Joint Sparsity and Low-Rank Minimization for Reconfigurable Intelligent Surface-Assisted Channel Estimation
IEEE Transactions on Communications(2024)
摘要
Reconfigurable intelligent surfaces (RISs) have attracted extensive attention in millimeter wave (mmWave) systems because of the capability of configuring the wireless propagation environment. However, due to the existence of a RIS between the transmitter and receiver, a large number of channel coefficients need to be estimated, resulting in more pilot overhead. In this paper, we propose a joint sparse and low-rank based two-stage channel estimation scheme for RIS-assisted mmWave systems. Specifically, we first establish a low-rank approximation model against the noisy channel, fitting in with the precondition of the compressed sensing theory for perfect signal recovery. To overcome the difficulty of solving the low-rank problem, we propose a trace operator to replace the traditional nuclear norm operator, which can better approximate the rank of a matrix. Furthermore, by utilizing the sparse characteristics of the mmWave channel, sparse recovery is carried out to estimate the RIS-assisted channel in the second stage. Simulation results show that the proposed scheme achieves significant performance gain in terms of estimation accuracy compared to the benchmark schemes.
更多查看译文
关键词
Channel estimation,reconfigurable intelligent surface,millimeter wave,compressed sensing,sparse and low-rank
AI 理解论文
溯源树
样例
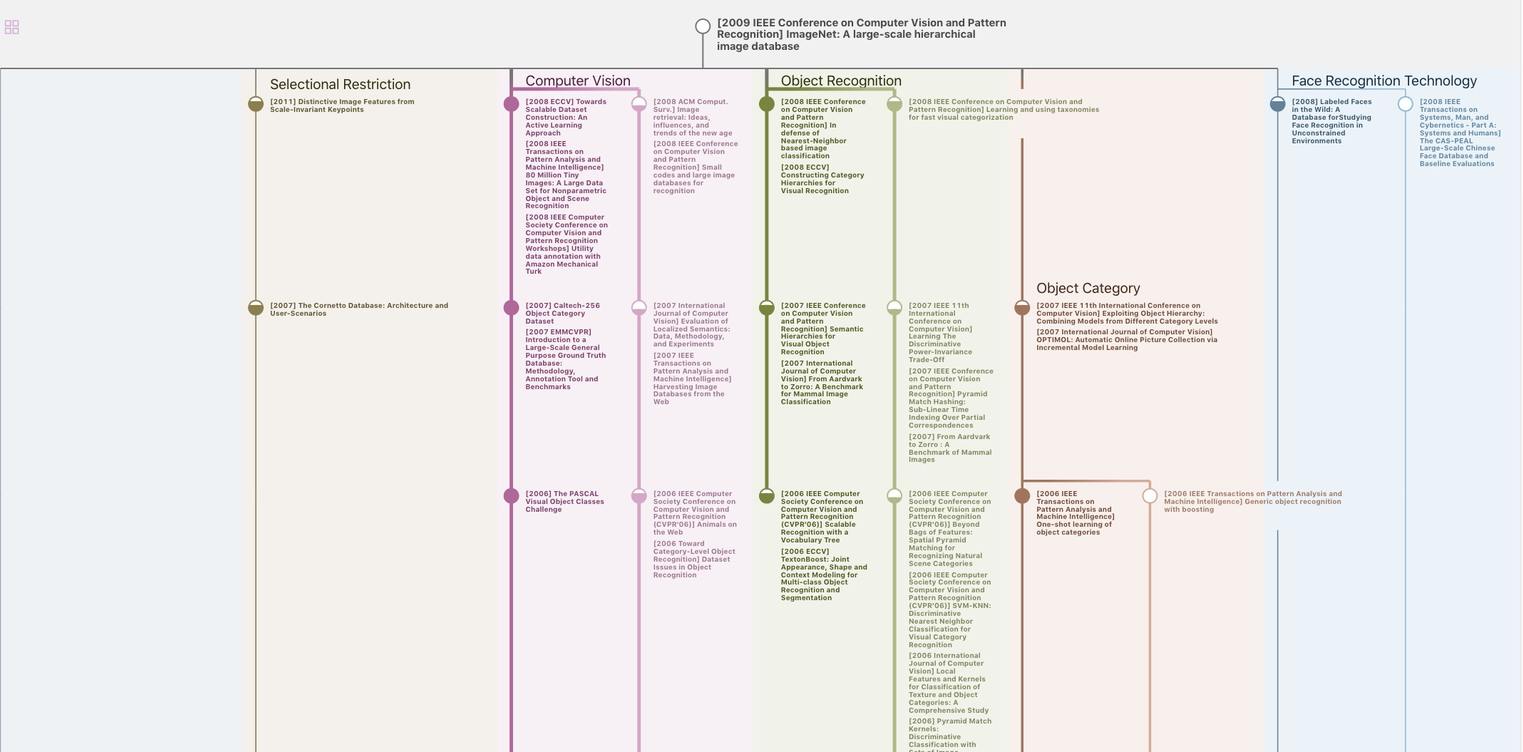
生成溯源树,研究论文发展脉络
Chat Paper
正在生成论文摘要