Performance of Evolutionary Optimized Machine Learning for Modeling Total Organic Carbon in Core Samples of Shale Gas Fields
Petroleum(2023)
Abstract
Rock samples' TOC content is the best indicator of the organic matter in source rocks. The origin rock samples’ analysis is used to calculate it manually by specialists. This method requires time and resources because it relies on samples from many well intervals in source rocks. Therefore, research has been done to aid this effort. Machine learning algorithms can estimate total organic carbon instead of well logs and stratigraphic studies. In light of these efforts, the current work present a study on automating the total organic carbon estimation using machine learning approaches improved by an evolutionary methodology to give the model flexibility and precision. Genetic algorithms, differential evolution, particle swarm optimization, grey wolf optimization, artificial bee colony, and evolution strategies were used to improve machine learning models to predict TOC. The six metaheuristics were integrated into four machine learning methods: extreme learning machine, elastic net linear model, linear support vector regression, and multivariate adaptive regression splines. Core samples from the YuDong-Nan shale gas field, located in the Sichuan basin, were used to evaluate the hybrid strategy. The findings show that combining machine learning models with an evolutionary algorithms in a hybrid fashion produce flexible models that accurately predict TOC. The results show that, independent of the metaheuristic used to guide the model selection, optimized extreme learning machines attained the best performance scores according to six metrics. Such hybrid models can be used in exploratory geological research, particularly for unconventional oil and gas resources.
MoreTranslated text
Key words
Total organic carbon,Hybrid models,Automated machine learning,Evolutionary algorithms,Geology
AI Read Science
Must-Reading Tree
Example
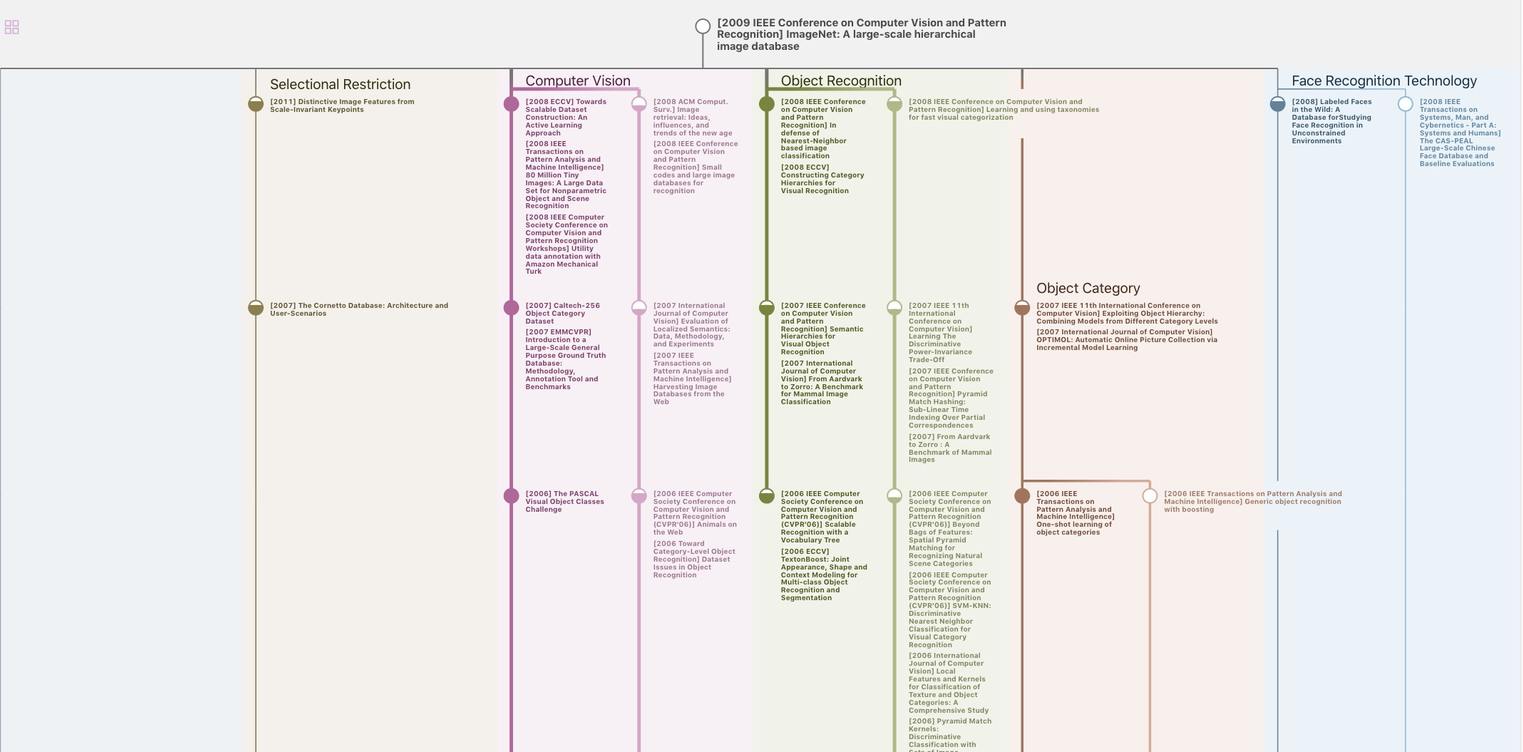
Generate MRT to find the research sequence of this paper
Chat Paper
Summary is being generated by the instructions you defined