The usefulness of machine-learning-based evaluation of clinical and pretreatment 18F-FDG-PET/CT radiomic features for predicting prognosis in patients with laryngeal cancer
British Journal of Radiology(2023)
摘要
Objective: To examine whether machine learning (ML) analyses involving clinical and 18 F-FDG-PET-based radiomic features are helpful in predicting prognosis in patients with laryngeal cancer. Methods: This retrospective study included 49 patients with laryngeal cancer who underwent 18 F-FDG-PET/CT before treatment, and these patients were divided into the training (n = 34) and testing (n = 15) cohorts.Seven clinical (age, sex, tumor size, T stage, N stage, Union for International Cancer Control stage, and treatment) and 40 18 F-FDG-PET–based radiomic features were used to predict disease progression and survival. Six ML algorithms (random forest, neural network, k-nearest neighbors, naïve Bayes, logistic regression, and support vector machine) were used for predicting disease progression. Two ML algorithms (cox proportional hazard and random survival forest [RSF] model) considering for time-to-event outcomes were used to assess progression-free survival (PFS), and prediction performance was assessed by the concordance index (C-index). Results: Tumor size, T stage, N stage, GLZLM_ZLNU, and GLCM_Entropy were the five most important features for predicting disease progression.In both cohorts, the naïve Bayes model constructed by these five features was the best performing classifier (training: AUC = 0.805; testing: AUC = 0.842). The RSF model using the five features (tumor size, GLZLM_ZLNU, GLCM_Entropy, GLRLM_LRHGE and GLRLM_SRHGE) exhibited the highest performance in predicting PFS (training: C-index = 0.840; testing: C-index = 0.808). Conclusion: ML analyses involving clinical and 18 F-FDG-PET–based radiomic features may help predict disease progression and survival in patients with laryngeal cancer. Advances in knowledge: ML approach using clinical and 18 F-FDG-PET–based radiomic features has the potential to predict prognosis of laryngeal cancer.
更多查看译文
关键词
laryngeal cancer,machine-learning-based machine-learning-based,prognosis,predicting,f-fdg-pet
AI 理解论文
溯源树
样例
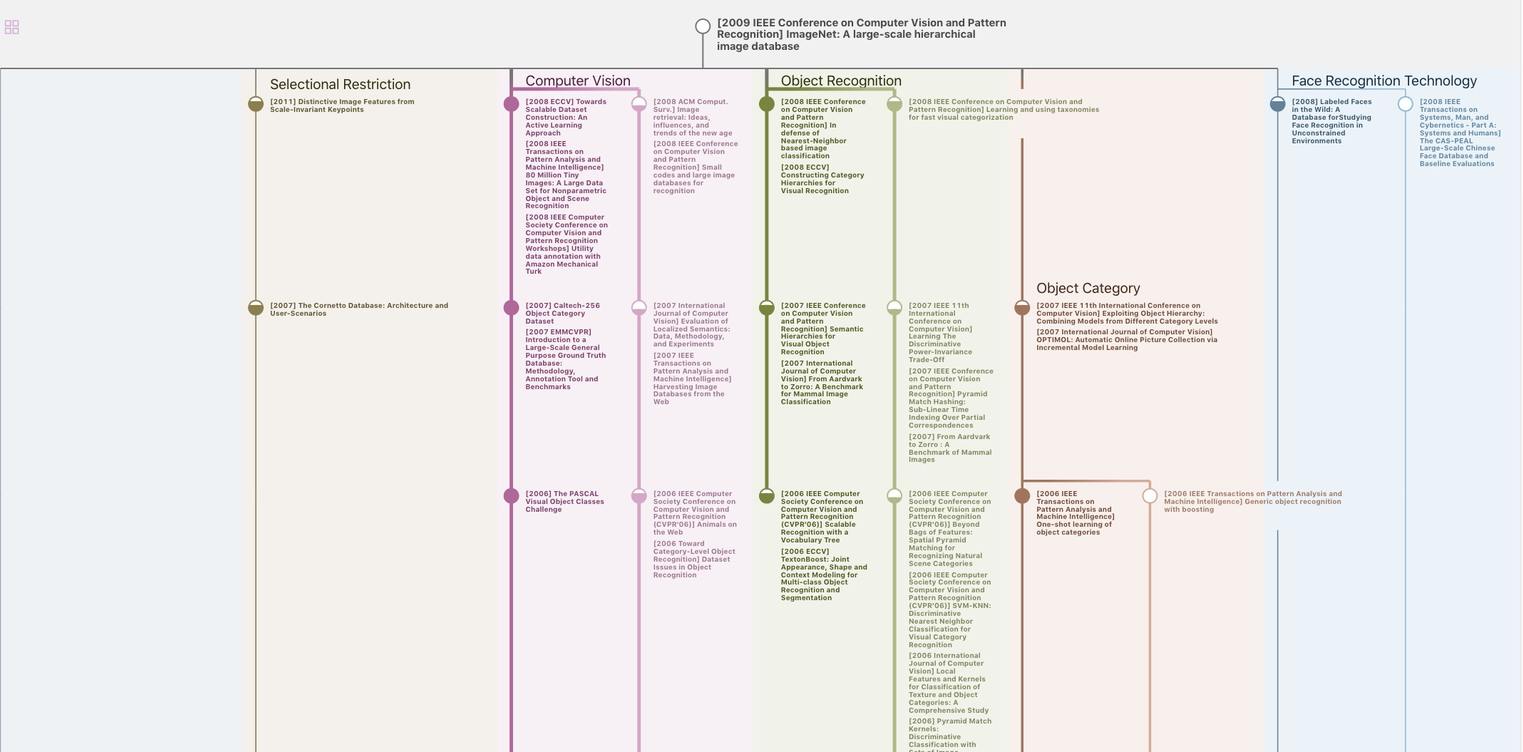
生成溯源树,研究论文发展脉络
Chat Paper
正在生成论文摘要