GOFL: An Accurate and Efficient Federated Learning Framework Based on Gradient Optimization in Heterogeneous IoT Systems
IEEE INTERNET OF THINGS JOURNAL(2024)
摘要
Federated learning (FL) is designed for training models using data distributed across multiple Internet of Things (IoT) devices or servers, reducing data transfer overhead and ensuring data security. However, the decentralization and diversity of IoT devices introduce statistical and system heterogeneity, which can lead to unstable model training and even system crashes. Although many studies attribute performance issues to client-drift caused by this heterogeneity, there is a lack of insight into how different forms of heterogeneity impact local model gradient variations and model convergence. In this article, we investigate model gradient distribution characteristics in heterogeneous training. We find that the challenge is not solely due to client-drift but is also closely linked to a high degree of model overfitting, which negatively affects local model training and equilibrium convergence. To address this challenge, we introduce an efficient framework called gradient optimization with FL (GOFL). First, GOFL incorporates the federated gradient normalization (FGN) technique to maintain gradient distribution consistency while mitigating client-drift stemming from heterogeneity. We also highlight the benefits of FGN in reducing local model overfitting and improving convergence. Second, GOFL introduces the federated device aggregation (FDA) strategy, a critical addition to FGN. It adaptively guides device selection and aggregation based on device contributions, ensuring a more balanced training approach in the face of system heterogeneity. The experimental results demonstrate that GOFL achieves state-of-the-art training accuracy while reducing the number of training rounds. In particular, it improves the accuracy of the classical FL framework FedAvg by 30.57% and reduces the number of convergence rounds by 5.17 times.
更多查看译文
关键词
Training,Optimization,Internet of Things,Biological system modeling,Data models,Servers,Federated learning,Edge intelligence systems,federated learning (FL),heterogeneous distributed training,Internet of Things (IoT) devices
AI 理解论文
溯源树
样例
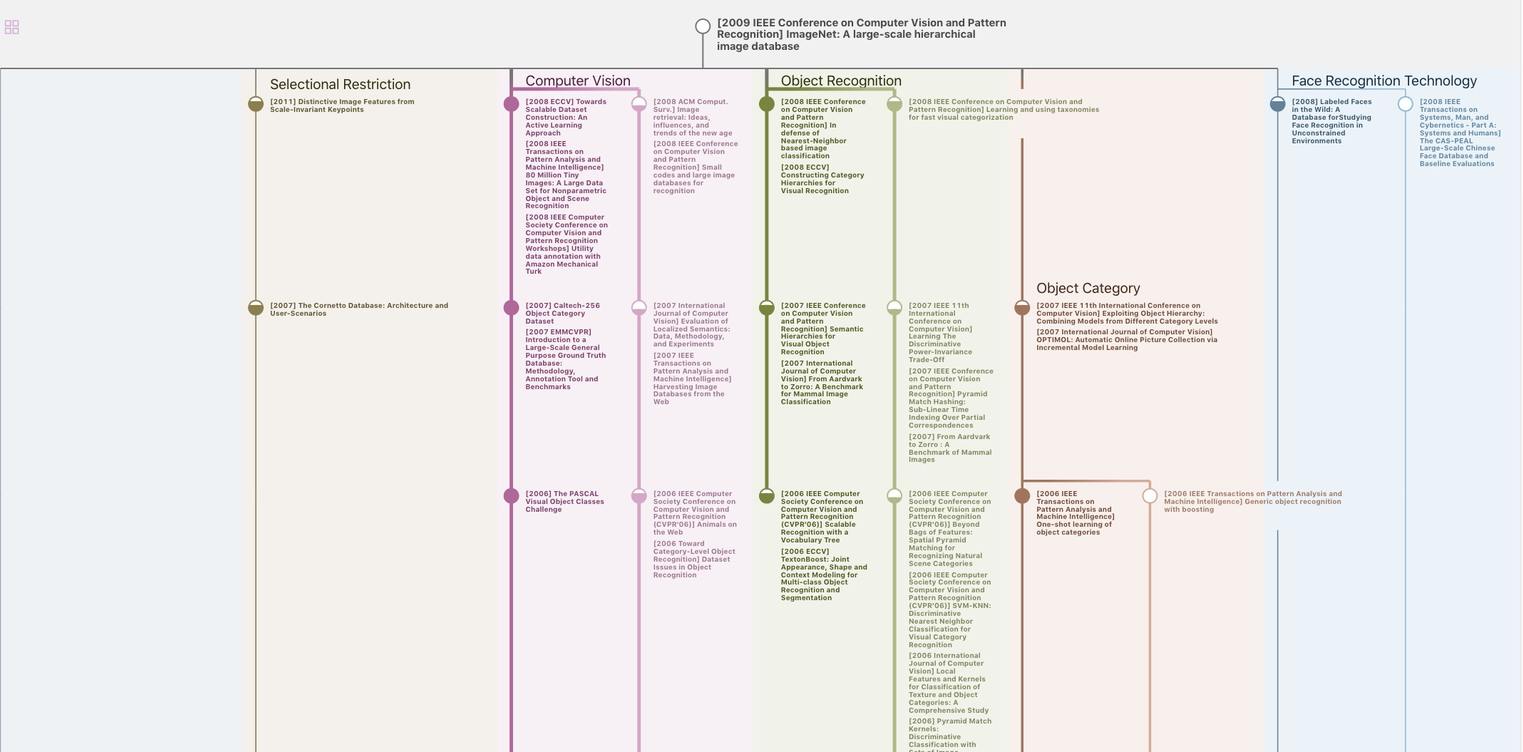
生成溯源树,研究论文发展脉络
Chat Paper
正在生成论文摘要