Industrial Data Modeling with Low-Dimensional Inputs and High-Dimensional Outputs.
IEEE transactions on industrial informatics(2024)
摘要
Data-driven modeling will be complicated for a process if the output quality indices are defined in a high-dimensional space, e.g., a quality distribution. In this article, a novel probabilistic modeling method is proposed for industrial processes with low-dimensional inputs and high-dimensional outputs. First, based on a limited sample set, the variational autoencoder (VAE) is applied to extract features of the high-dimensional outputs. Next, a Gaussian process (GP) model is established on the submanifold space defined by the low-dimensional features, and the high-dimensional predictions can be obtained through the VAE reverse procedure. Finally, a deep composing kernel strategy is developed to capture the nonlinearity and correlation hidden in the features. It can significantly improve the generalization performance of the GP model. The effectiveness of the proposed modeling algorithm is demonstrated by applications in a continuous crystallizer system and an ethylene homopolymerization system.
更多查看译文
关键词
Deep composing kernel,Gaussian process (GP),high-dimensional output,limited data,variational autoencoder (VAE)
AI 理解论文
溯源树
样例
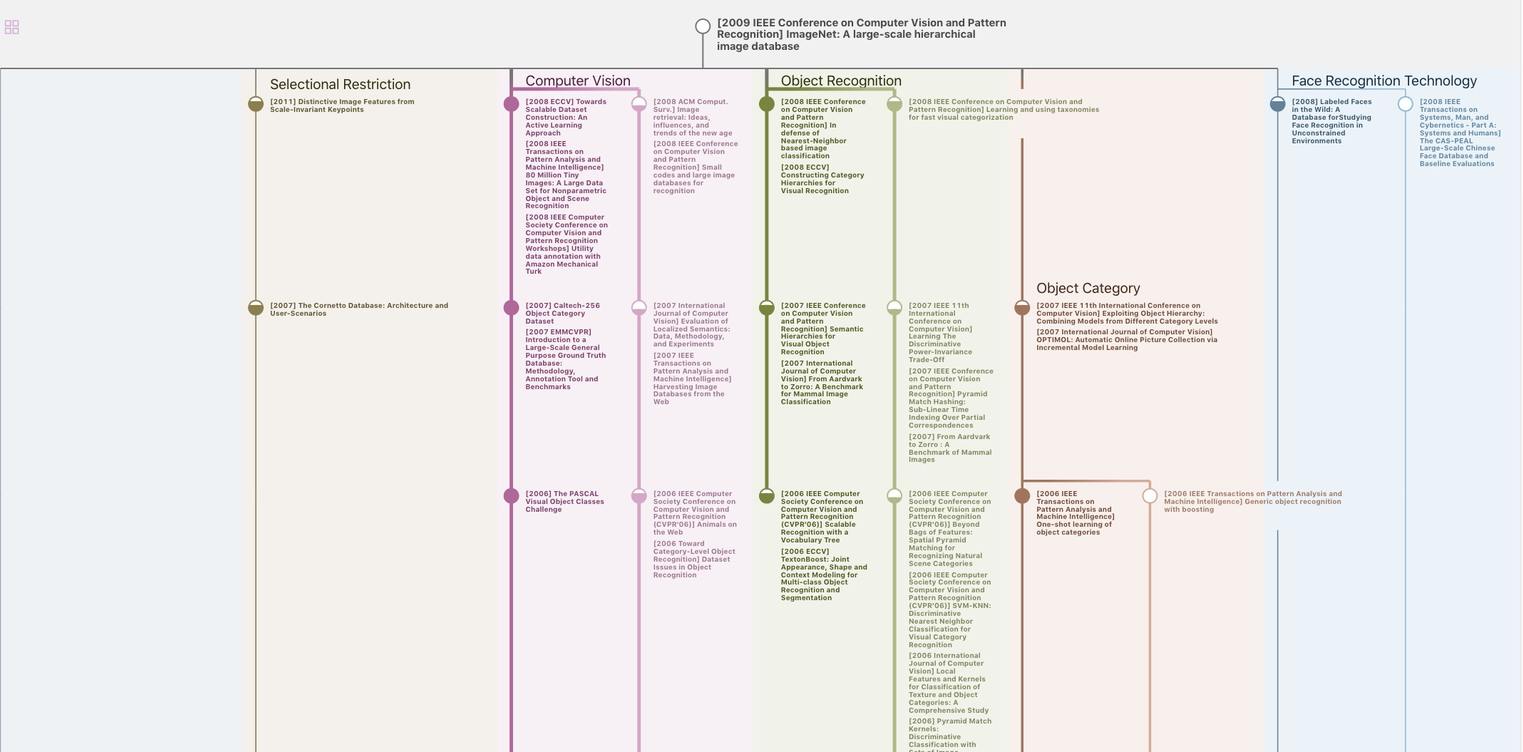
生成溯源树,研究论文发展脉络
Chat Paper
正在生成论文摘要