Transductive Learning With Prior Knowledge for Generalized Zero-Shot Action Recognition
IEEE TRANSACTIONS ON CIRCUITS AND SYSTEMS FOR VIDEO TECHNOLOGY(2024)
摘要
It is challenging to achieve generalized zero-shot action recognition. Different from the conventional zero-shot tasks which assume that the instances of the source classes are absent in the test set, the generalized zero-shot task studies the case that the test set contains both the source and the target classes. Due to the gap between visual feature and semantic embedding as well as the inherent bias of the learned classifier towards the source classes, the existing generalized zero-shot action recognition approaches are still far less effective than traditional zero-shot action recognition approaches. Facing these challenges, a novel transductive learning with prior knowledge (TLPK) model is proposed for generalized zero-shot action recognition. First, TLPK learns the prior knowledge which assists in bridging the gap between visual features and semantic embeddings, and preliminarily reduces the bias caused by the visual-semantic gap. Then, a transductive learning method that employs unlabeled target data is designed to overcome the bias problem in an effective manner. To achieve this, a target semantic-available approach and a target semantic-free approach are devised to utilize the target semantics in two different ways, where the target semantic-free approach exploits prior knowledge to produce well-performed semantic embeddings. By exploring the usage of the aforementioned prior-knowledge learning and transductive learning strategies, TLPK significantly bridges the visual-semantic gap and alleviates the bias between the source and the target classes. The experiments on the benchmark datasets of HMDB51 and UCF101 demonstrate the effectiveness of the proposed model compared to the state-of-the-art methods.
更多查看译文
关键词
Action recognition,generalized zero-shot learning,transductive learning,transfer learning,semantic embedding
AI 理解论文
溯源树
样例
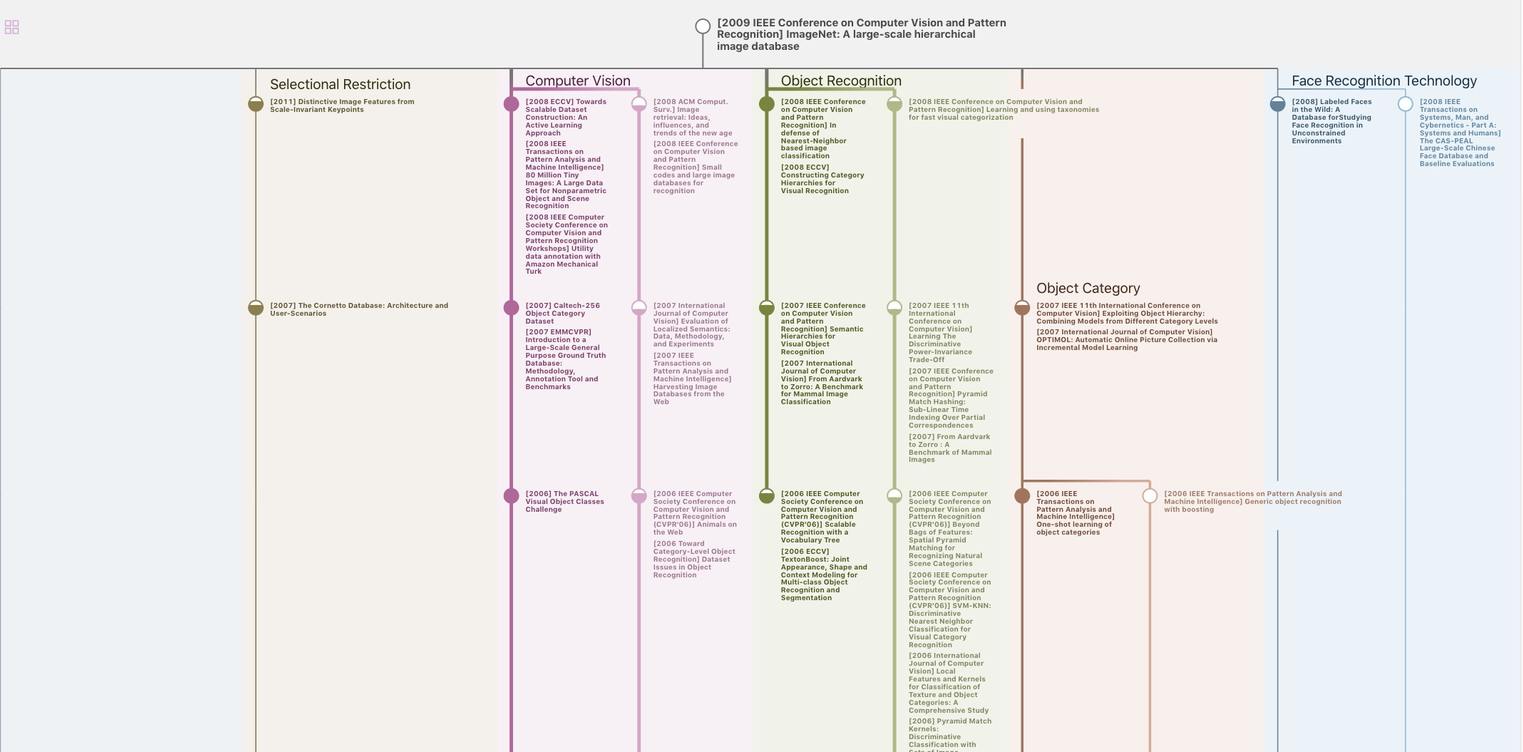
生成溯源树,研究论文发展脉络
Chat Paper
正在生成论文摘要