Enhancing hourly heat demand prediction through artificial neural networks: A national level case study
ENERGY AND AI(2024)
摘要
Meeting the goal of zero emissions in the energy sector by 2050 requires accurate prediction of energy consumption, which is increasingly important. However, conventional bottom -up model -based heat demand forecasting methods are not suitable for large-scale, high -resolution, and fast forecasting due to their complexity and the difficulty in obtaining model parameters. This paper presents an artificial neural network (ANN) model to predict hourly heat demand on a national level, which replaces the traditional bottom -up model based on extensive building simulations and computation. The ANN model significantly reduces prediction time and complexity by reducing the number of model input types through feature selection, making the model more realistic by removing non -essential inputs. The improved model can be trained using fewer meteorological data types and insufficient data, while accurately forecasting the hourly heat demand throughout the year within an acceptable error range. The model provides a framework to obtain accurate heat demand predictions for largescale areas, which can be used as a reference for stakeholders, especially policymakers, to make informed decisions.
更多查看译文
关键词
Heating demand in buildings,National level forecast,Feature selection,Machine learning,Artificial neural network
AI 理解论文
溯源树
样例
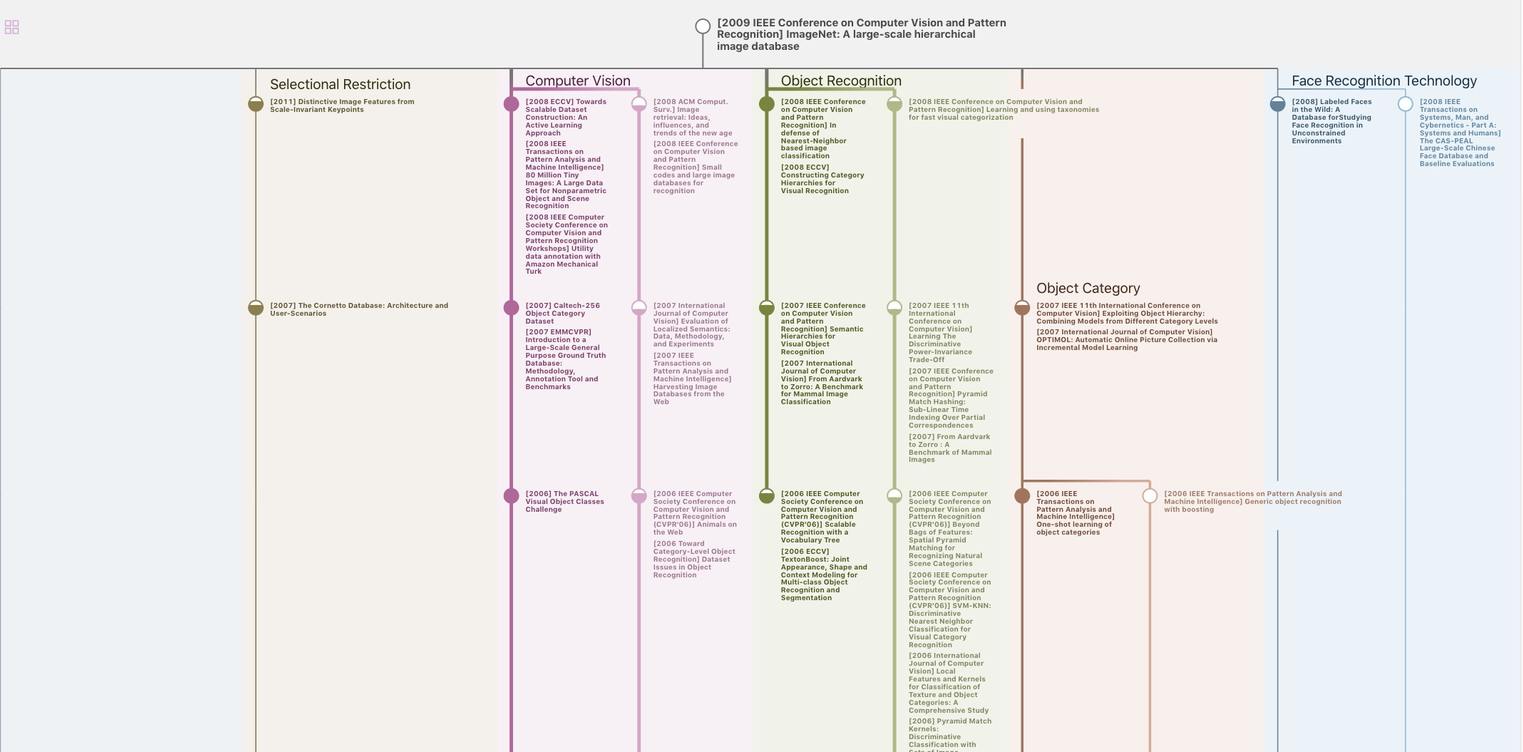
生成溯源树,研究论文发展脉络
Chat Paper
正在生成论文摘要