Self Supervised Cloud Classification
Artificial Intelligence for the Earth Systems(2023)
摘要
Low-level marine clouds play a pivotal role in Earth’s weather and climate through their interactions with radiation, heat and moisture transport, and the hydrological cycle. These interactions depend on a range of dynamical and microphysical processes that result in a broad diversity of cloud types and spatial structures, and a comprehensive understanding of cloud morphology is critical for continued improvement of our atmospheric modeling and prediction capabilities moving forward. Deep learning has recently accelerated our ability to study clouds using satellite remote sensing, and machine learning classifiers have enabled detailed studies of cloud morphology. A major limitation of deep learning approaches to this problem, however, is the large number of hand-labeled samples that are required for training. This work applies a recently developed self-supervised learning scheme to train a deep convolutional neural network (CNN) to map marine cloud imagery to vector embeddings that capture information about mesoscale cloud morphology and can be used for satellite image classification. The model is evaluated against existing cloud classification datasets and several use cases are demonstrated, including: training cloud classifiers with very few labeled samples, interrogation of the CNN’s learned internal feature representations, cross-instrument application, and resilience against sensor calibration drift and changing scene brightness. The self-supervised approach learns meaningful internal representations of cloud structures and achieves comparable classification accuracy to supervised deep learning methods without the expense of creating large hand-annotated training datasets.
更多查看译文
AI 理解论文
溯源树
样例
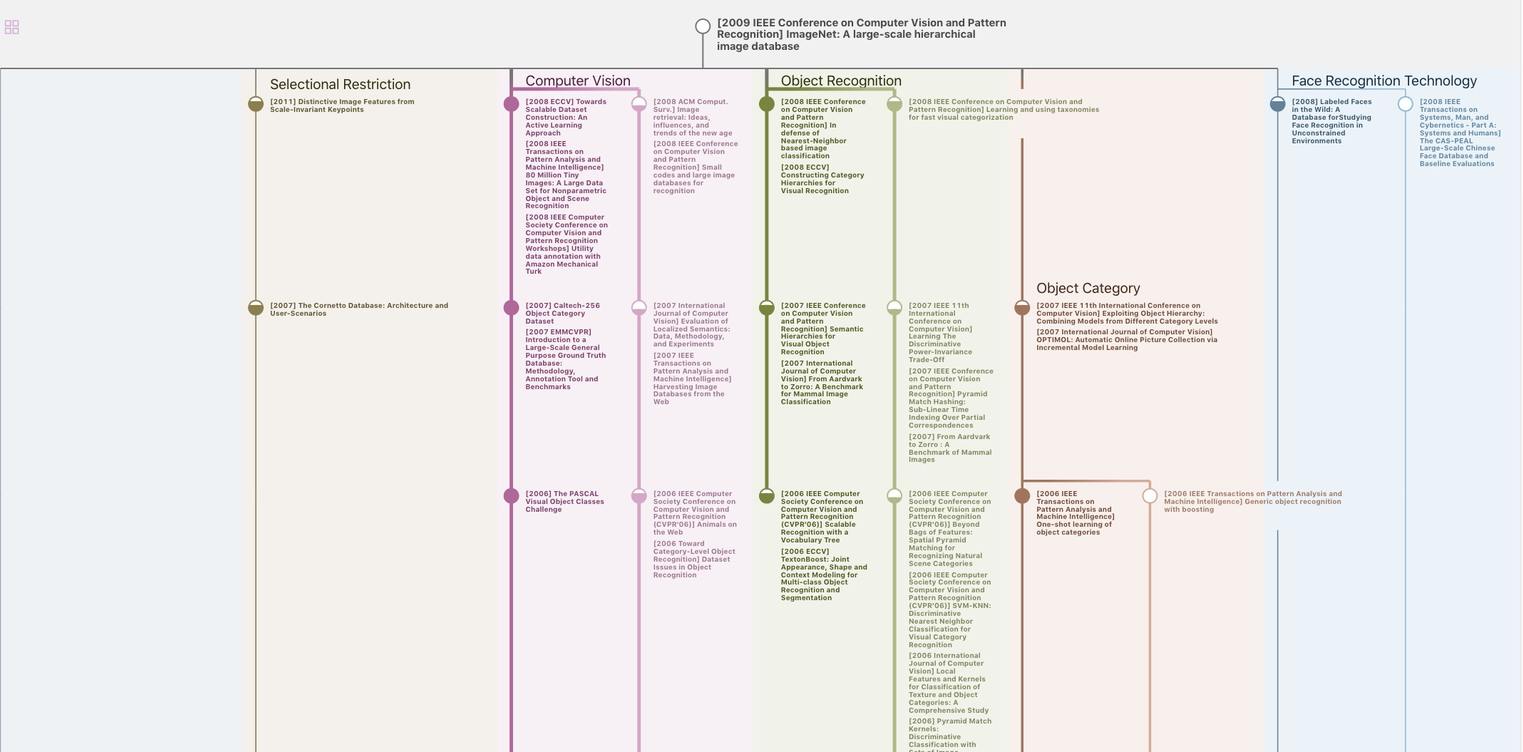
生成溯源树,研究论文发展脉络
Chat Paper
正在生成论文摘要