FedSH: Towards Privacy-Preserving Text-Based Person Re-Identification
IEEE TRANSACTIONS ON MULTIMEDIA(2024)
摘要
Text-based person re-identification (ReID) has enabled canonical applications in searching for and tracking targets from large-scale surveillance images with textual descriptions. Yet, existing text-based person ReID systems employ centralized model training that gathers images captured by different institutes' cameras into one place, which poses severe privacy threats to sensitive institutional information. This work is then devoted to exploring privacy-preserving text-based person ReID and proposes the framework of FedSH by tailoring the federated learning paradigm for distributed searching knowledge extraction. Specifically, FedSH resolves the local model generalization and entity boundary obscuring limitations, caused by inner-institute data homogeneity and inter-institute data heterogeneity, via building multi-granularity feature representation and a semantically self-aligned network. Meanwhile, it reduces the communication burden introduced by the embedding for multiple modals by updating common representation subspaces during federated learning. Experimental results on two public benchmarks demonstrate that our method can achieve at most 16.47% and 16.02% person ReID performance improvement by the Rank-1 metric, compared with 6 State-of-The-Art (SoTA) baselines and 6 ablation studies. We believe that our work will inspire the community to investigate the potential of implementing Federated Learning in real-world image retrieval and ReID scenarios.
更多查看译文
关键词
Semantics,Training,Task analysis,Privacy,Visualization,Federated learning,Servers,Text-based Person ReID,Cross-modal Retrieval,Federated Learning,Multi-granularity Representation
AI 理解论文
溯源树
样例
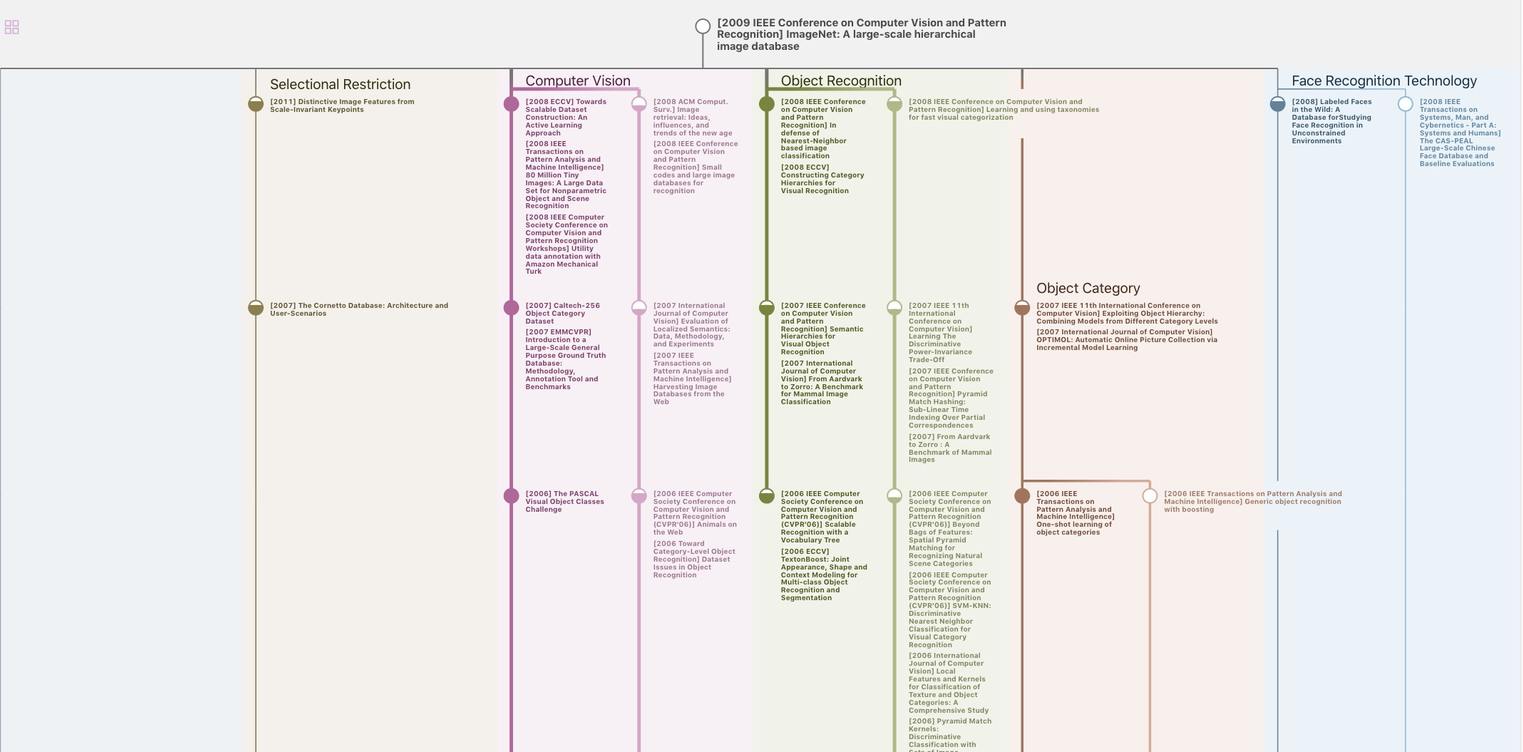
生成溯源树,研究论文发展脉络
Chat Paper
正在生成论文摘要