Probable Deviation Outlier-Based Classification of Obesity with Eating Habits and Physical Condition
Smart innovation, systems and technologies(2023)
摘要
Obesity is harmful because it would be associated to poor mental health and lower living standards. Obesity has also been linked to several of the world’s most common causes of death, including diabetes, heart disease, stroke and certain types of cancer. Obesity is a serious health problem that can be influenced by a multitude of factors, including genetics and behavior. Because the original cause of obesity is unknown, determining the levels of obesity remains a difficult task for doctors and researchers. With this overview, this project uses machine learning algorithms to identify obesity levels based on eating habits and physical condition. The obesity dataset from the KAGGLE database repository is used for implementation for the classification of obesity based on eating habits and physical condition. The obesity dataset contains 17 features and 2111 patient details and it is preprocessed with encoding and missing values. To analyze the performance metrics, the original dataset is used with all classifiers, both with and without feature scaling. The exploratory data analysis is done to figure out how the target obesity variable is distributed. The dataset is analyzed with the scatterplot to detect the features that are having outliers. From the implementation, it is observed that the features age and weight have outliers. The dataset is applied with outlier detection methods like interquartile range and standard deviation to balance the outliers in age and weight features. The outlier is removed to form the following dataset as age outlier removed using STD, age outlier removed using IQR, weight outlier removed using STD, age and weight outlier removed using STD, age and weight outlier removed using IQR. The above outlier removed datasets are applied with various classifiers to predict the obesity levels before and after feature scaling to analyze the performances indices precision, recall, f score and accuracy. Experimental results shows that the Decision Tree classifier shows accuracy of 94% before removing outliers on age and weight feature. The same Decision Tree classifier shows the accuracy of 96% after removing outliers on age and weight feature.
更多查看译文
关键词
eating habits,obesity,classification,deviation,outlier-based
AI 理解论文
溯源树
样例
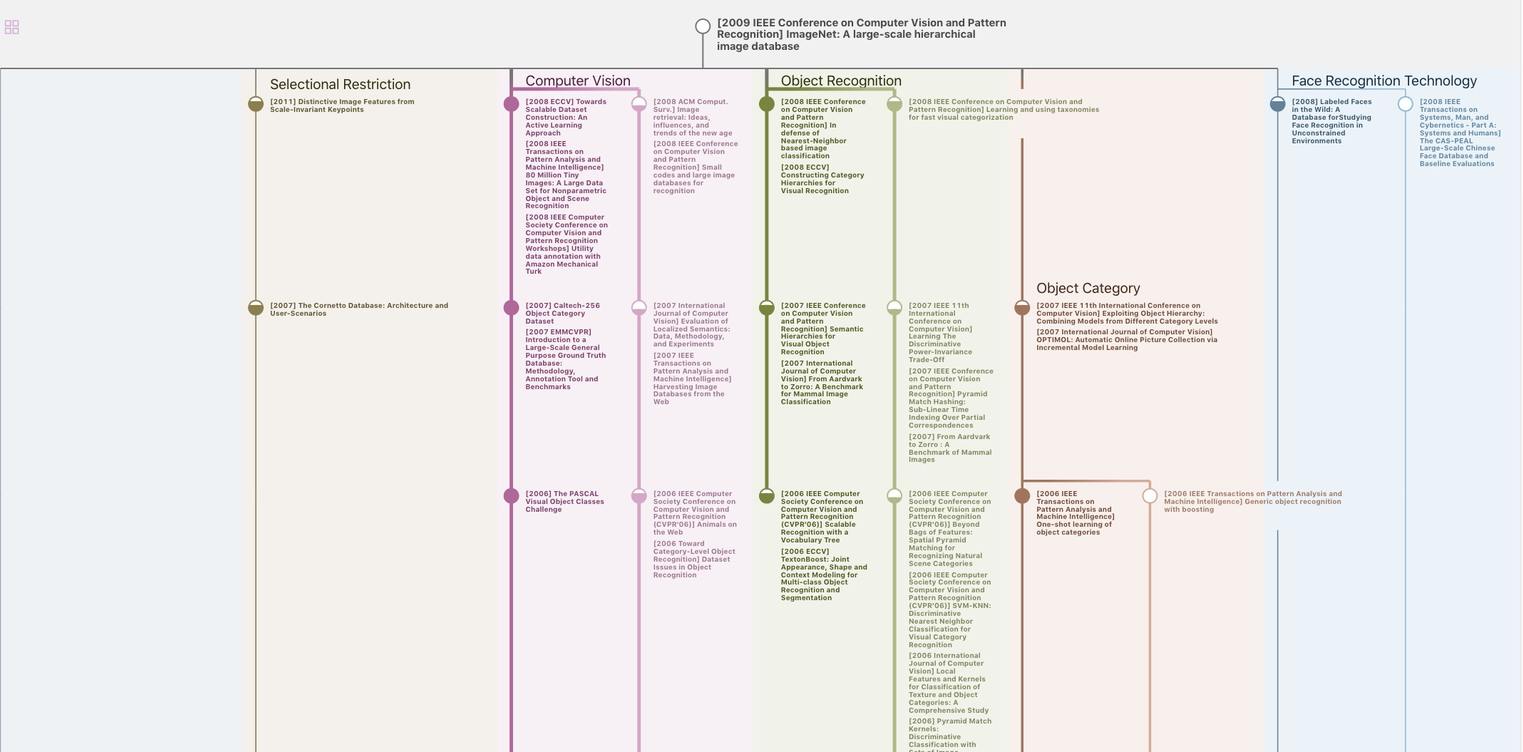
生成溯源树,研究论文发展脉络
Chat Paper
正在生成论文摘要