Comparative Analysis of Parameter Convergence for Several Least-Squares Estimation Schemes.
IEEE TRANSACTIONS ON AUTOMATIC CONTROL(2024)
Abstract
Least-squares parameter estimation is important in system identification and adaptive control due to its enhanced performance and robustness compared to gradient-descent parameter estimation. Based on a unified class of uncertain nonlinear systems, we introduce the standard least-squares estimator (LSE) and six variations from the old to the latest ones, namely a filterless LSE, a high-gain LSE, a filtered high-gain LSE, a finite-time LSE, a dynamic regressor extension and mixing (DREM)-based LSE, and a composite learning LSE. A unique and in-depth comparative analysis of these LSEs is provided to reveal their technical natures and clarify several theoretical misunderstandings. Besides, it is demonstrated that only the finite-time LSE, DREM-LSE, and composite learning LSE can achieve parameter convergence under sufficient excitation that is strictly weaker than persistent excitation, and they can also be easily extended to the case with nonlinear-in-the-parameters uncertainties. Comprehensive simulation comparisons have verified the above theoretical findings.
MoreTranslated text
Key words
Adaptive control,closed-loop identification,least-squares estimation,nonlinear parametrization,parameter convergence
AI Read Science
Must-Reading Tree
Example
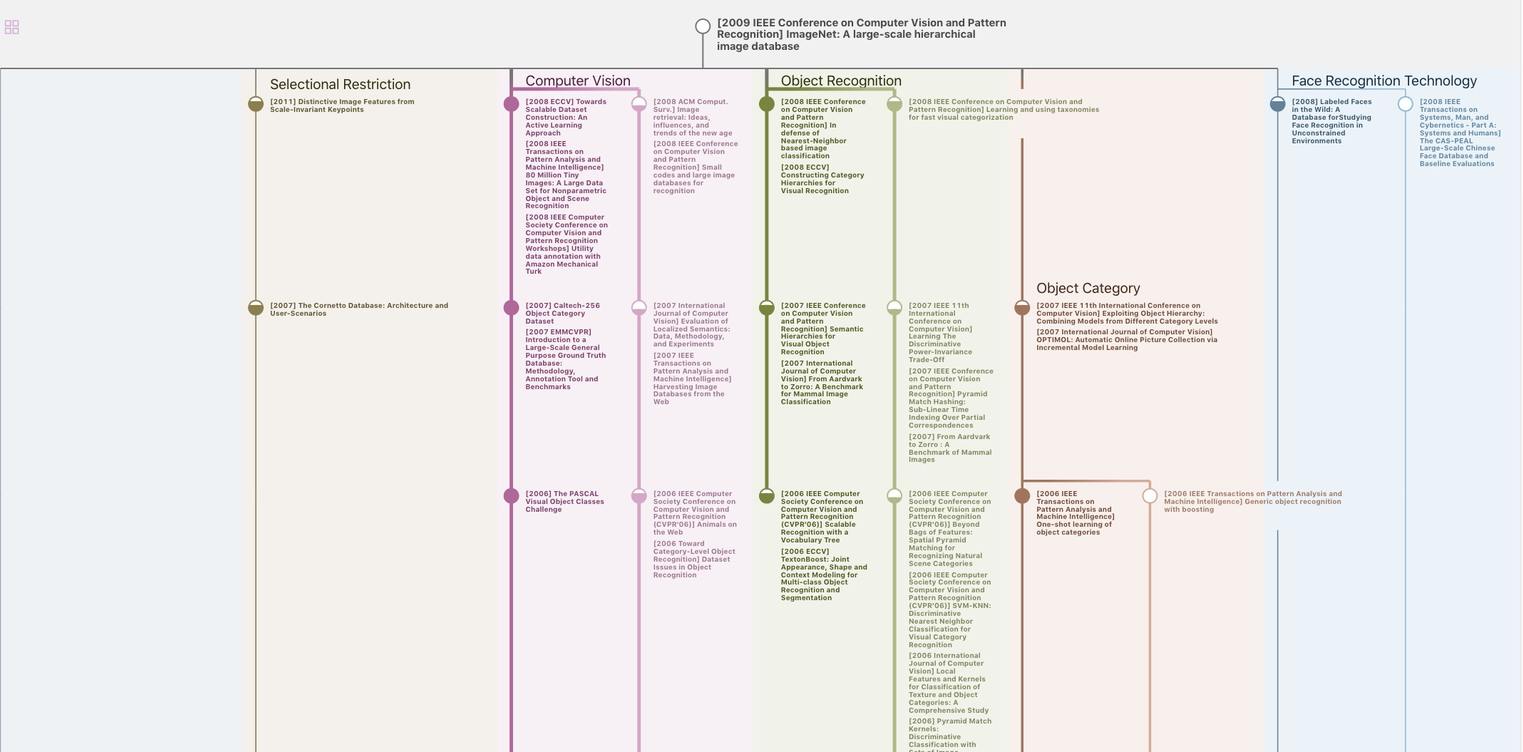
Generate MRT to find the research sequence of this paper
Chat Paper
Summary is being generated by the instructions you defined