Causal reasoning modeling (CRM) for rivers’ runoff behavior analysis and prediction
Elsevier eBooks(2023)
摘要
Hydrological processes are experimenting an unprecedented shift toward increasing variability on a global and local scale. Therefore, water resource management requires the proper use and management of a great range and amount of data sources, where climatic and hydrological variables are essential. The uncertainty characterization and quantification of hydrologic models basically produced for the random nature of hydrological processes is largely based on probabilistic method. This chapter is focused on the development and usage of a technique called Causal Reasoning (CR) that uses probability to deal with the uncertainty. In this sense, randomness and variability of hydrological processes are incorporated and quantified by means of a type of CR modeling which is called Bayesian Causal Modeling (BCM) using the conditional probability to compute the influence among the variables. This technique has been successfully applied for modeling and predicting the general runoff behavior (annual time scale). Also, the evaluation of double influence of space and time (spatiotemporal) on the runoff behavior is currently being investigated through the design of CRM models heavily populated from very large datasets with thousands of multidirectional observations Furthermore, there are currently several developments on the modeling and predicting of unsteady time series (monthly, daily) and sudden hydrological processes (hourly, minute). This chapter provides the insights of a long-term research activity that started back in 2005. Over this time, this general methodology has been evolved, improved, and expanded, aimed to provide a useful modeling approach and tools largely for the advanced description and prediction of river’s flow behavior.
更多查看译文
关键词
runoff behavior analysis,causal reasoning modeling,rivers,crm
AI 理解论文
溯源树
样例
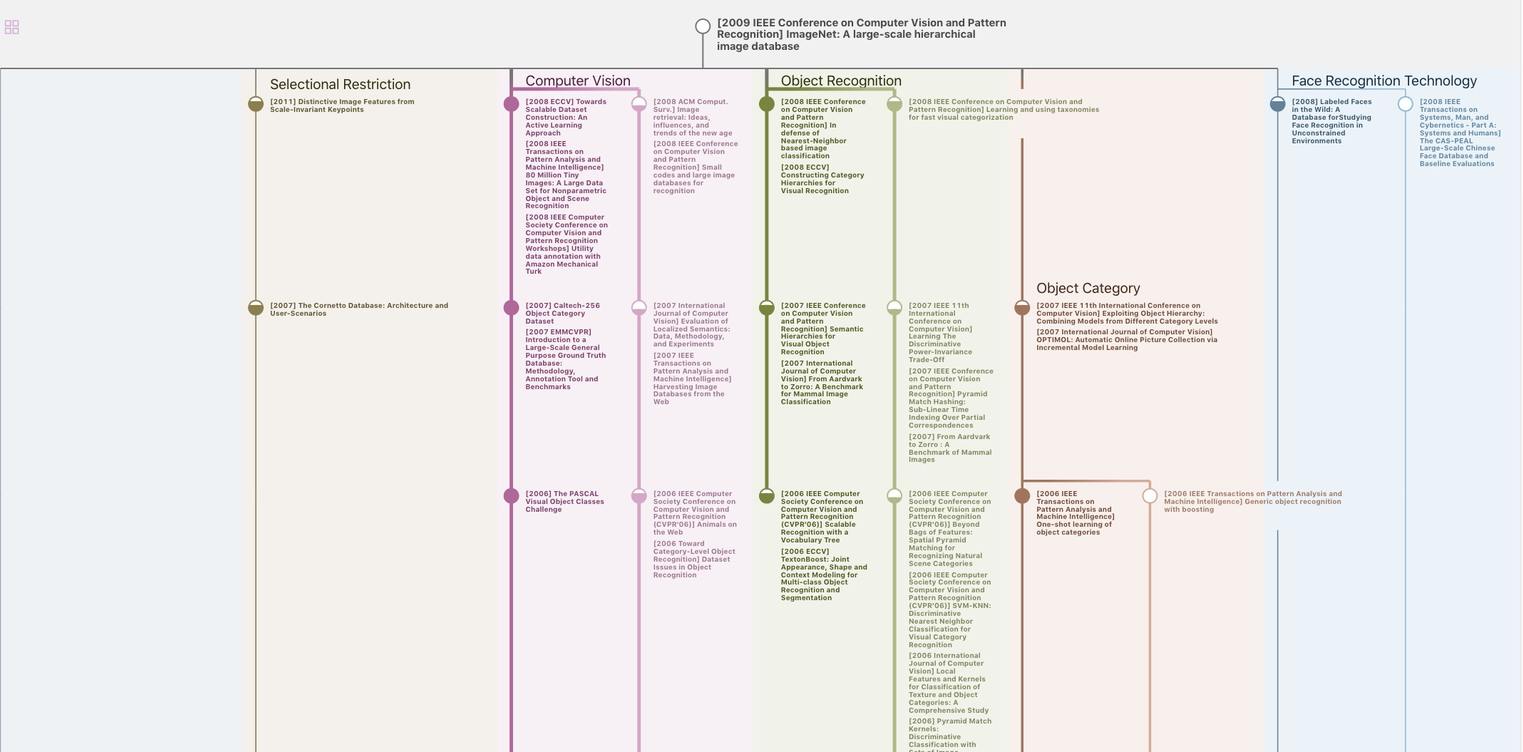
生成溯源树,研究论文发展脉络
Chat Paper
正在生成论文摘要