Selecting relevant moderators with Bayesian regularized meta‐regression
Research Synthesis Methods(2023)
摘要
Abstract When meta‐analyzing heterogeneous bodies of literature, meta‐regression can be used to account for potentially relevant between‐studies differences. A key challenge is that the number of candidate moderators is often high relative to the number of studies. This introduces risks of overfitting, spurious results, and model non‐convergence. To overcome these challenges, we introduce Bayesian Regularized Meta‐Analysis (BRMA), which selects relevant moderators from a larger set of candidates by shrinking small regression coefficients towards zero with regularizing (LASSO or horseshoe) priors. This method is suitable when there are many potential moderators, but it is not known beforehand which of them are relevant. A simulation study compared BRMA against state‐of‐the‐art random effects meta‐regression using restricted maximum likelihood (RMA). Results indicated that BRMA outperformed RMA on three metrics: BRMA had superior predictive performance, which means that the results generalized better; BRMA was better at rejecting irrelevant moderators, and worse at detecting true effects of relevant moderators, while the overall proportion of Type I and Type II errors was equivalent to RMA. BRMA regression coefficients were slightly biased towards zero (by design), but its residual heterogeneity estimates were less biased than those of RMA. BRMA performed well with as few as 20 studies, suggesting its suitability as a small sample solution. We present free open source software implementations in the R‐package pema (for penalized meta‐analysis) and in the stand‐alone statistical program JASP. An applied example demonstrates the use of the R‐package.
更多查看译文
关键词
relevant moderators,meta‐regression,bayesian
AI 理解论文
溯源树
样例
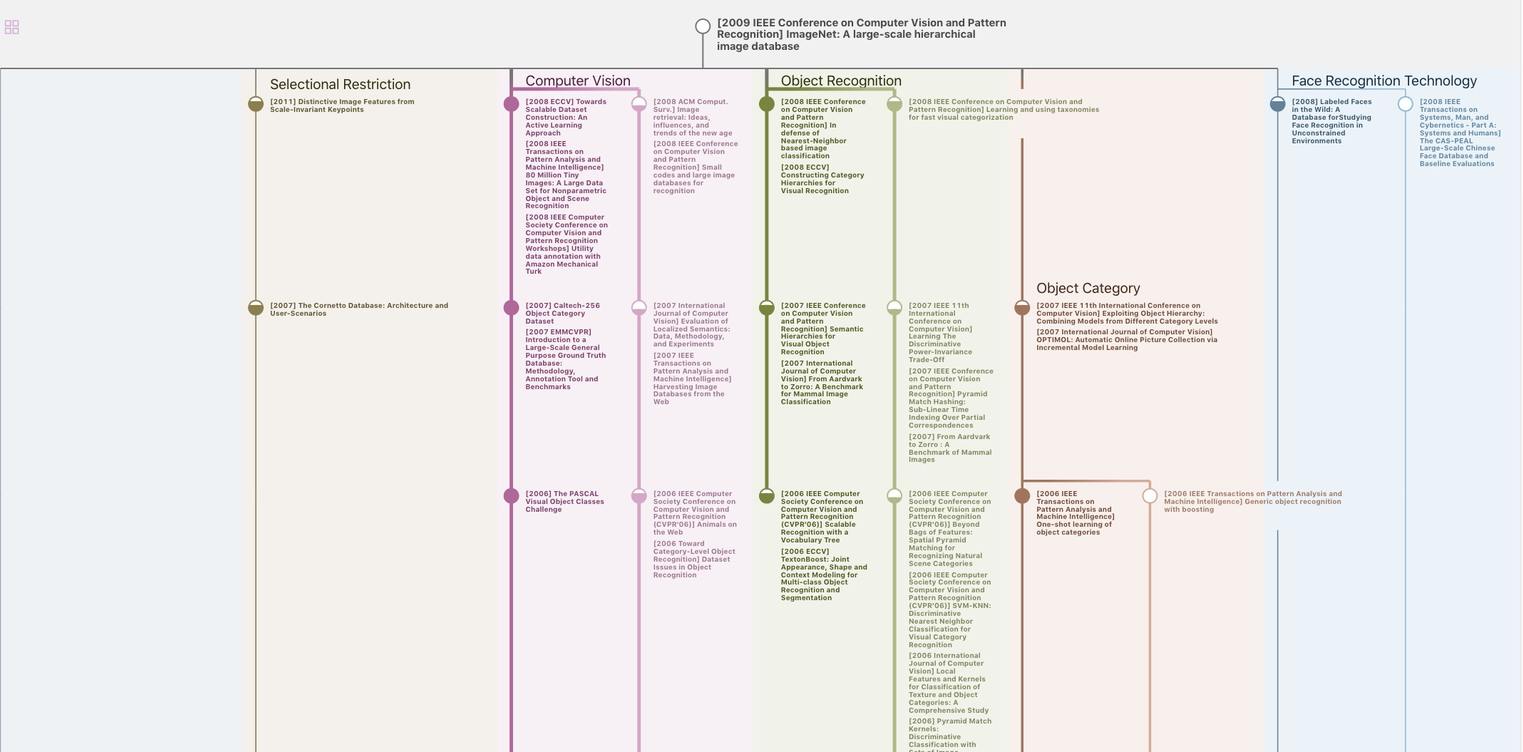
生成溯源树,研究论文发展脉络
Chat Paper
正在生成论文摘要