Survival Machine Learning Methods for Mortality Prediction After Heart Transplantation
Research Square (Research Square)(2023)
摘要
Although prognostic models for heart transplantation (HTx) have been developed, a comprehensive benchmarking of survival machine learning methods for mortality prognosis has not been performed. Futhermore, assessing mortality in the most contemporary era of heart transplants following the 2018 donor heart allocation policy change is warranted. This study included 7,160 adult heart-only transplant recipients in the Scientific Registry of Transplant Recipients database who received their first transplant on or after October 18, 2018, with at least one recorded follow-up visit until June 3, 2021. Each model was trained using a nested 5-repeat, 5-fold cross-validation. Seven statistical and machine learning algorithms – Lasso, Ridge, Elastic Net, Gradient Boost, Extreme Gradient Boost Linear, Extreme Gradient Boost Tree, and Random Survival Forests were benchmarked against Cox PH in both post-policy and pre-policy eras. In the post-policy era, there was higher discriminatory power of machine learning models for one-year all-cause mortality compared to Cox. Bilirubin, age, BMI, total ischemic time, donor age, and history of ischemic cardiomyopathy were some of the most predictive selected variables. ECMO was highly predictive in the pre-policy cohort, but less so in the post-policy cohort. Machine learning (particularly tree-based ensemble) survival models can be used to capture complex short-term posttransplant mortality outcomes.
更多查看译文
关键词
mortality prediction,heart transplantation,machine learning methods,survival
AI 理解论文
溯源树
样例
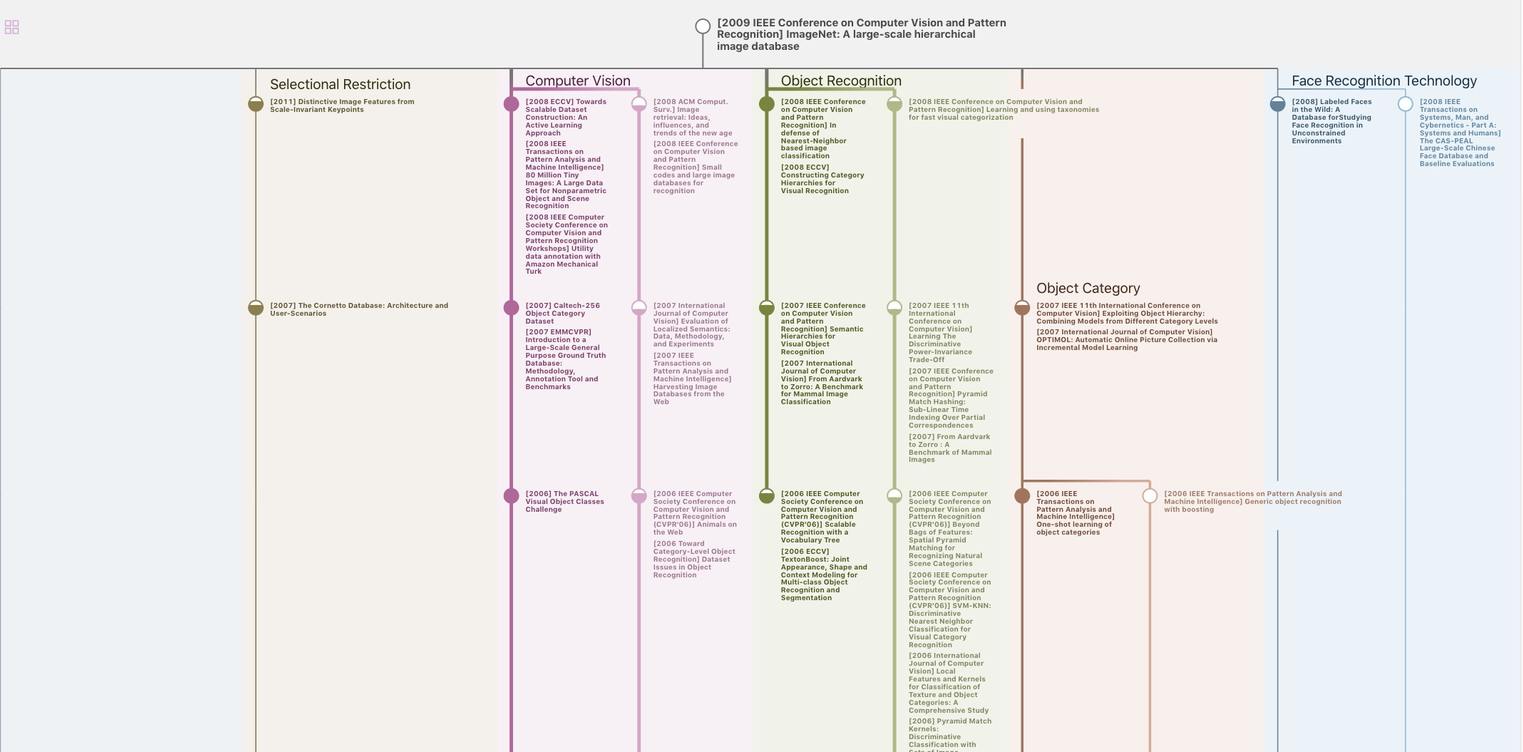
生成溯源树,研究论文发展脉络
Chat Paper
正在生成论文摘要