Highest Posterior Model Computation and Variable Selection via Simulated Annealing
The New England Journal of Statistics in Data Science(2023)
摘要
Variable selection is widely used in all application areas of data analytics, ranging from optimal selection of genes in large scale micro-array studies, to optimal selection of biomarkers for targeted therapy in cancer genomics to selection of optimal predictors in business analytics. A formal way to perform this selection under the Bayesian approach is to select the model with highest posterior probability. The problem may be thought as an optimization problem over the model space where the objective function is the posterior probability of model. We propose to carry out this optimization using simulated annealing and we illustrate its feasibility in high dimensional problems. By means of various simulation studies, this new approach has been shown to be efficient. Theoretical justifications are provided and applications to high dimensional datasets are discussed. The proposed method is implemented in an R package sahpm for general use and is made available on R CRAN.
更多查看译文
关键词
variable selection,model
AI 理解论文
溯源树
样例
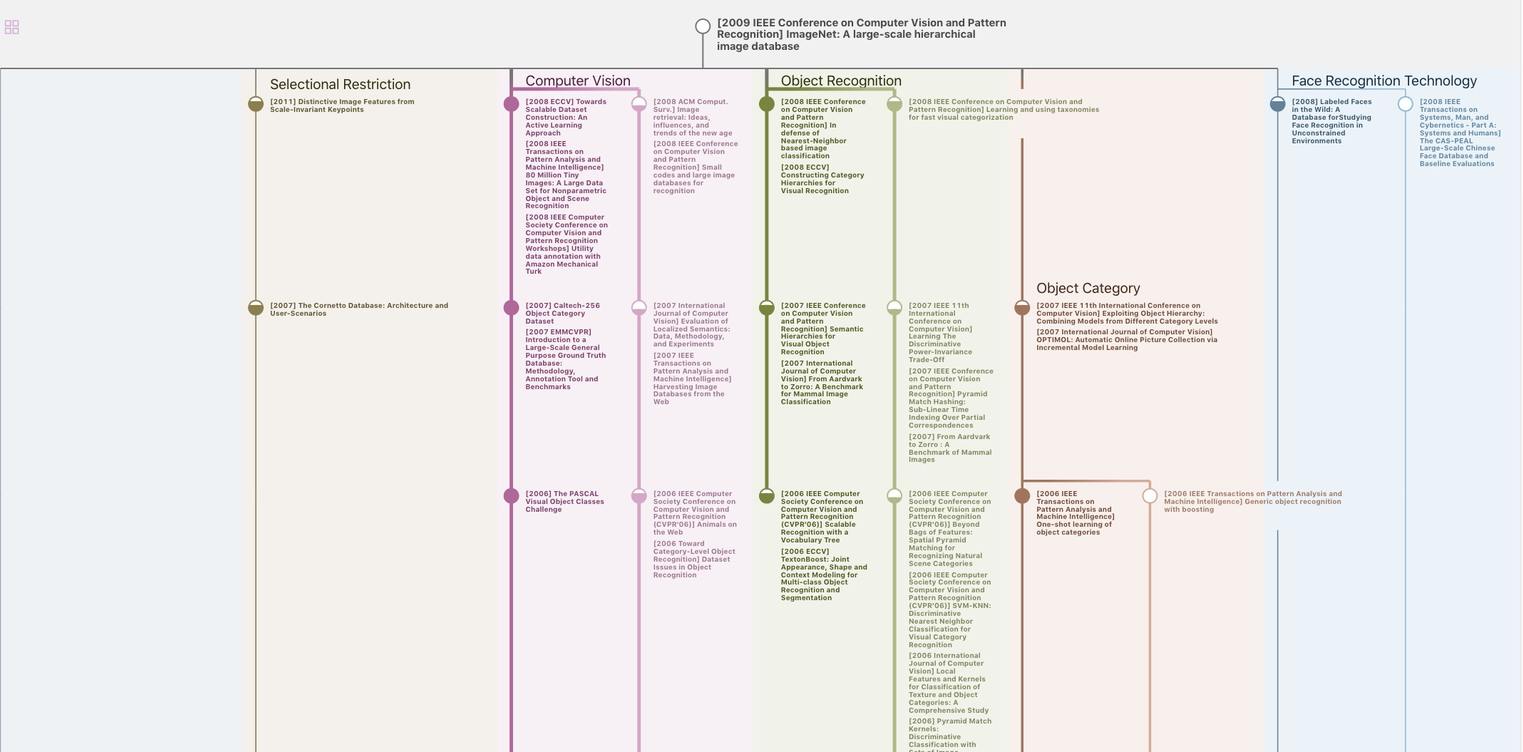
生成溯源树,研究论文发展脉络
Chat Paper
正在生成论文摘要