Utilizing Greedy Nature for Multimodal Conditional Image Synthesis in Transformers
IEEE TRANSACTIONS ON MULTIMEDIA(2024)
摘要
Multimodal Conditional Image Synthesis(MCIS) aims to generate images according to different modalities input and their combination, which allows users to describe their requirements in complementary ways, e.g. segmentation for shapes and text for attributes. Despite satisfying results in MCIS, a non-trivial issue is neglected. Some modalities are fully optimized and dominate the generation, while other modalities are sub-optimized and fail to contribute their complementary information. We coin this phenomenon as Modality Bias. Our analysis reveals that generative models own greedy nature. Specifically, the modality that shares less semantic gap with the synthesized modality will be greedily incorporated and thus takes a larger proportion in synthesis. The main idea of previous works in Modality Bias is to punish the greedy nature, which hurts the performance of dominant modalities and impedes their contribution to multimodal synthesis. Instead, we propose to utilize the greedy nature by setting dominant modalities as guidance for sub-optimized modalities through coordinated feature space, named Coordinated Knowledge Mining. Afterwards, improved uni-modalities are aggregated by fusing coordinated features to further boost the performance of multimodal image synthesis, called Coordinated Knowledge Fusion. Extensive experiments prove that our method not only increases uni-modal performance by a large margin, but also promotes multimodal image synthesis by fully utilizing complementary information from different modalities.
更多查看译文
关键词
Transformers,Image synthesis,Visualization,Image segmentation,Task analysis,Image reconstruction,Computer architecture,multimodal learning,transformer
AI 理解论文
溯源树
样例
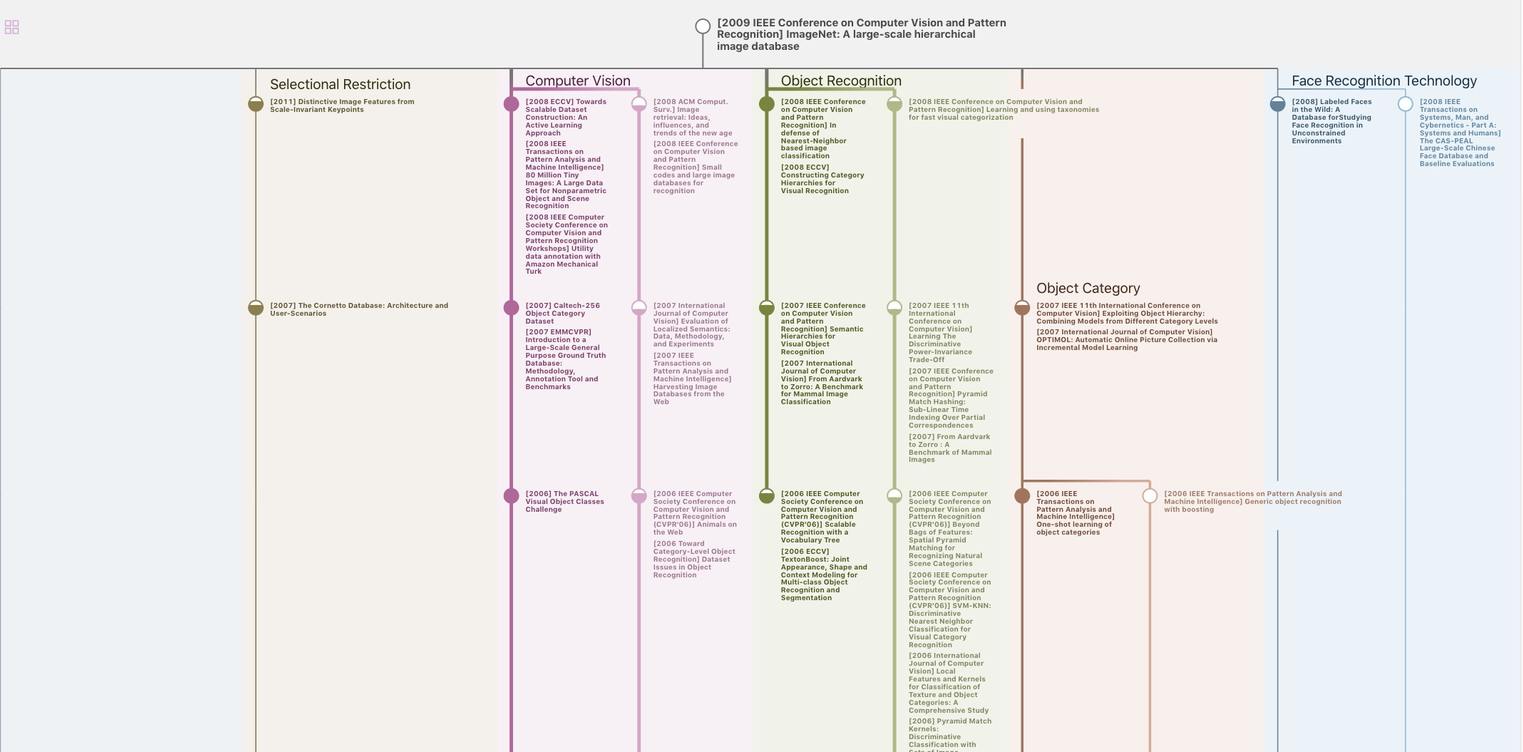
生成溯源树,研究论文发展脉络
Chat Paper
正在生成论文摘要