Identifying characteristic genes of ferroptosis in osteoarthritis based on machine learning and analysis of immune infiltration
Research Square (Research Square)(2023)
摘要
Abstract Objective: Machine learning was used to identify the characteristic genes associated with ferroptosis in osteoarthritis (OA) and immune infiltration analysis. Methods: Microarray datasets were downloaded from the GEO database, while genes associated with ferroptosis were retrieved from the FerrDb website. R language was used to batch correct data, extract OA ferroptosis genes, and analyze the differences between them. GO、KEGG、DO and PPI analysis was performed on DEGs, and machine learning techniques were utilized for the selection of characteristic genes associated with ferroptosis in OA. Further PCR assay validation and relevant immune infiltration analysis were carried out. Results: 24 DEGs related to ferroptosis in OA were obtained, which included 21 down-regulated and 3 up-regulated genes. It mainly involves the reaction to oxidative stress, apical cell , IL17 and TNF signaling pathways. Ferroptosis is also associated with female genital tumors and other diseases. WGCNA, SVM-RFE, and LASSO regression analyses were utilized to select 12, 9, and 4 genes. The characteristic gene KLF2 was obtained after the intersection. After verification, it was found that KLF2 was more accurate as a disease-characteristic gene; It was further confirmed that the expression of KLF2 was lower in the test group than in the control group using PCR assay. Immune infiltration analysis showed that activated mast cells were closely related to resting dendritic cells, and KLF2 was closely related to T cells follicular helper. Conclusion: Machine learning methods can be used to obtain KLF2, a gene characteristic of OA Ferroptosis, and effectively elucidate its pathogenesis.
更多查看译文
关键词
osteoarthritis,ferroptosis,machine learning,characteristic genes
AI 理解论文
溯源树
样例
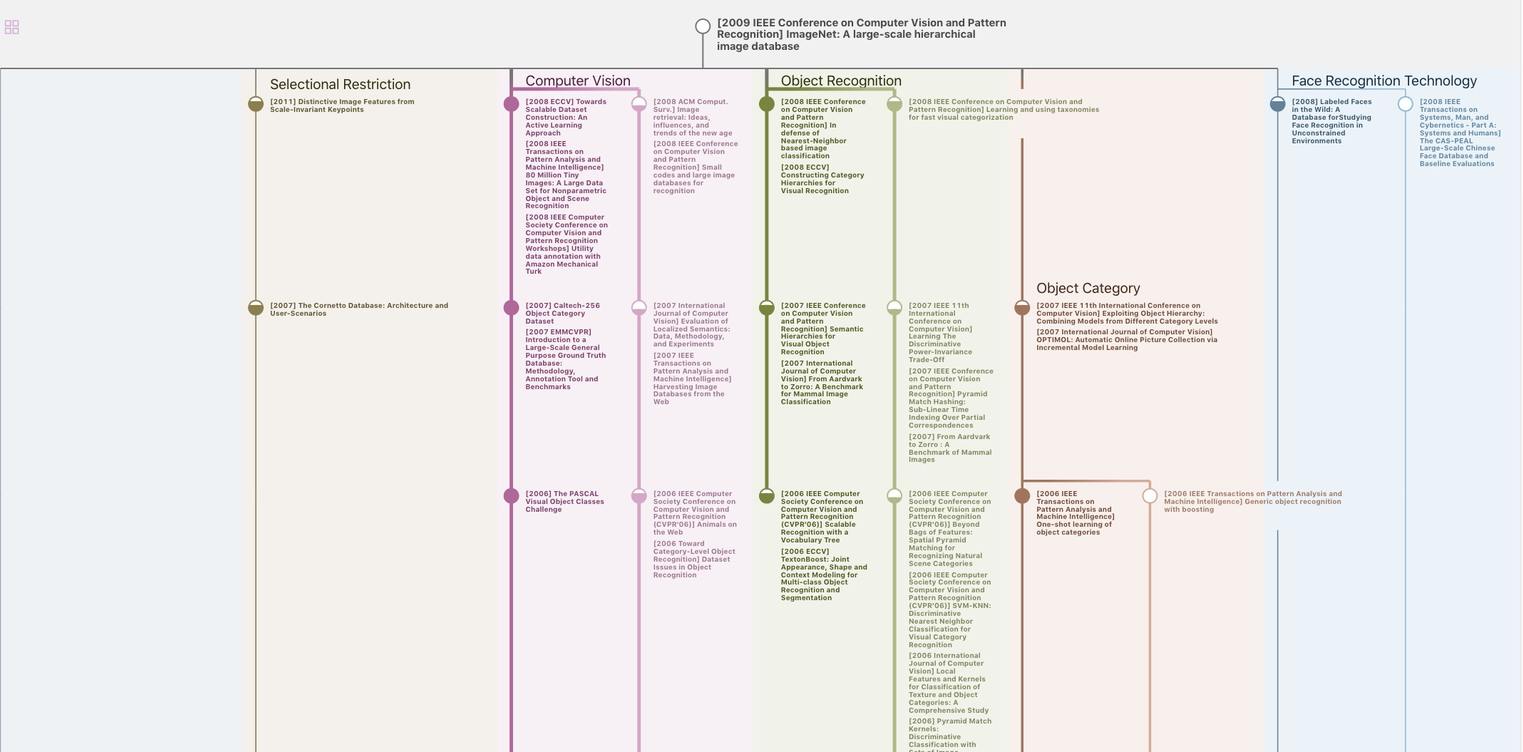
生成溯源树,研究论文发展脉络
Chat Paper
正在生成论文摘要