FSRD: few-shot fuzzy rumor detection system with stance-enhanced prompt learning
Soft Computing(2024)
摘要
Rumor detection is a classification task that utilizes information including the content of a rumor, the comments surrounding it, and information about the users involved. In existing social networks, often emerge unexpectedly and typically concern unknown events, prompting researchers to explore tasks in low-resource situations, including early rumor detection and unknown rumor detection. Existing approaches require constructing rumor representations based on the entire propagation cycle of rumors or specific features of current events. They can lead to models that are too dependent on existing data features and lack generality in low-resource situations and are unsuitable for ever-changing real-world environments. This paper proposes prompt-learning based on few-shot and zero-shot rumor fuzzy detection systems FSRD. These two approaches can detect rumors in a variety of low-resource scenarios (e.g., early rumor detection and unknown rumor detection) using only a small amount of source rumors aided by highly explainable prompt templates, without waiting for rumors to spread to obtain more features. On several standard rumor detection datasets, FSRD outperforms various other detection methods for few-shot scenarios, and achieves competitive results on zero-shot tasks as well.
更多查看译文
关键词
Rumor detection,Prompt learning,Pre-trained model,Few-shot learning,Early rumor detection,Unknown rumor event detection
AI 理解论文
溯源树
样例
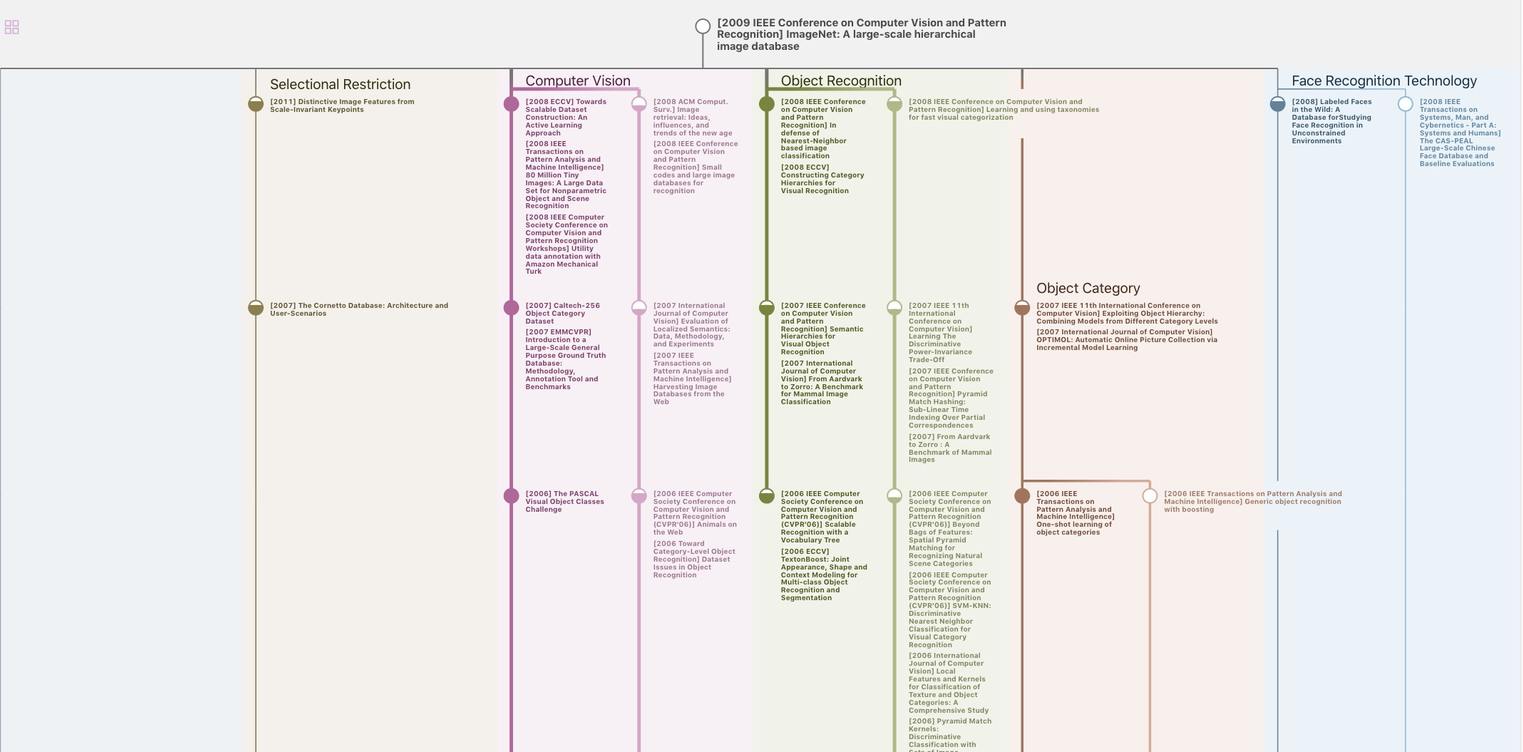
生成溯源树,研究论文发展脉络
Chat Paper
正在生成论文摘要