Frequency-aware optical coherence tomographyimage super-resolution via conditionalgenerative adversarial neural network
Biomedical Optics Express(2023)
摘要
Optical coherence tomography (OCT) has stimulated a wide range of medical image-based diagnosis and treatment in fields such as cardiology and ophthalmology. Such applications can be further facilitated by deep learning-based super-resolution technology, which improves the capability of resolving morphological structures. However, existing deep learning-based method only focuses on spatial distribution and disregard frequency fidelity in image reconstruction, leading to a frequency bias. To overcome this limitation, we propose a frequency-aware super-resolution framework that integrates three critical frequency-based modules (i.e., frequency transformation, frequency skip connection, and frequency alignment) and frequency-based loss function into a conditional generative adversarial network (cGAN). We conducted a large-scale quantitative study from an existing coronary OCT dataset to demonstrate the superiority of our proposed framework over existing deep learning frameworks. In addition, we confirmed the generalizability of our framework by applying it to fish corneal images and rat retinal images, demonstrating its capability to super-resolve morphological details in eye imaging.
更多查看译文
关键词
conditionalgenerative adversarial neural network,coherence,frequency-aware,super-resolution
AI 理解论文
溯源树
样例
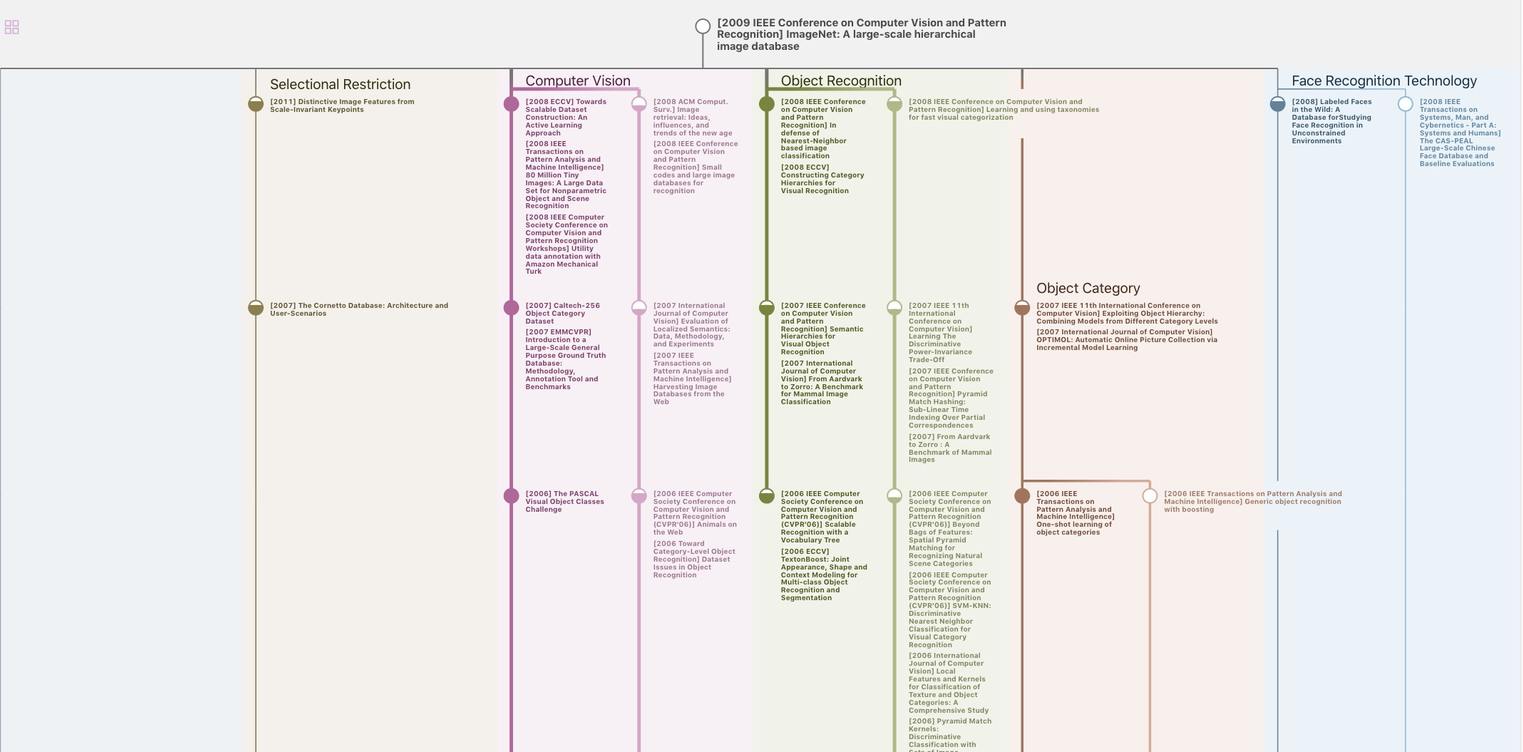
生成溯源树,研究论文发展脉络
Chat Paper
正在生成论文摘要