Global-Local Association Discrepancy for Multivariate Time Series Anomaly Detection in IIoT
IEEE INTERNET OF THINGS JOURNAL(2024)
摘要
Detecting anomalies in multivariate time series (MTS) data collected from industrial Internet of Things (IIoT) systems is essential for a variety of applications, including smart manufacturing. Existing methods typically learn local spatiotemporal representations from nearby time points and neighboring nodes to reconstruct or predict sensor data. However, these local representations are insufficient to model the complex nonlinear topological relationships and dynamic temporal patterns of IIoT systems, which often results in a high-false alarm rate. To address this issue, we propose a new MTS anomaly detection framework called GLAD, which is based on the global-local association discrepancy. The key concept is to detect anomalies based on the difference between the global and local spatiotemporal associations of each data sample, as the association distribution of each data sample provides a more informative description. Specifically, we introduce a Gumbel-Softmax-based graph structure learning strategy to capture the global topological connections from data. Based on the topological graph structure, we utilize a graph attention network (GAT) and transformer to extract both the global and local spatiotemporal associations of each data sample. Finally, we leverage the global-local association discrepancy to effectively detect anomalies from normal data samples. Extensive experiments on five real-world data sets demonstrate the superiority of GLAD over other state-of-the-art methods.
更多查看译文
关键词
Anomaly detection,Industrial Internet of Things,Time series analysis,Transformers,Predictive models,Data models,Training,graph attention network (GAT),Industrial Internet of Things (IIoT),multivariate time series (MTS),transformer
AI 理解论文
溯源树
样例
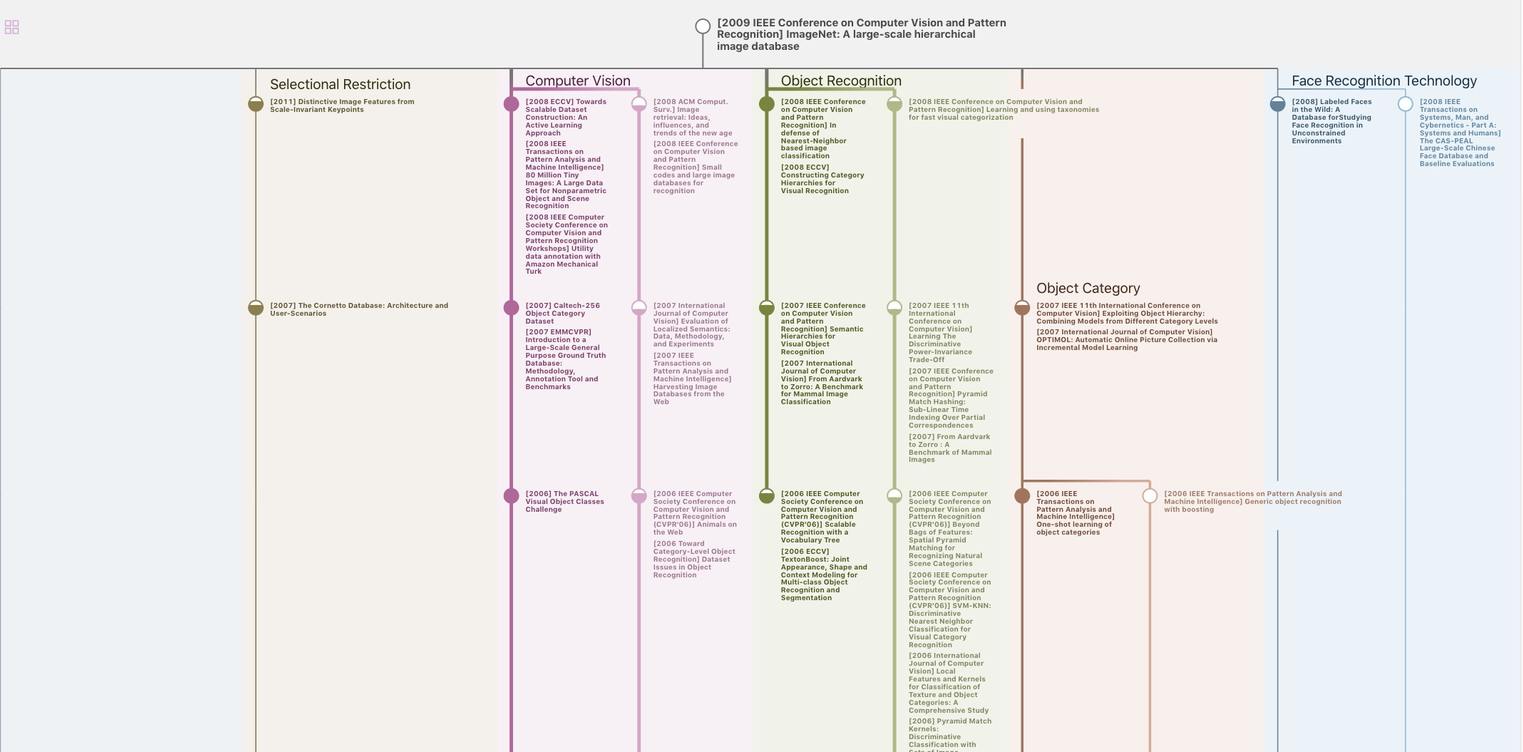
生成溯源树,研究论文发展脉络
Chat Paper
正在生成论文摘要