Carbon Neutrality Computational Cost Optimization for Economic Dispatch With Carbon Capture Power Plants in Smart Grid
IEEE transactions on sustainable computing(2023)
摘要
To achieve carbon neutrality, reducing carbon emissions is crucial in dispatching problems in smart grid. Though renewable energy such as wind power has low carbon emissions, it suffers from random generation, which makes the thermal power necessary for a stable supply power system. To reduce carbon emissions, the thermal power plants are transformed into carbon capture power plants, which brings new challenges to economic dispatch algorithms. Besides, there are usually many constraints to keep the security operation of power systems, which incurs a large problem scale and high computational cost. Most existing methods either do not consider reducing carbon emissions, or suffer from high computational costs. In this paper, a framework for the carbon capture plants with wind power to reduce both running costs and carbon emissions is designed to support carbon neutrality. To reduce computational cost, initial-training and fine-tuning are used. A deep neural network is employed to describe the relationship between users' load and the constraints, which provides guides for finding the active constraints. Therefore, the problem scale can be significantly decreased, making the optimal dispatching strategy obtained quickly. The experimental results on real-world data show that the proposed framework can obtain the optimal strategy efficiently.
更多查看译文
关键词
Carbon capture power plants,carbon neutrality,deep learning,security constrained economic dispatch
AI 理解论文
溯源树
样例
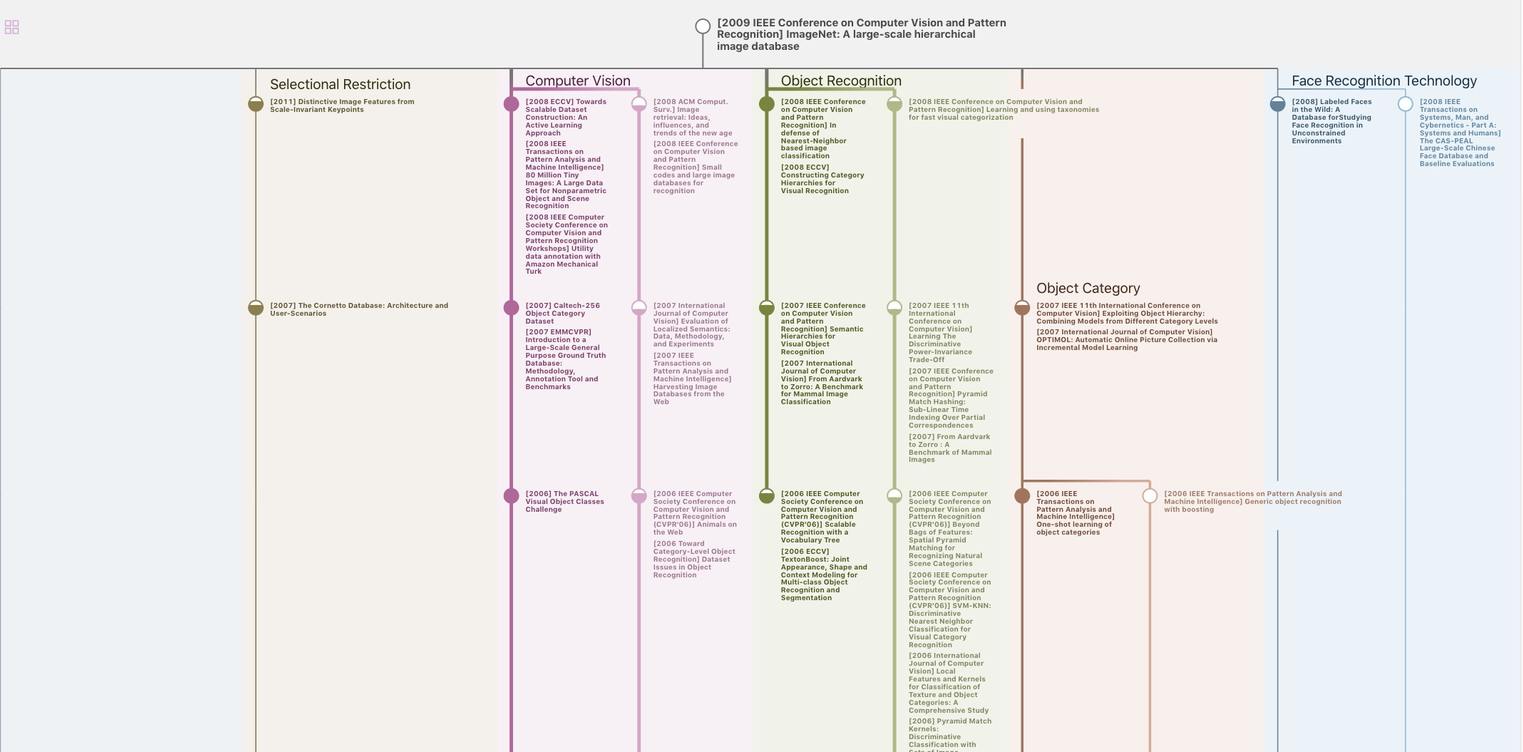
生成溯源树,研究论文发展脉络
Chat Paper
正在生成论文摘要