Pos1469 longitudinal characterization of disease complexity and flares in systemic lupus erythematosus: development of an electronic health records algorithm
Annals of the Rheumatic Diseases(2023)
摘要
Background Systemic Lupus Erythematous(SLE) is a heterogeneous disease, with a relapsing-remitting pattern that contributes to create difficulties for diagnosis and management. Besides, disease activity indices are not always able to capture the complexity of the disease over time and to guide therapy modification. Data mining and machine learning algorithms developed based on Electronic Health Records(EHR) could help in characterizing the disease complexity and electronic trajectory of evolution. Objectives to develop a machine-learning methodology to identify SLE phenotypes and flare trajectories in a large longitudinal database. Methods an observational retrospective monocenter study was performed using the EHR of our Tertiary Care University Hospital. Adult SLE patients (pts), with at least one hospital contact between January 2012 and December 2020, were included. For each patient, clinical reports including demographics characteristics, symptoms and clinical history retrieved from text notes recorded during the hospitalization and the out-patient visits, laboratory values, medication and laboratory prescriptions as well as therapy, were extracted from EHR (ICD9 710.0 and 695.4 to identify the pts). A predefined combination of Natural Language Processing techniques (NLP) and abnormal laboratory parameters at each visit was used to identify: 1)presence of 8 different SLE clinical phenotypes (hematological, muco-cutaneous, articular, renal, systemic, neurologic, vascular involvement and serositis); 2)disease complexity based on the combination of the presence of single or multiple organ domains involved and therapy escalation (defined as low, medium, high complexity); 3)disease flares in each of the above clinical phenotypes. Data extraction, from unstructured data of EHR, was performed using topic models and rule-based techniques to build a usable data mart. A p-value<0.05 was considered as significant. Typical explorative analyses were also used to identify SLE phenotypes, together with longitudinal analyses, for the observation of evolution of complexity trajectories. Results A total of 1000 SLE pts with at least one contact were identified in our EHR; of them 477 presented at least one hospitalization and 255 pts presented at least one year of follow-up with a median of years of observation of 5.0(3.0, 8.0). In the longitudinal cohort of 255 pts, the median number of outpatient clinic contacts was 14(8-20) and of hospitalizations: 1 [0-2]. The median number of clinical phenotypes identified at baseline was 4(2.25-5), with 50.3% of pts having more than 3; and their distribution was: hematological(71.4%), muco-cutaneous(68.2%), articular(64.3%), renal (62.4%), vascular(34.9%), serositis(24.3%), systemic(16.5%), neurological(16.1%). At baseline, SLE complexity was categorized as low, medium and high in 13.7%, 34.5% and 51.8% of cases respectively. During follow up the complexity of pts changed as reported in Figure 1 , and 93% of pts had a flare. During longitudinal evaluation, 65.9% pts presented hematologic flare, 60% muco-cutaneous, 47.8% renal, 18.8% articular, 12.9% vascular, 7.8% systemic, 5.1% serositis and 3.9% neurological. The median number of flares significantly increased with disease complexity [(3.5(2.0-6.0), 4.0(2.0-8.0), 6(3.0-9.2) in pts with low, medium and high complexity respectively,p<0.05]. Moreover, the severity of flares for each clinical phenotypes also increased according to the patient’s complexity(p<0.05). Overall, 279 therapy escalations were made over the follow up time, 62% due to disease flares, and 38% due to other situations (e.g. intolerance, contraindications). Conclusion Machine learning algorithms can help to describe SLE heterogeneity and to characterize clinical phenotypes and trajectories of pts. Next step will be the evaluation of the present procedure on external cohorts to develop a tool able to identify situations needing more intensive and personalized care. Figure 1. Acknowledgements The project has been developed with the financial contribution of Astra Zeneca. Disclosure of Interests None Declared.
更多查看译文
关键词
disease complexity,systemic lupus erythematosus,lupus erythematosus,systemic lupus,electronic health records
AI 理解论文
溯源树
样例
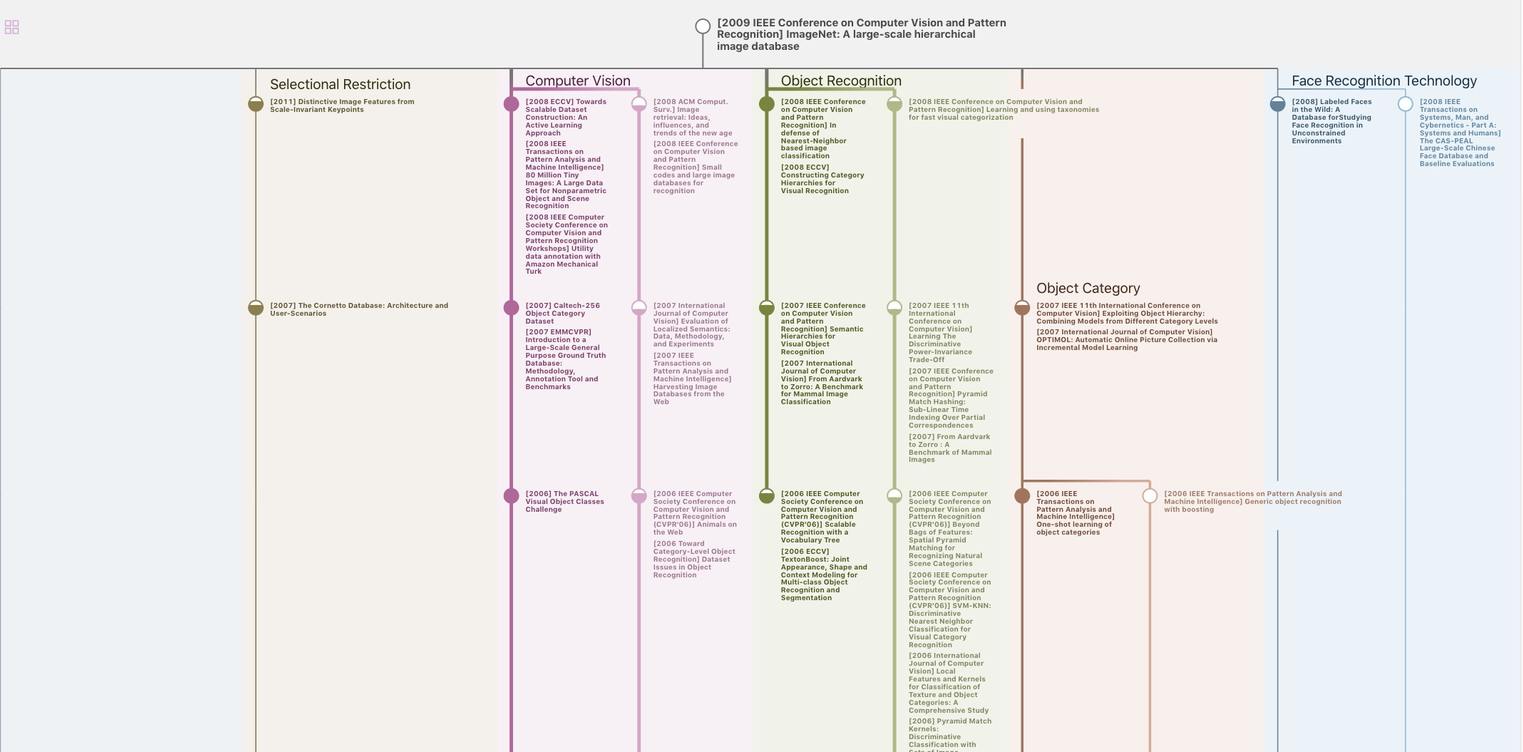
生成溯源树,研究论文发展脉络
Chat Paper
正在生成论文摘要