Lightweight Privacy-Preserving Feature Extraction for EEG Signals Under Edge Computing
IEEE INTERNET OF THINGS JOURNAL(2024)
摘要
The health-related Internet of Things (IoT) plays an irreplaceable role in the collection, analysis, and transmission of medical data. As a device of the health-related IoT, the electroencephalogram (EEG) has long been a powerful tool for physiological and clinical brain research, which contains a wealth of personal information. Due to its rich computational/storage resources, cloud computing is a promising solution to extract the sophisticated feature of massive EEG signals in the age of big data. However, it needs to solve both response latency and privacy leakage. To reduce latency between users and servers while ensuring data privacy, we propose a privacy-preserving feature extraction scheme, called LightPyFE, for EEG signals in the edge computing environment. In this scheme, we design an outsourced computing toolkit, which allows the users to achieve a series of secure integer and floating-point computing operations. During the implementation, LightPyFE can ensure that the users just perform the encryption and decryption operations, where all computing tasks are outsourced to edge servers for specific processing. Theoretical analysis and experimental results have demonstrated that our scheme can successfully achieve privacy-preserving feature extraction for EEG signals, and is practical yet effective.
更多查看译文
关键词
Additive secret sharing,edge computing,electroencephalogram (EEG) signal,Internet of Things (IoT),privacy-preserving
AI 理解论文
溯源树
样例
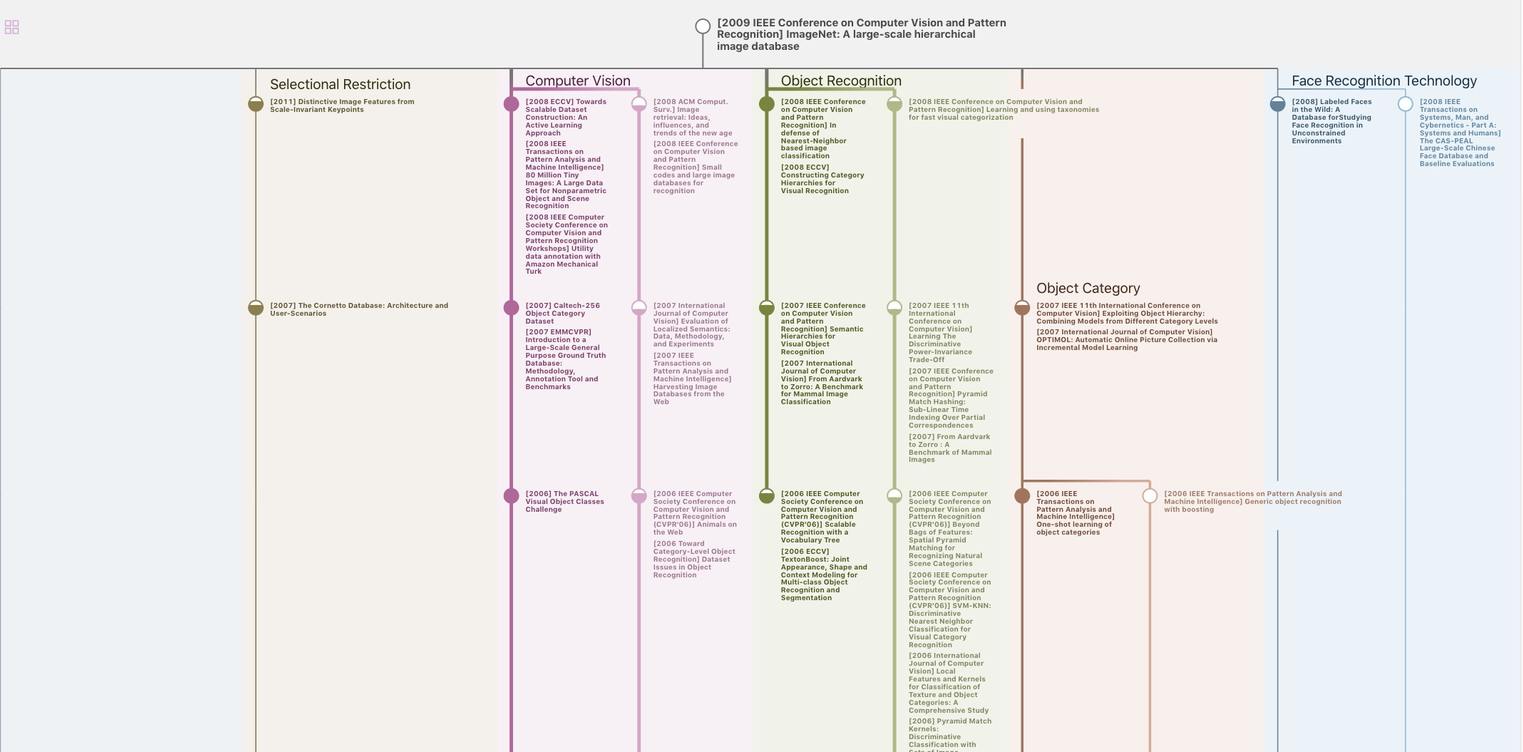
生成溯源树,研究论文发展脉络
Chat Paper
正在生成论文摘要