Self-Attention Enhanced Auto-Encoder for Link Weight Prediction with Graph Compression
IEEE transactions on network science and engineering(2024)
摘要
Predicting unobservable or missing weights over links on various real-world networks is of fundamental scientific significance in disparate disciplines like sociology, biology, and physics, as it has been revealed by scientists that link weights, representing strong or weak ties, are able to help us understand the mechanism of link formation, community growth, and network evolution. Nevertheless, previous studies mainly relied on shallow graph features to fulfill the task of link weight prediction and thus the proposed models have relatively poor predictive performance. By learning deep graph features to improve the predictive capability, a Self-attention Enhanced graph Auto-encoder called SEA is proposed. To resolve the challenge of the model's scalability for large graph applications, a two-phased link weight prediction framework is further proposed, which comprises the modules of graph compression and SEA. Experiments on seven real-world networks (four uncompressed networks and three compressed networks) demonstrated that SEA can achieve an average 6% improvement of accuracy in missing link weight prediction against state-of-the-art methods. Some extended experiments and analyses verified that the proposed framework can significantly reduce the network's size by 60%-80% while maintaining the predictive power.
更多查看译文
关键词
Predictive models,Proteins,Task analysis,Evolution (biology),Computational modeling,Visualization,Time complexity,Auto-encoder,graph compression,link weight prediction,self-attention mechanism
AI 理解论文
溯源树
样例
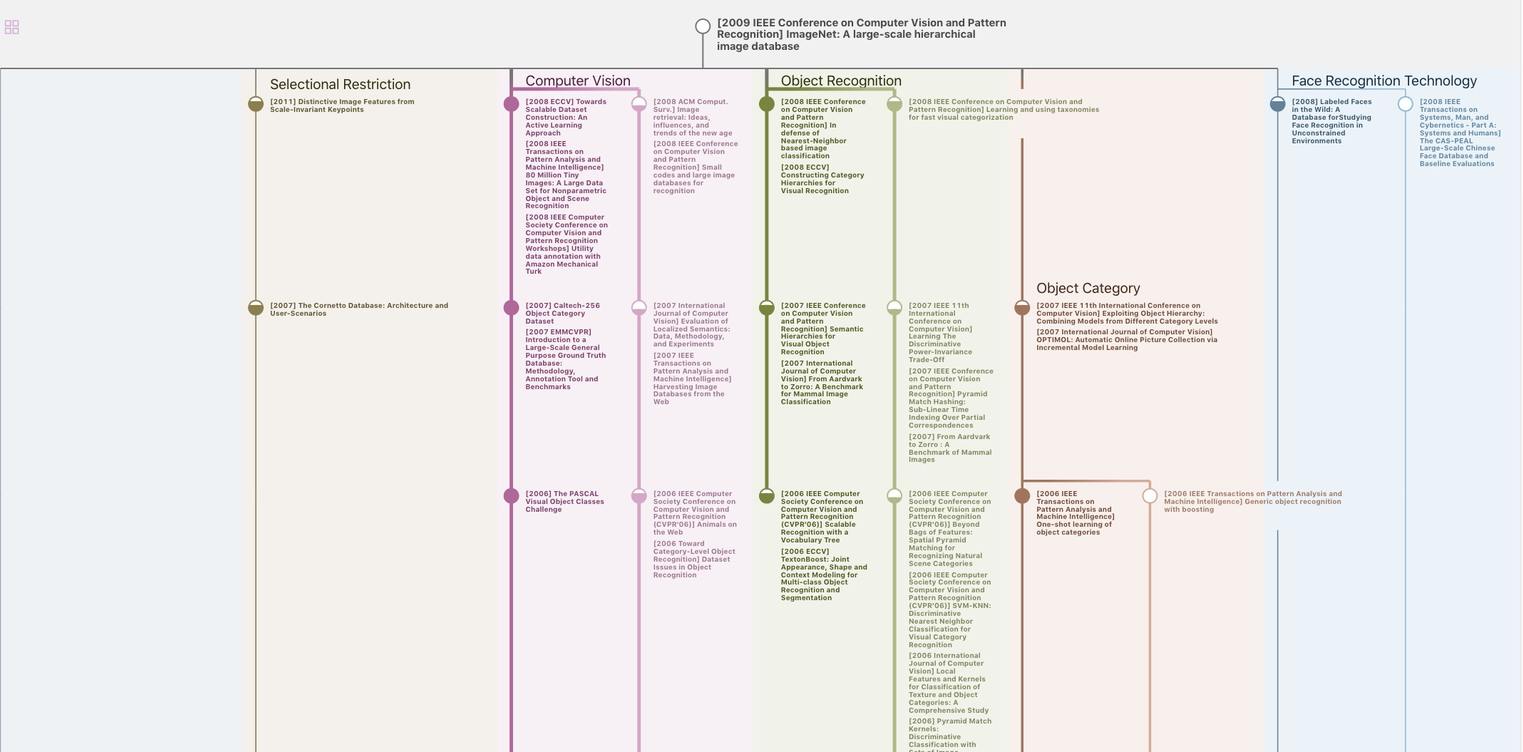
生成溯源树,研究论文发展脉络
Chat Paper
正在生成论文摘要