CAMF: An Interpretable Infrared and Visible Image Fusion Network Based on Class Activation Mapping
IEEE TRANSACTIONS ON MULTIMEDIA(2024)
摘要
Image fusion aims to integrate the complementary information of source images and synthesize a single fused image. Existing image fusion algorithms apply hand-crafted fusion rules to merge deep features which cause information loss and limit the fusion performance of methods since the uninterpretability of deep learning. To overcome the above shortcomings, we propose a learnable fusion rule for infrared and visible image fusion based on class activation mapping. Our proposed fusion rule can selectively preserve meaningful information and reduce distortion. More specifically, we first train an encoder-decoder network and an auxiliary classifier based on the shared encoder. Then, the class activation weights are taken out from the auxiliary classifier, which indicates the importance of each channel. Finally, the deep features extracted by the encoder are adaptively fused according to the class activation weights and the fused image is reconstructed from the fused features via the pre-trained decoder. Note that our learnable fusion rule can automatically measure the importance of each deep feature without human participation. Moreover, it fully preserves the significant features of source images such as salient targets and texture details. Extensive experiments manifest our superiority over state-of-the-art algorithms. Visualization of feature maps and their corresponding weights reveals the high interpretability of our method.
更多查看译文
关键词
Image fusion,Feature extraction,Transforms,Image reconstruction,Deep learning,Task analysis,Pollution measurement,learnable fusion rule,class activation mapping,deep learning
AI 理解论文
溯源树
样例
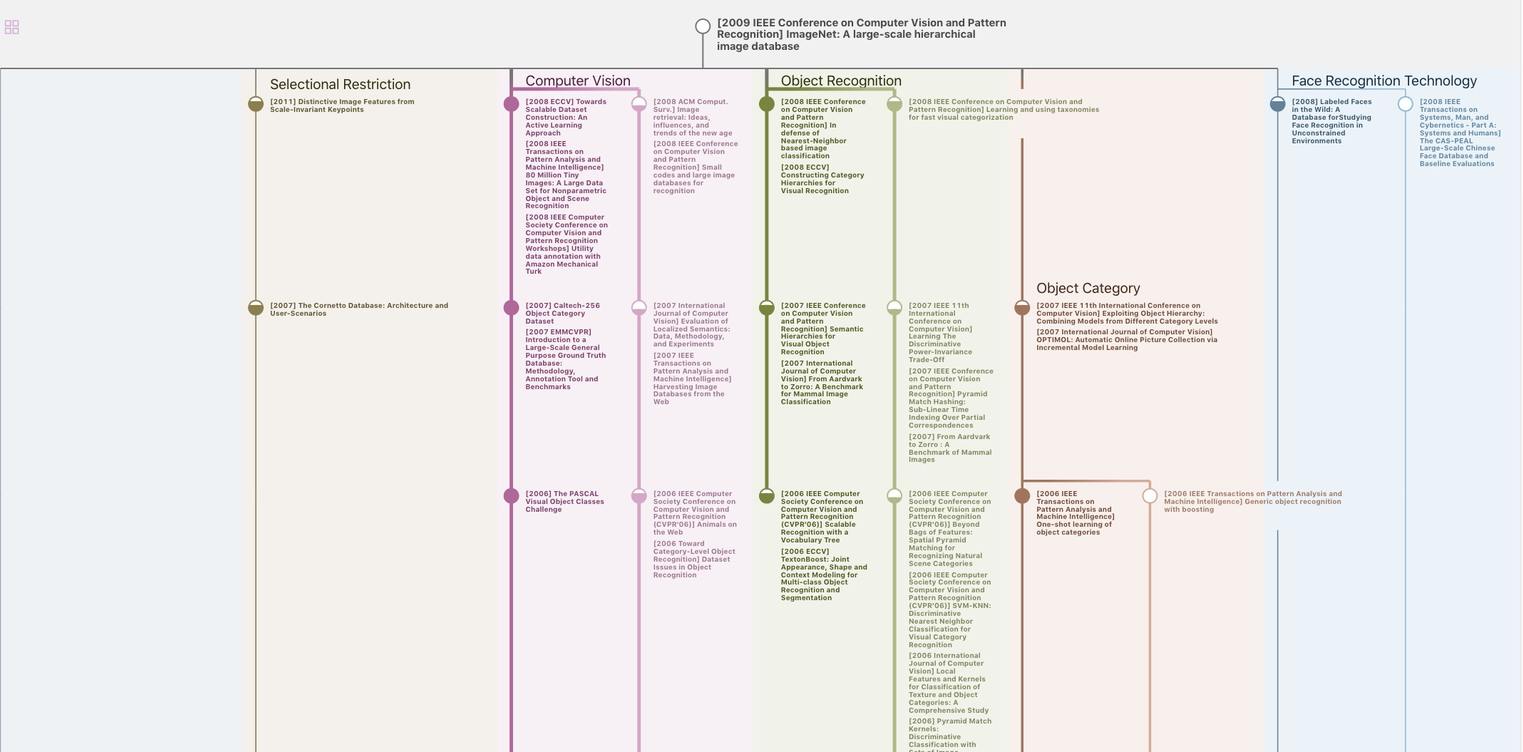
生成溯源树,研究论文发展脉络
Chat Paper
正在生成论文摘要