Predicting compound activity from phenotypic profiles and chemical structures
Nature Communications(2023)
摘要
Predicting assay results for compounds virtually using chemical structures and phenotypic profiles has the potential to reduce the time and resources of screens for drug discovery. Here, we evaluate the relative strength of three high-throughput data sources—chemical structures, imaging (Cell Painting), and gene-expression profiles (L1000)—to predict compound bioactivity using a historical collection of 16,170 compounds tested in 270 assays for a total of 585,439 readouts. All three data modalities can predict compound activity for 6–10% of assays, and in combination they predict 21% of assays with high accuracy, which is a 2 to 3 times higher success rate than using a single modality alone. In practice, the accuracy of predictors could be lower and still be useful, increasing the assays that can be predicted from 37% with chemical structures alone up to 64% when combined with phenotypic data. Our study shows that unbiased phenotypic profiling can be leveraged to enhance compound bioactivity prediction to accelerate the early stages of the drug-discovery process.
更多查看译文
关键词
compound activity,phenotypic profiles
AI 理解论文
溯源树
样例
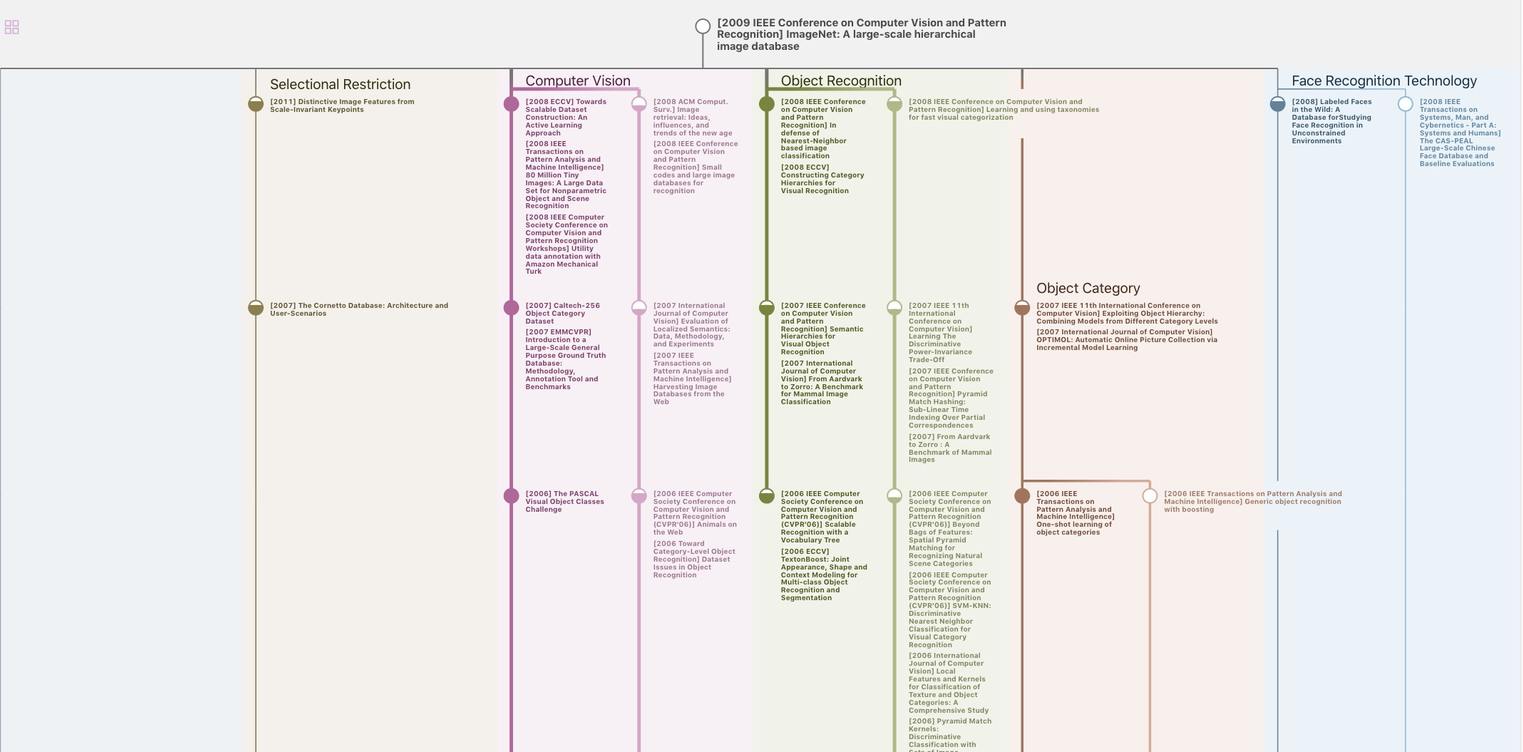
生成溯源树,研究论文发展脉络
Chat Paper
正在生成论文摘要