Clarifying the Meaning of Exposure-Response Curves with Causal AI and ML
International series in management science/operations research(2023)
摘要
Exposure-response curves are among the most widely used tools of quantitative health risk assessment. This chapter argues that what they mean is importantly ambiguous at a fundamental conceptual and definitional level. They leave unanswered such fundamental questions as whether and by how much reducing exposure would change average population risks and distributions of individual risks. The following sections show recent ideas from causal artificial intelligence (CAI) and machine learning (ML) can be applied to clarify what an exposure-response curve means, what other variables are held fixed in estimating it, and how much inter-individual variability there is around population average exposure-response curves. These advances in concepts and computational methods not only enable epidemiologists and risk analysis practitioners to quantify precisely defined population and individual exposure-response curves but also challenge them to make good use of this new capability by defining exactly what exposure-response relationships they want to quantify and communicate to risk managers and by specifying how to use the resulting information to improve risk management decisions.
更多查看译文
关键词
causal ai,exposure-response
AI 理解论文
溯源树
样例
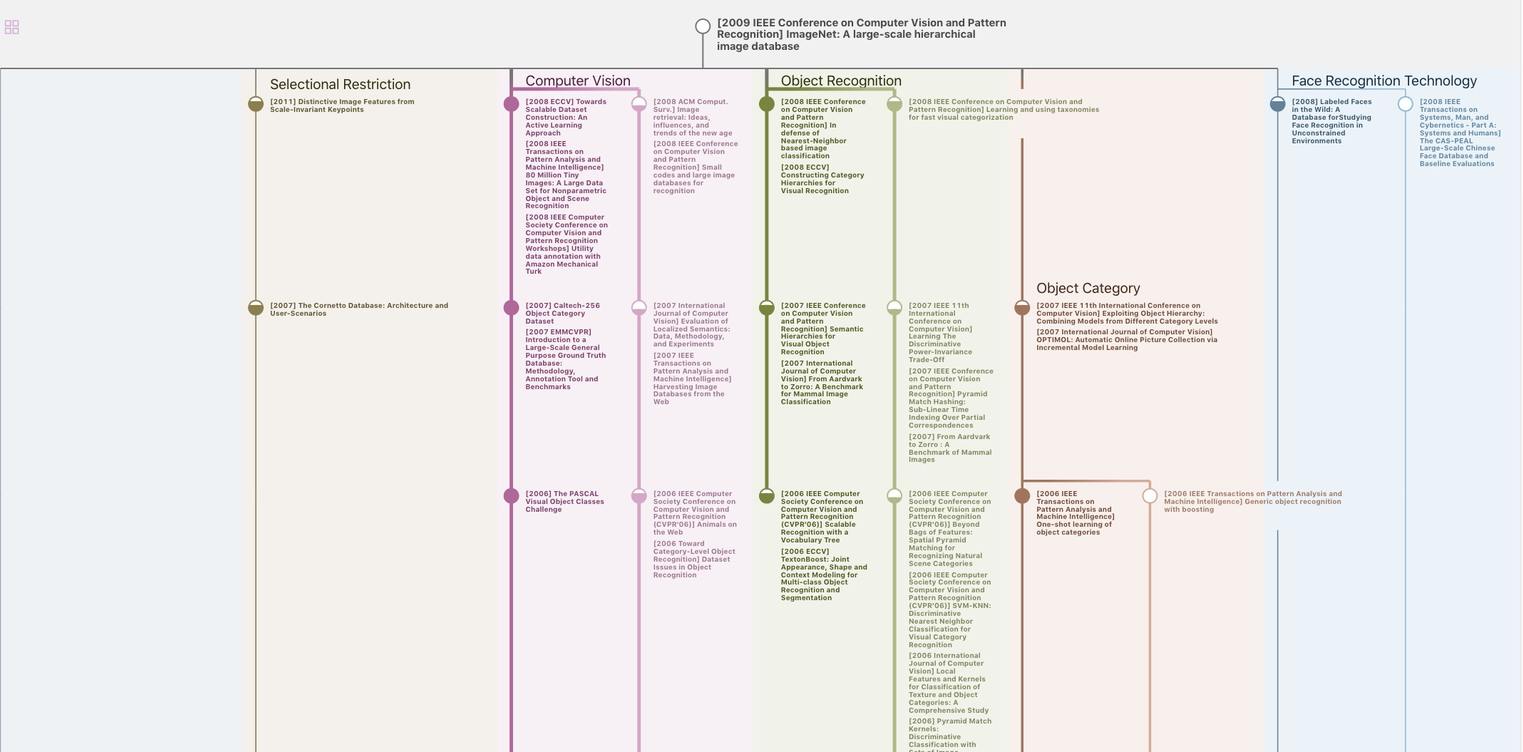
生成溯源树,研究论文发展脉络
Chat Paper
正在生成论文摘要