Flexible product quantization for fast approximate nearest neighbor search
Multimedia Tools and Applications(2023)
摘要
Product quantization (PQ) is an effective solution to approximate nearest neighbor (ANN) search. The idea of PQ is to decompose the space into Cartesian product of several low-dimensional subspaces and quantize each subspace separately. Database vectors are represented by short codes with different length composed of their subspace quantization indices. A vector is encoded to a short code consisting of its subspace quantization indices. PQ allows to generate a large size codebook with very low memory and time cost, and the selection of the number M of sub-vectors is the most essential and important problem, but is easily neglected. Motivated by this observation, we propose a method named Flexible Product quantization (FPQ) to optimize PQ-based methods that fix M for all vectors. FPQ achieves flexible M by concatenating the sub-vector pairs through constrains on quantization distortion. The concatenating operation is implemented individually among different database vectors and can lead to far less additions in the search process. Different from existing PQ-based fast search methods, this operation of our proposed FPQ is to reduce the computational complexity for searching each single database vector, which provides a new perspective to better accelerate ANN search. Experimental results show that our method for product quantization based methods can significantly reduce nearly 25 % computational complexity by using appropriate parameters, while the search accuracy can be guaranteed.
更多查看译文
关键词
Approximate nearest neighbor search,Product quantization,The number of sub-vectors,Sub-vector concatenation,Flexible product quantization
AI 理解论文
溯源树
样例
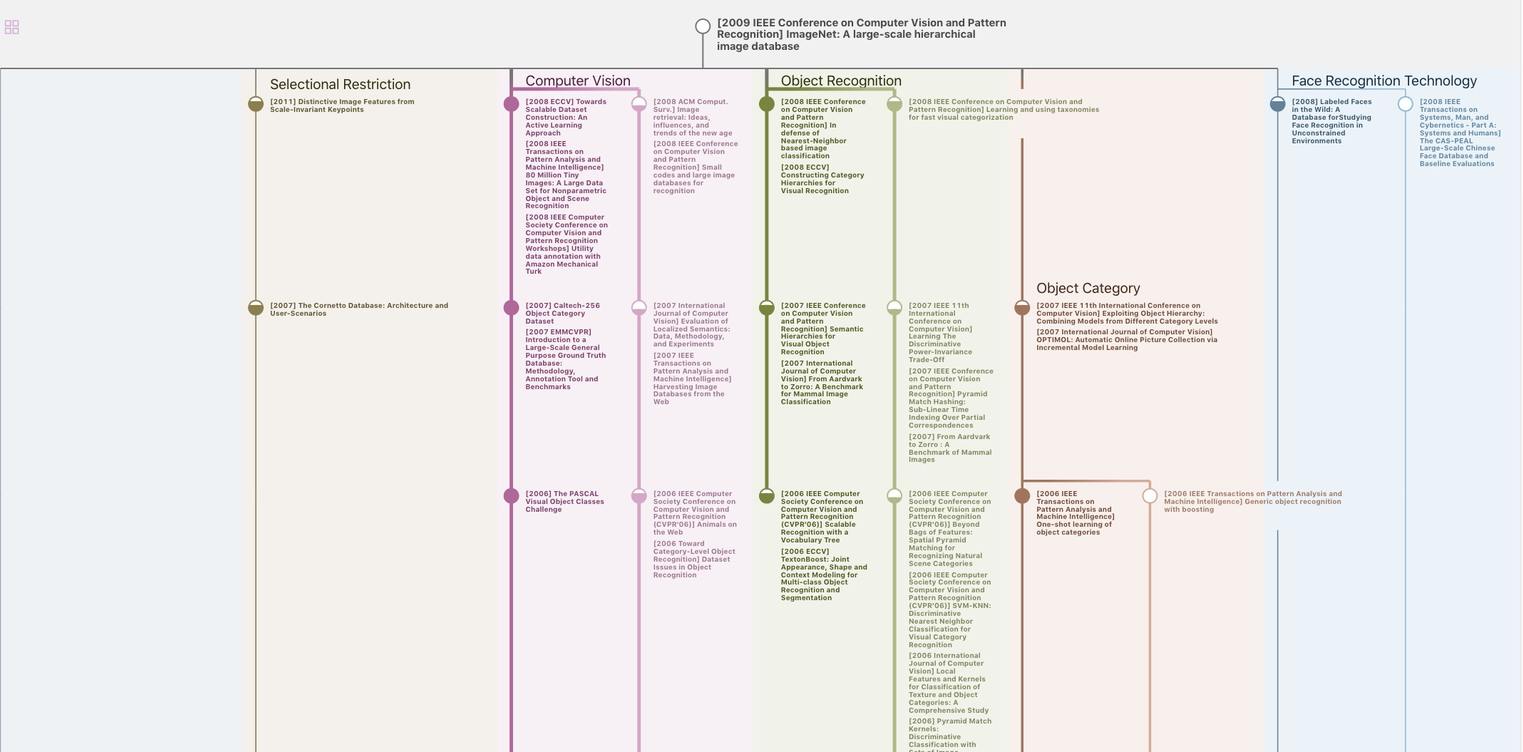
生成溯源树,研究论文发展脉络
Chat Paper
正在生成论文摘要