Using Meta-Learning to Reduce the Effort of Training New Workpiece Geometries for Entanglement Detection in Bin-Picking Applications
ARENA2036(2023)
摘要
Abstract In this paper, we introduce a scaling method for the training of neural networks used for entanglement detection in Bin-Picking. In the Bin-Picking process of complex-shaped and chaotically stored objects, entangled workpieces are a common source of problems. It has been shown that deep neural networks, which are trained using supervised learning, can be used to detect entangled workpieces. However, this strategy requires time-consuming data generation and an additional training process when adapting to previously unseen geometries. To solve this problem, we analyze and compare several Meta-Learning techniques like Reptile, MAML and TAMS for their feasibility as a scaling method for the entanglement detection. These methods search for a strongly generalized model for entanglement detection by learning from the training process of various workpieces with different geometries. Using this generalized model for entanglement detection as initialization helps to increase the learning success with only few training epochs and reduces the required amount of data and therefore the setup effort significantly.
更多查看译文
关键词
entanglement detection,training new workpiece geometries,meta-learning,bin-picking
AI 理解论文
溯源树
样例
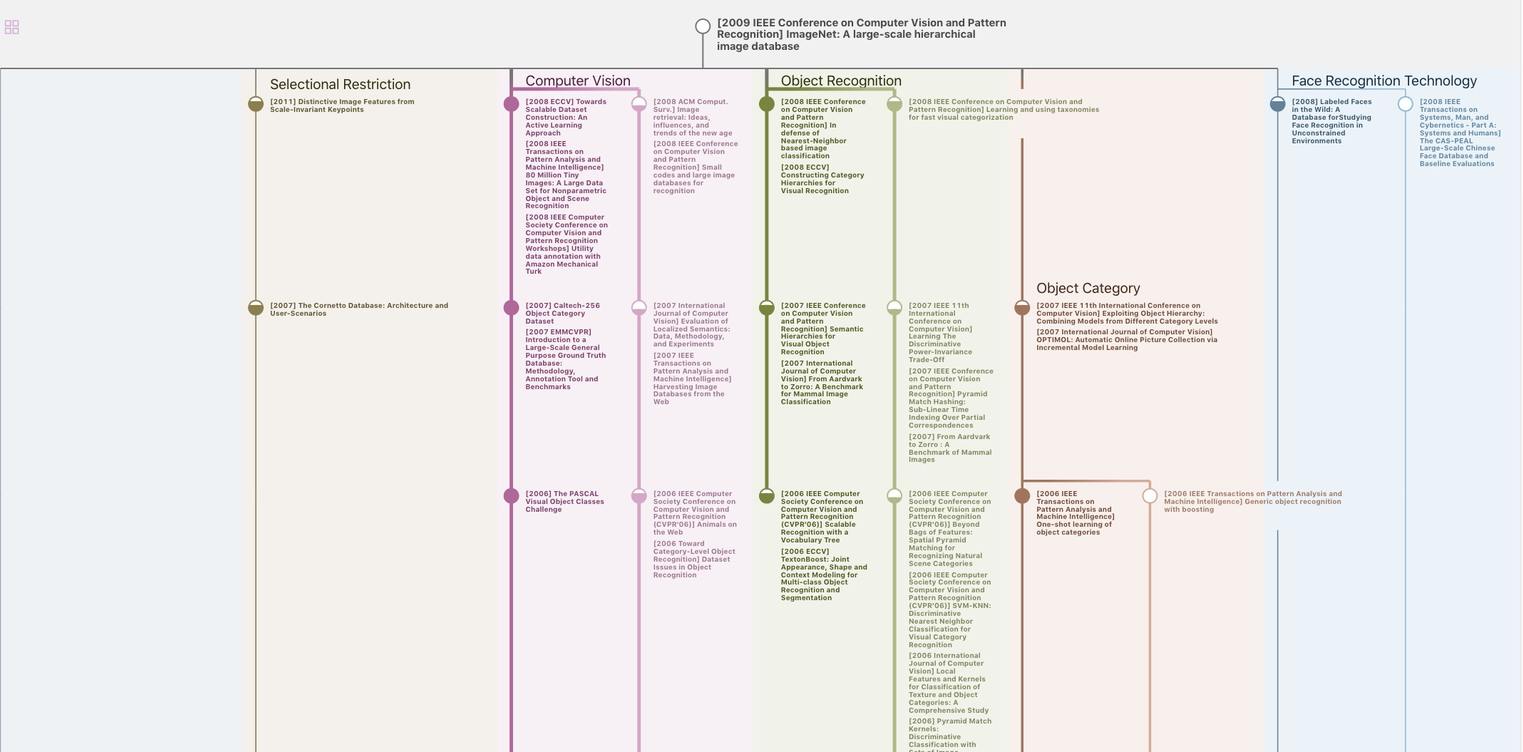
生成溯源树,研究论文发展脉络
Chat Paper
正在生成论文摘要