Online Training Flow Scheduling for Geo-Distributed Machine Learning Jobs over Heterogeneous and Dynamic Networks
IEEE TRANSACTIONS ON COGNITIVE COMMUNICATIONS AND NETWORKING(2024)
Key words
Training,Wide area networks,Bandwidth,Resource management,Machine learning,Synchronization,Data models,Geo-distributed machine leaning,training jobs,resource allocation,online scheduling
AI Read Science
Must-Reading Tree
Example
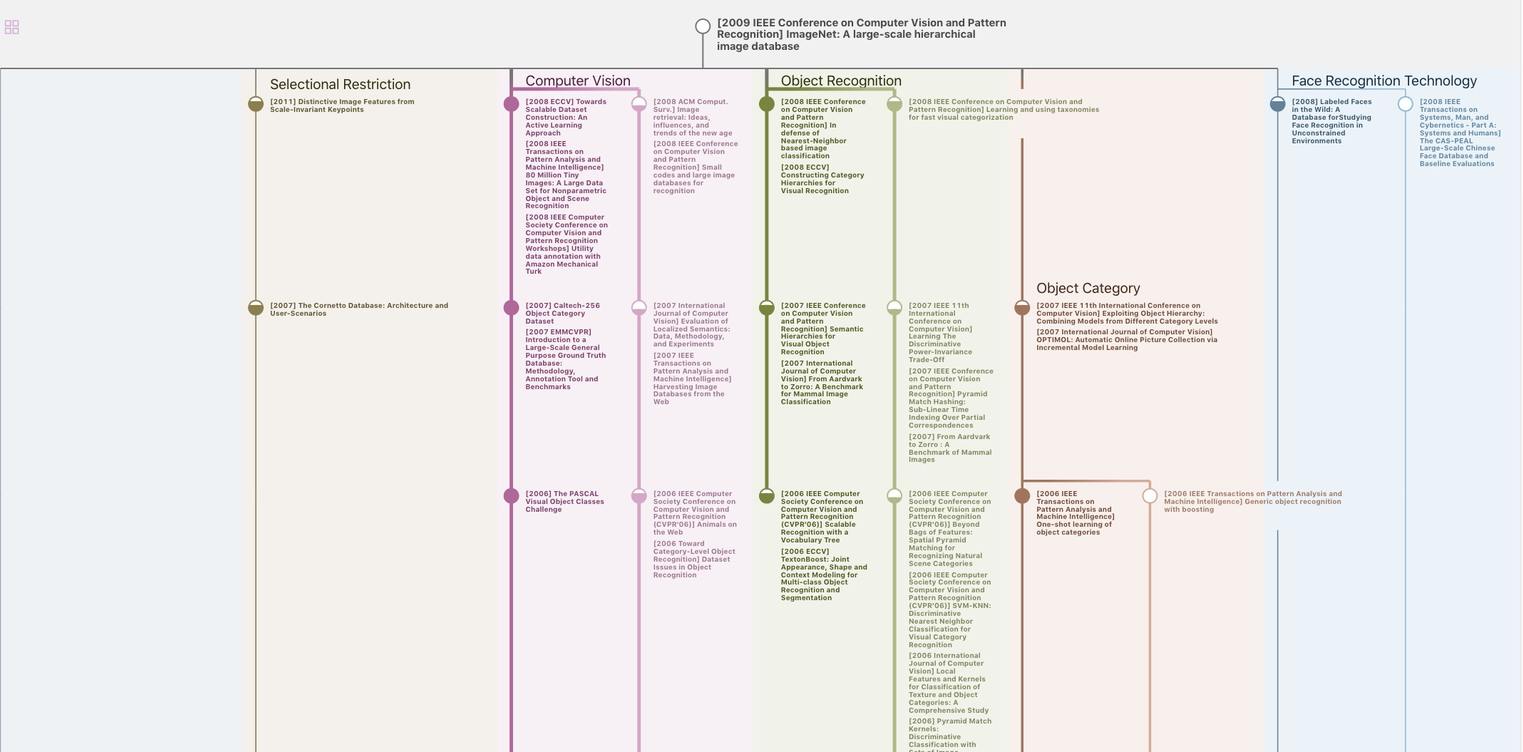
Generate MRT to find the research sequence of this paper
Chat Paper
Summary is being generated by the instructions you defined