Prognostic value of deep learning based mitotic count in hormonal receptor- and HER2-positive breast cancer
Research Square (Research Square)(2023)
摘要
Abstract The discovery of molecular subtypes has corroborated the heterogeneity of breast cancers. But in clinical routine, treatment selection relies on measuring the aggressiveness of the tumor via histopathology, which is routinely based on grading, a prognostic yet laborious, poorly reproducible, and subjective procedure defined more than 30 years ago, before molecular subtypes existed. In this paper, we present an interpretable deep learning-based computer-aided diagnosis method to automate mitotic count , one of the components of grading; the method complies with current guidelines and automates hot-spot finding on full digital whole-slide images of routinely prepared pathologic slides stained with hematoxylin and eosin. We use the computer model to query the current “one-size-fits-all” mitotic count procedure by investigating subtype-specific prognostic value of mitotic count using an external independent large-scale multicentric dataset of 1,709 breast cancers. We show that for human epidermal growth factor-2 (HER2) positive tumors, mitotic count was not prognostic. In hormone-receptor positive/HER2 negative cancers, an average mitotic count density different from clinically used cut offs predicted worse recurrence free survival in multivariable analysis, adjusted for known clinical prognosticators. We provided some first experimental evidence that the prognostic role of mitotic count should be reviewed.
更多查看译文
关键词
breast cancer,deep learning,mitotic count,prognostic value
AI 理解论文
溯源树
样例
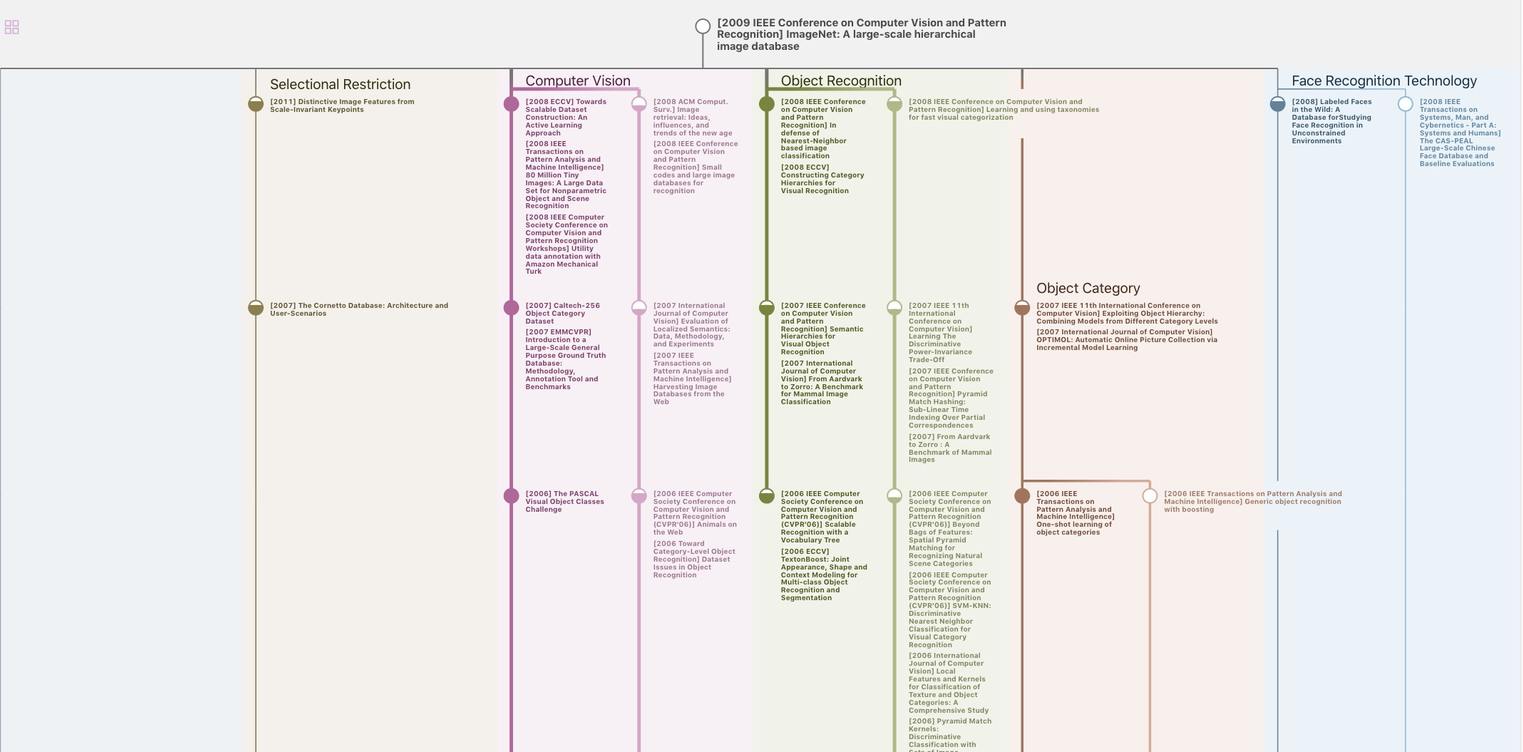
生成溯源树,研究论文发展脉络
Chat Paper
正在生成论文摘要