Adversarial Search and Tracking with Multiagent Reinforcement Learning in Sparsely Observable Environment
2023 INTERNATIONAL SYMPOSIUM ON MULTI-ROBOT AND MULTI-AGENT SYSTEMS, MRS(2023)
摘要
We study a search and tracking (S&T) problem where a team of dynamic search agents must collaborate to track an adversarial, evasive agent. The heterogeneous search team may only have access to a limited number of past adversary trajectories within a large search space. This problem is challenging for both model-based searching and reinforcement learning (RL) methods since the adversary exhibits reactionary and deceptive evasive behaviors in a large space leading to sparse detections for the search agents. To address this challenge, we propose a novel Multi-Agent RL (MARL) framework that leverages the estimated adversary location from our learnable filtering model. We show that our MARL architecture can outperform all baselines and achieves a 46% increase in detection rate.
更多查看译文
关键词
Multi-agent Reinforcement Learning,Adversarial Search,Search For Agents,Filter Model,Agent Dynamics,Increase In Detection Rate,Neural Network,State Space,Mixture Model,Current Position,Sampling Efficiency,Motion Model,Heuristic Search,Drug Trafficking,Markov Decision Process,Learned Weights,Closest Distance,Current Time Step,Filter Module,Prior Network,Classical Filter
AI 理解论文
溯源树
样例
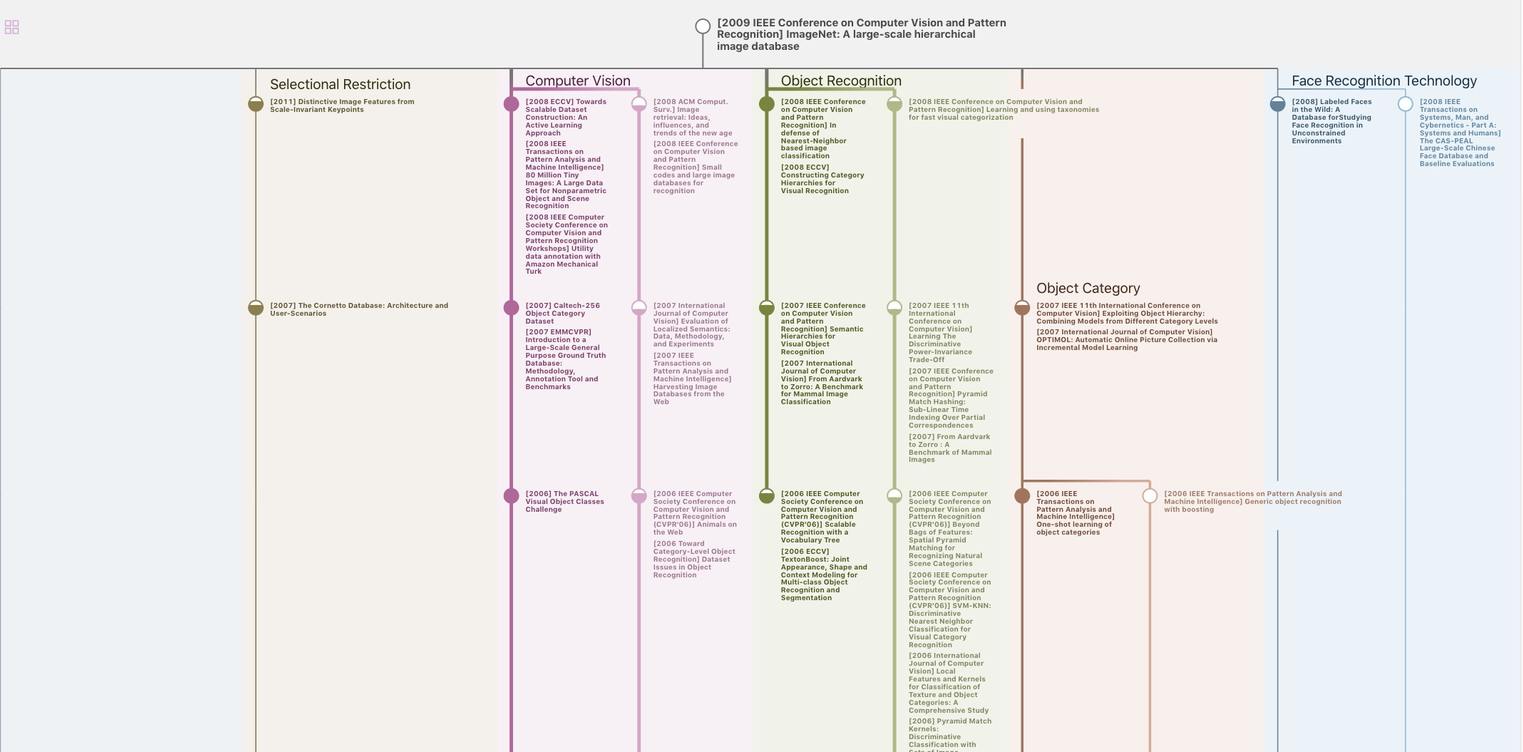
生成溯源树,研究论文发展脉络
Chat Paper
正在生成论文摘要