Interpretable Machine Learning-based Individual Analysis of Acute Kidney Injury in Immune Checkpoint Inhibitor Therapy
Research Square (Research Square)(2023)
摘要
Abstract Acute kidney injury (AKI) is a critical complication in immune checkpoint inhibitor (ICI) therapy. Although risk factors of AKI in ICI therapy have been extensively studied, individualized and real-time assessment of AKI risk has not been achieved. We aimed to clarify the underlying causes of individual AKI in ICI-treated patients using a novel time-series clustering approach with Shapley Additive exPlanations (SHAP) values and decision tree-based machine learning (ML) prediction models. The medical records of 616 cancer patients receiving ICI therapy were used for model construction, and 112 patients (18.2%) had at least one AKI episode. They were clustered per key features and their SHAP value patterns, and three nephrologists assessed the clusters’ clinical relevance. Receiver operating characteristic analysis revealed that the area under the curve was 0.880. Patients with AKI were categorized into four clusters with significant prognostic differences (p = 0.010). The leading causes of AKI (such as hypovolemia, drug-related, and cancer cachexia) for each cluster were interpretable by nephrologists. These results suggest that our clustering method of time-series SHAP values can clarify the underlying background of AKI in ICI-treated patients and possibly be used for personalized AKI risk assessment and early intervention for improved outcomes.
更多查看译文
关键词
acute kidney injury,learning-based
AI 理解论文
溯源树
样例
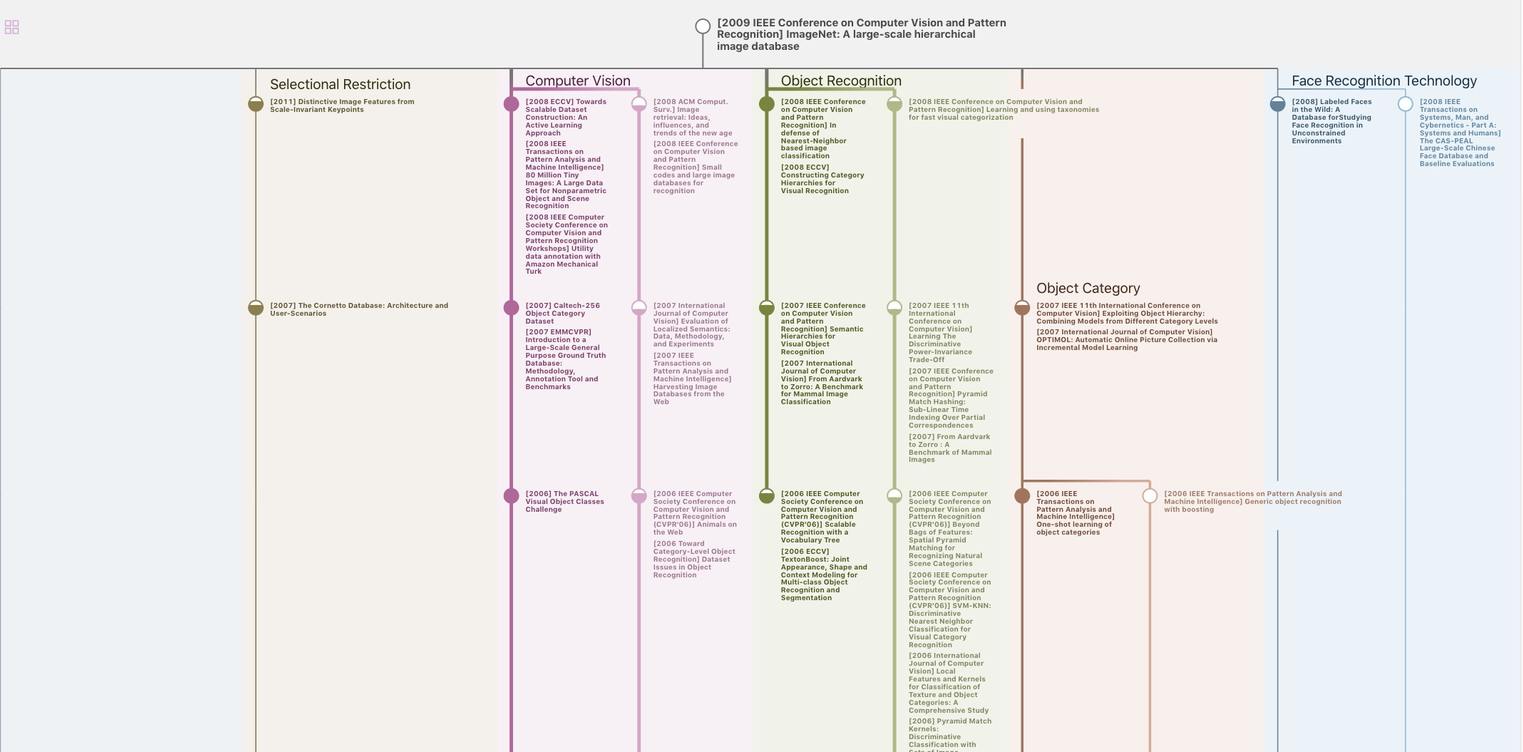
生成溯源树,研究论文发展脉络
Chat Paper
正在生成论文摘要