Reinforcement Learning with Decoupled State Representation for Robot Manipulations
Research Square (Research Square)(2023)
摘要
Deep reinforcement learning (DRL) has advanced robot manipulations with an alternative solution to design a control strategy using the raw image as the input directly. Although the image usually comes up with more knowledge about the environment, it needs the policy to achieve representation learning and task learning simultaneously, which is a sample inefficient task. Previous attempts, such as Variational Autoencoder (VAE) based DRL algorithms have attempted to solve this problem by learning a visual representation model, which encodes the entire image into a low-dimension vector. However, since the vector contains both the robot and object information, the coupling within the state is inevitable, which could mislead the training process of DRL policy. In this study, a novel method named Reinforcement Learning with Decoupled State Representation (RLDS) is proposed to decouple the robot and object information to increase the learning efficiency and effectiveness. The experimental results have shown that the proposed method has a faster learning speed and can achieve better performance compared with previous methods in several typical robot tasks. Additionally, with only 3,096 offline images, the proposed method can be successfully applied to a real robot pushing task, which demonstrates its high practicability.
更多查看译文
关键词
reinforcement learning,robot manipulations,decoupled state representation
AI 理解论文
溯源树
样例
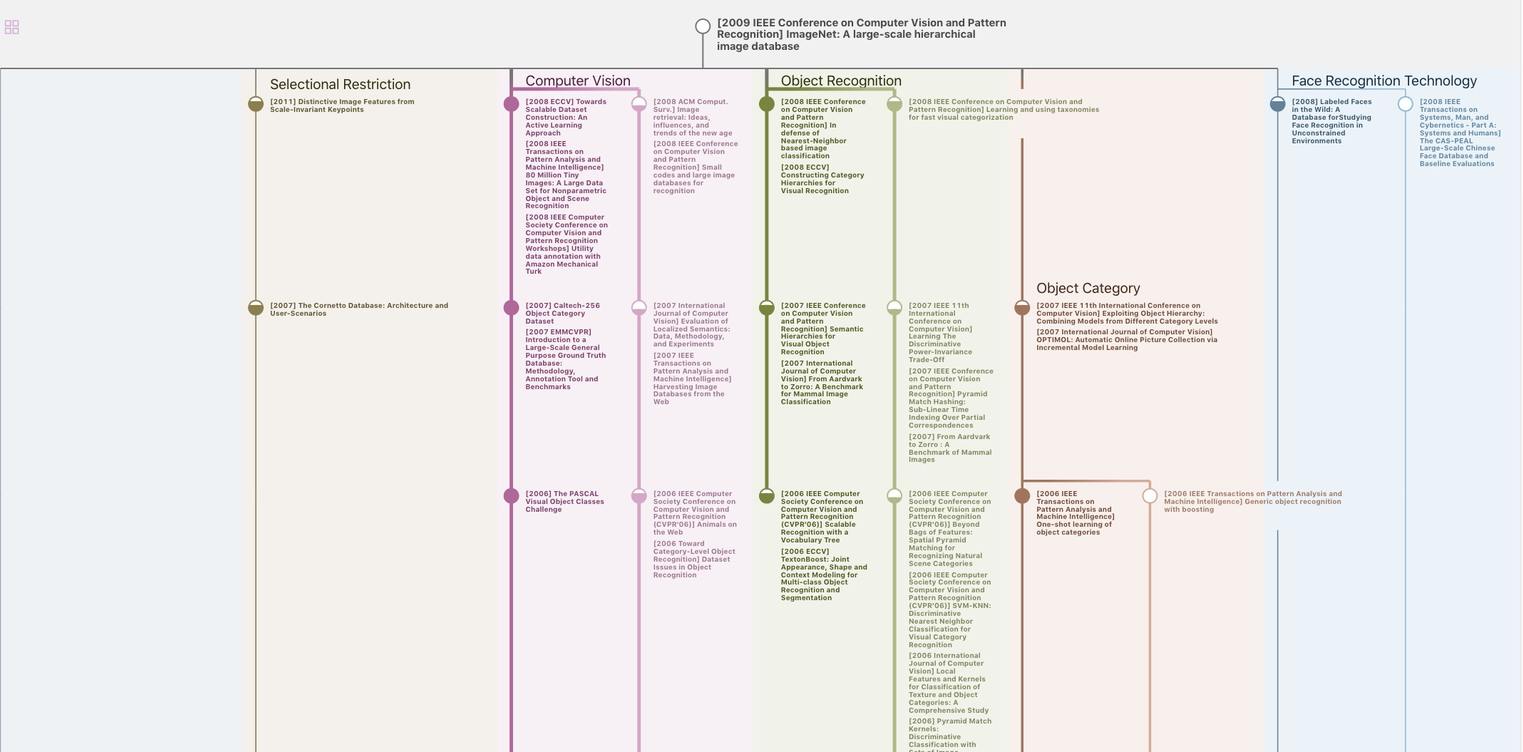
生成溯源树,研究论文发展脉络
Chat Paper
正在生成论文摘要