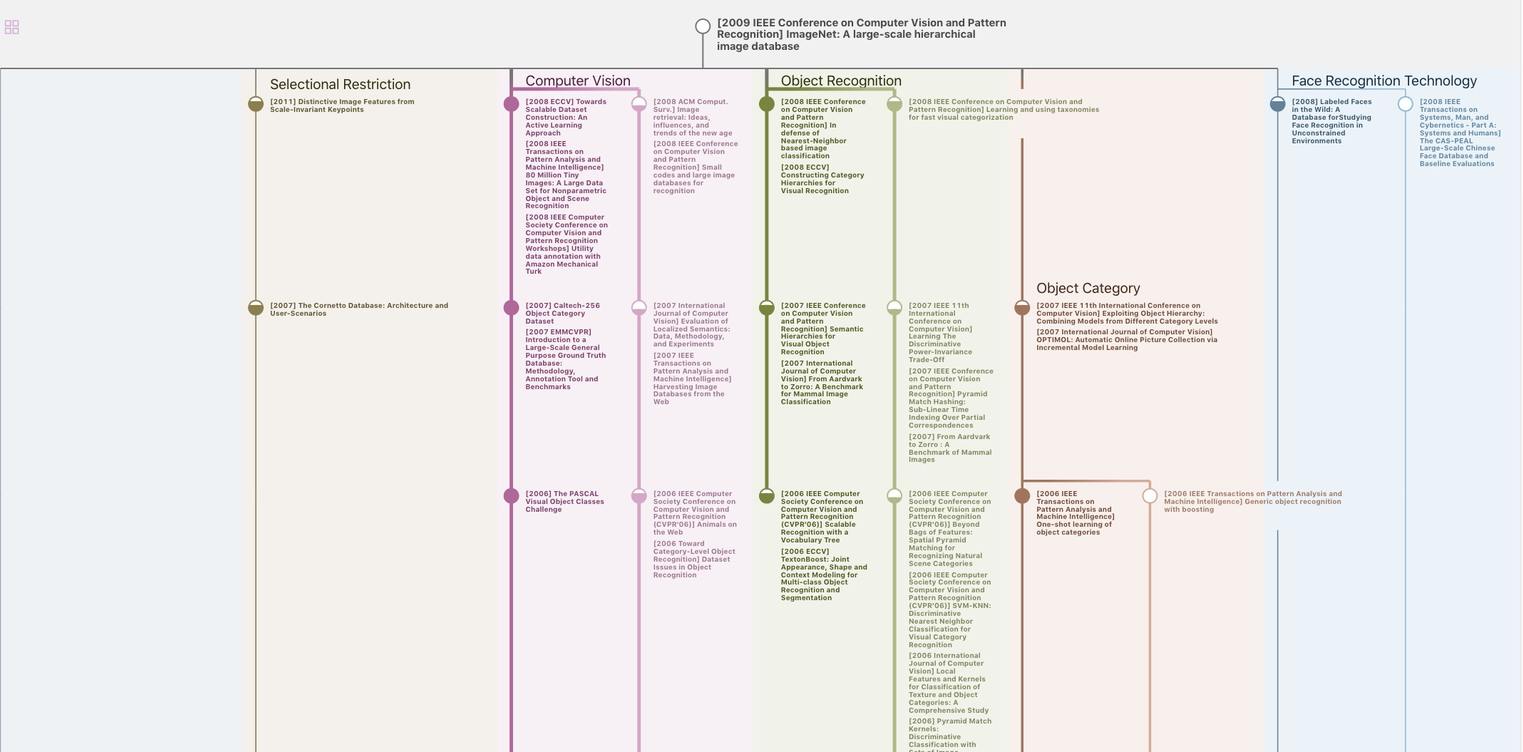
生成溯源树,研究论文发展脉络
Chat Paper
正在生成论文摘要