Deep learning enables non-invasive estimation of mean pulmonary arterial pressure from PREFUL-MRI
Imaging(2023)
摘要
Introduction: Mean pulmonary arterial pressure (mPAP) is a biomarker of pulmonary hypertension (PHT), which is currently determined by right heart catheterization (RHC) as the clinical standard. Phase-resolved functional lung MRI (PREFUL) is a non-invasive imaging technique, which can calculate the pulse wave propagation in pulmonary vasculature during a virtual cardiac cycle of 15 phases. We hypothesize that deep learning tools may be able to estimate mPAP from dynamic pulse wave propagation images derived by PREFUL. Method: 92 (f=48, PHT=57) subjects underwent PREFUL and RHC. Per patient, pulse wave propagation images of coronal, central slice as well as the next ventral and dorsal location in the thorax were combined as input data. mPAP given by RHC was defined as target output data. Finally, a combination of three Denset-201 with one regression network was trained to realize the aforementioned mapping with 60 subjects, tested with 32 remaining cases. The correlation of the network’s final output with the mPAP from RHC was measured using Pearson’s Correlation. Results: For both training and holdout test data, final output of our method showed a significant correlation with RHC (p<0.05). Conclusions: Non-invasive measurement of mPAP using PREFUL-MRI and deep learning appears feasible and needs to be further explored.
更多查看译文
关键词
mean pulmonary arterial pressure,deep learning,non-invasive,preful-mri
AI 理解论文
溯源树
样例
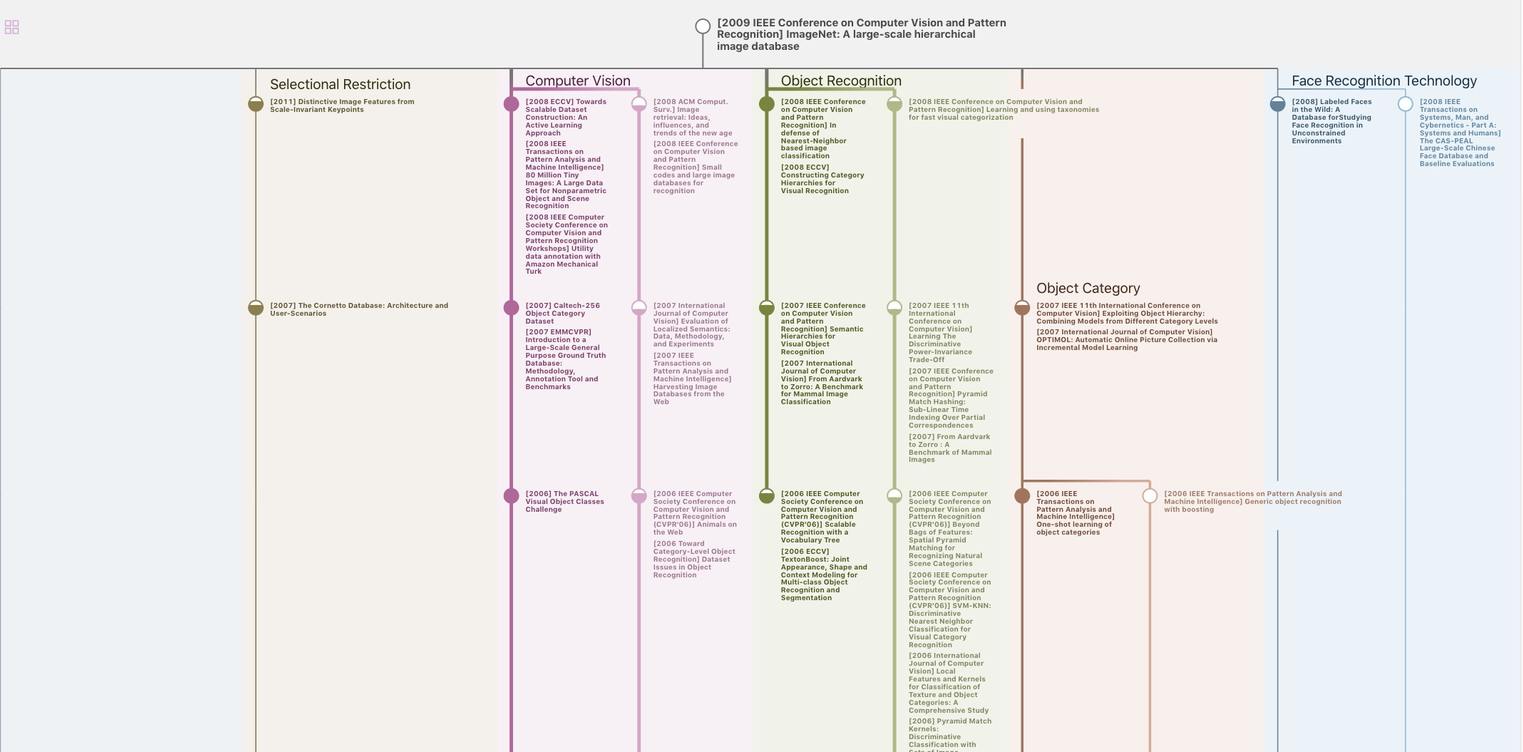
生成溯源树,研究论文发展脉络
Chat Paper
正在生成论文摘要