Abstract LB077: Analysis of indel and structural variant error profiles in deep next generation sequencing data
Cancer Research(2023)
摘要
Abstract Background: Accurate detection of low frequency mutations is of critical importance in the study of genetic heterogeneity, such as on the detection of minimal residual diseases for leukemias. Our prior work has resulted in successful error suppression for substitutions (10−4-10−5). However, the error profiles of indels and structural variants (SVs) remain elusive. Results: In this work, we generated ultra-deep sequencing data using our previously established dilution models (COLO829) on known somatic indels (n=23) and SVs (n=17). We discovered that the error rate of indels (10−6) and SVs (<2 × 10−7) are 100- to >1000-fold lower than that of SNVs. This finding was fully recapitulated in our analysis of 347 indels and 1248 SVs discovered from a relapsed B-ALL cohort of 103 patients, although homopolymer indels can have high error rates (>1%). We then performed a comprehensive study of homopolymer indels in 361 cancer driver genes by using whole genome data from 1662 healthy donors from the SJLIFE cohort. Our data indicated that the number of repeating units are highly predictive relative to the error rate of homopolymer indels (R2=0.988, p=4.89 × 10−8). Utilizing these insights, we assayed end-of-induction remission samples from 72 B-cell lymphoblastic leukemia patients that relapsed by selecting ~5 somatic clonal SNV/Indel/SV markers, which confirmed that SVs and indels have >10-fold lower error rates than SNVs. Our next generation sequencing (NGS) approach had 44 positive detections (61%) and outperformed the current standard method of clinical flow cytometry (n=37; 51%) for detecting minimal residual disease. The NGS-based method detected 92% of designed markers for samples with MRD>0.3%, and this detection rate dropped to 27% for MRD between 0.1% and 0.01%, indicating the difficulty in recovering mutant molecules when their frequencies are very low. Conclusions: Overall, we established indel and SV error profiles in deep next generation sequencing data enabling superior tumor detection performance at very low burdens, with lower error rates than what is observed for SNVs. Our work will have a significant impact on the clinical diagnosis and monitoring of human cancers and beyond. Citation Format: Ying Shao, Quang Tran, Pandurang Kolekar, Yanling Liu, Andrea McBride, Tyler Jones, Heather Mulder, Lingyun Ji, Benjamin Huang, Soheil Meshinchi, Jeffery Klco, Jinghui Zhang, William Carroll, Mignon Loh, Patrick Brown, John Easton, Xiaotu Ma. Analysis of indel and structural variant error profiles in deep next generation sequencing data [abstract]. In: Proceedings of the American Association for Cancer Research Annual Meeting 2023; Part 2 (Clinical Trials and Late-Breaking Research); 2023 Apr 14-19; Orlando, FL. Philadelphia (PA): AACR; Cancer Res 2023;83(8_Suppl):Abstract nr LB077.
更多查看译文
关键词
structural variant error profiles,sequencing,abstract lb077,deep next generation
AI 理解论文
溯源树
样例
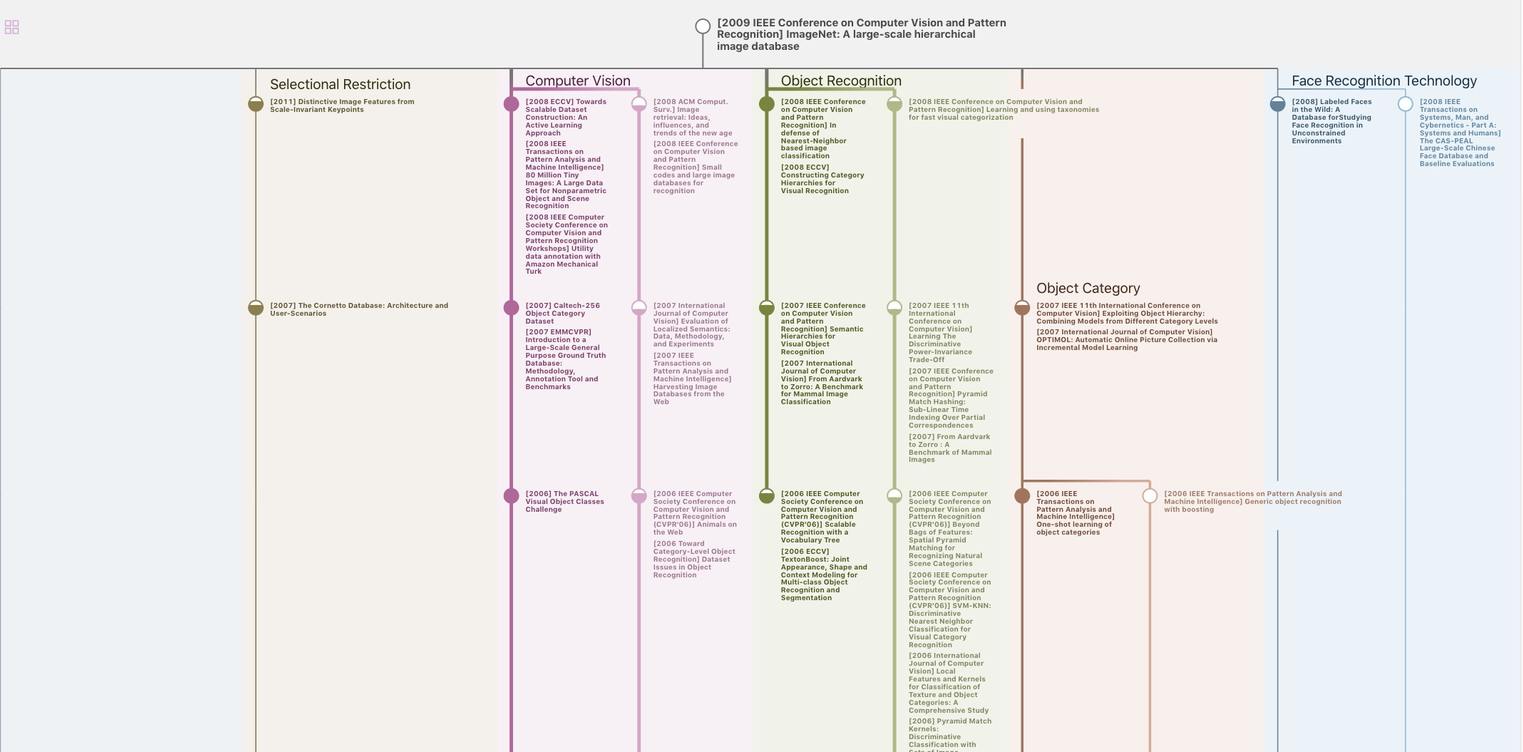
生成溯源树,研究论文发展脉络
Chat Paper
正在生成论文摘要