Harnessing Voice Analysis and Machine Learning for Early Diagnosis of Parkinson's Disease: A Comprehensive Study Across Diverse Datasets
Research Square (Research Square)(2023)
摘要
Abstract Objective To evaluate the efficacy of integrating voice analysis with machine learning techniques for the early diagnosis of Parkinson's Disease (PD) across diverse datasets. Methods Voice data were sourced from three distinct datasets available on the UCI Machine Learning Repository. These datasets encompassed voice measurements from various PD patients and healthy individuals, characterized by different voice recording exercises and conditions and including time and spectral voice features. Machine learning models were trained and validated using these features to differentiate between PD patients and healthy subjects. Results Our machine learning model demonstrated high diagnostic accuracy across all datasets. Specifically, the model achieved promising indicators of efficacy, including high averages across datasets of accuracy (99% ± 3.9%), sensitivity (98.8% ± 5.3%), specificity (99.1% ± 5.1%), precision (98.5% ± 4.2%), F1 score (97.9% ± 4.9%), and ROC AUC (99.3% ± 2.7%). The results were consistent across datasets, highlighting the model's robustness and adaptability. Conclusion The integration of voice analysis with machine learning offers a promising avenue for the early diagnosis of PD. Given the non-invasive nature and cost-efficiency of voice analysis, this approach could revolutionize early PD detection and monitoring. While the preliminary results are encouraging, further validation in clinical settings and larger cohorts is essential before widespread adoption.
更多查看译文
关键词
parkinson,voice,machine learning,early diagnosis,diverse datasets
AI 理解论文
溯源树
样例
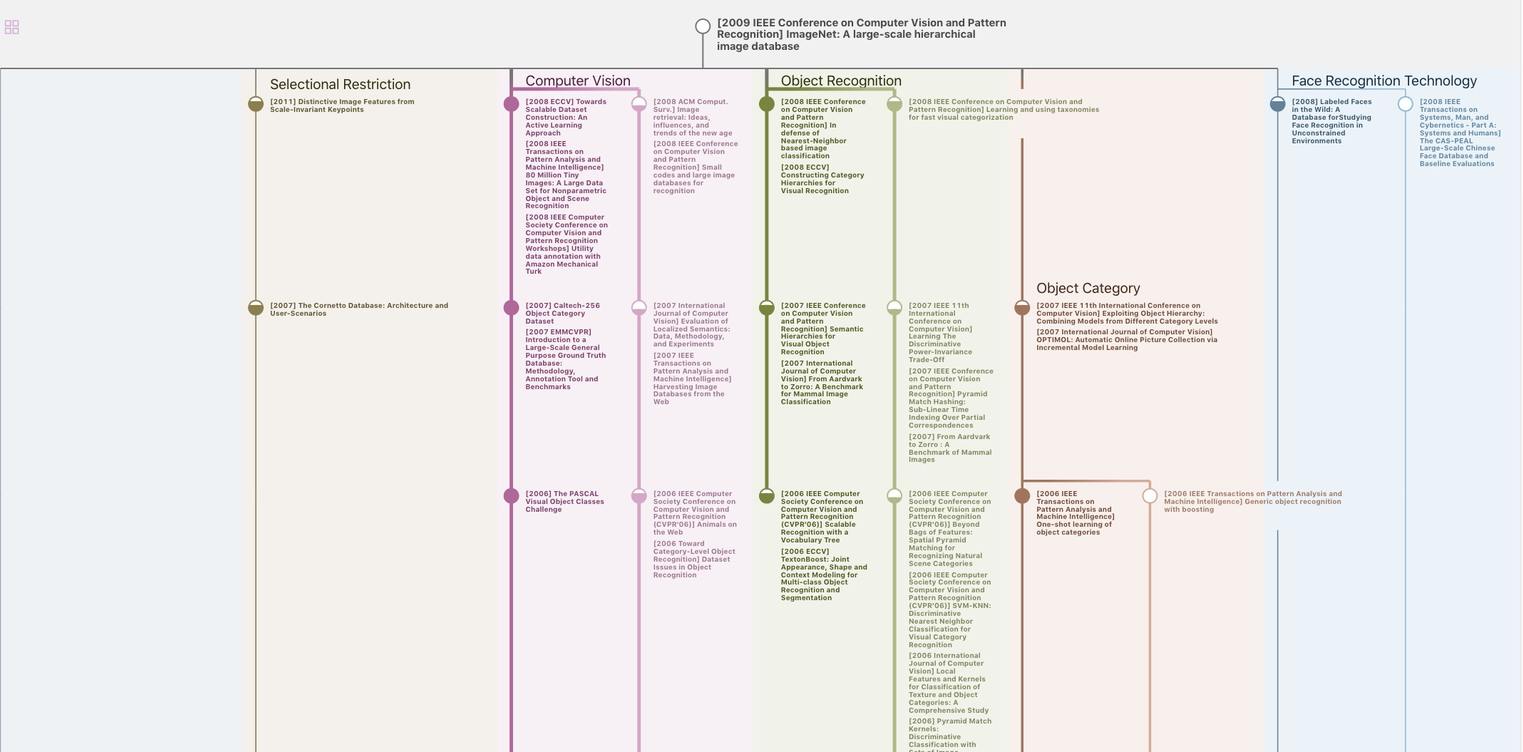
生成溯源树,研究论文发展脉络
Chat Paper
正在生成论文摘要