Adaptive Training and Aggregation for Federated Learning in Multi-Tier Computing Networks.
IEEE Trans. Mob. Comput.(2024)
摘要
Multi-tier computing (MC) utilizes computing resources from the cloud, fog, edge, and end layers to promote intelligent Internet of Things (IoT) applications. Federated learning (FL) in MC offers a prospective distributed and privacy-preserving framework to deploy deep learning applications in different layers. Due to time-varying network topologies, wireless channel states, and computational workloads, MC faces a dynamic and uncertain environment, which poses additional challenges to FL task processing. In this paper, we propose a novel adaptive training and aggregation federated learning (ATAFL) framework. Specifically, local model training can be performed at end devices, edge nodes, and fog nodes. The global aggregator can choose from the edge, fog, and cloud layers. A joint optimization problem of training, aggregation node selection, and resource allocation is further formulated to minimize system latency and energy consumption. Moreover, digital twin and deep reinforcement learning (DRL) techniques are integrated into the MC network to design optimal node selection and resource allocation strategies based on captured state information of the MC system. We implement a prototype, and experimental results show that our proposed DRL-based algorithm reduces system latency and energy consumption compared with the benchmark algorithms.
更多查看译文
关键词
Multi-tier computing,Resource allocation,Task scheduling,Deep reinforcement learning,Federated learning
AI 理解论文
溯源树
样例
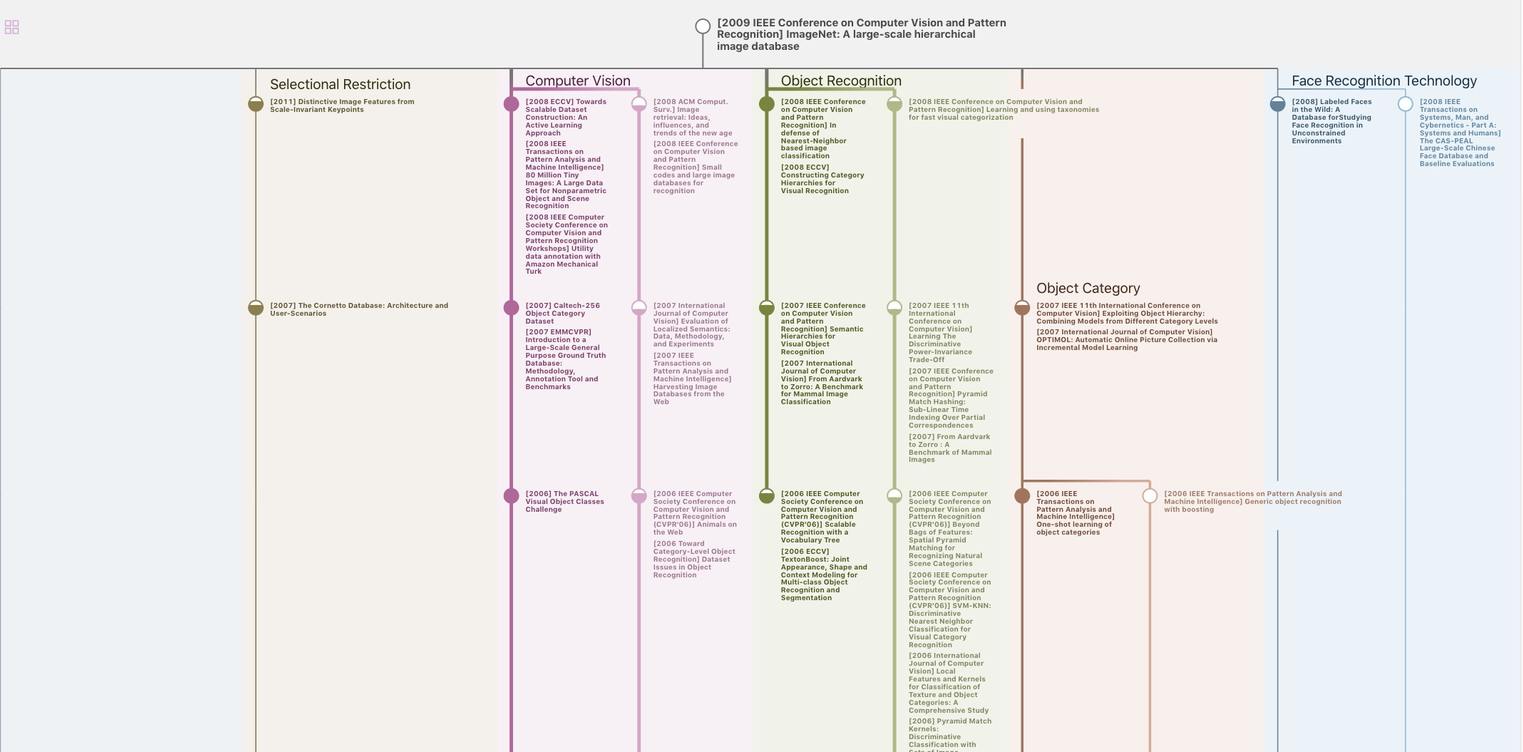
生成溯源树,研究论文发展脉络
Chat Paper
正在生成论文摘要