State and Parameter Estimation in Dynamic Real-time Optimization with Closed-Loop Prediction
Computer-aided chemical engineering(2023)
摘要
In this paper, we propose the integration of a state and parameter estimation step with closed-loop dynamic real-time optimization (CL-DRTO). Different from other dynamic real-time optimization strategies, CL-DRTO uses a dynamic model that considers the closed-loop response of the plant under the action of a controller. So far, plant feedback has been incorporated into CL-DRTO using an additive noise paradigm by a bias updating strategy. In this work, we perform the feedback step in CL-DRTO updating model states and parameters via Moving Horizon Estimation. As an additional contribution, we compare CL-DRTO with two other production optimization schemes, Steady-state Real-time Optimization (SRTO) and Real-time Optimization with Persistent Parameter Adaptation (ROPA). In the latter, a dynamic model is used for only the state and parameter estimation step in SRTO, eliminating the requirement for the plant to be at steady state prior to the economic optimization execution. We show that CL-DRTO outperforms the other two schemes in terms of economic and control performance when applied to systems under control performance limitations.
更多查看译文
关键词
parameter estimation,optimization,prediction,real-time,closed-loop
AI 理解论文
溯源树
样例
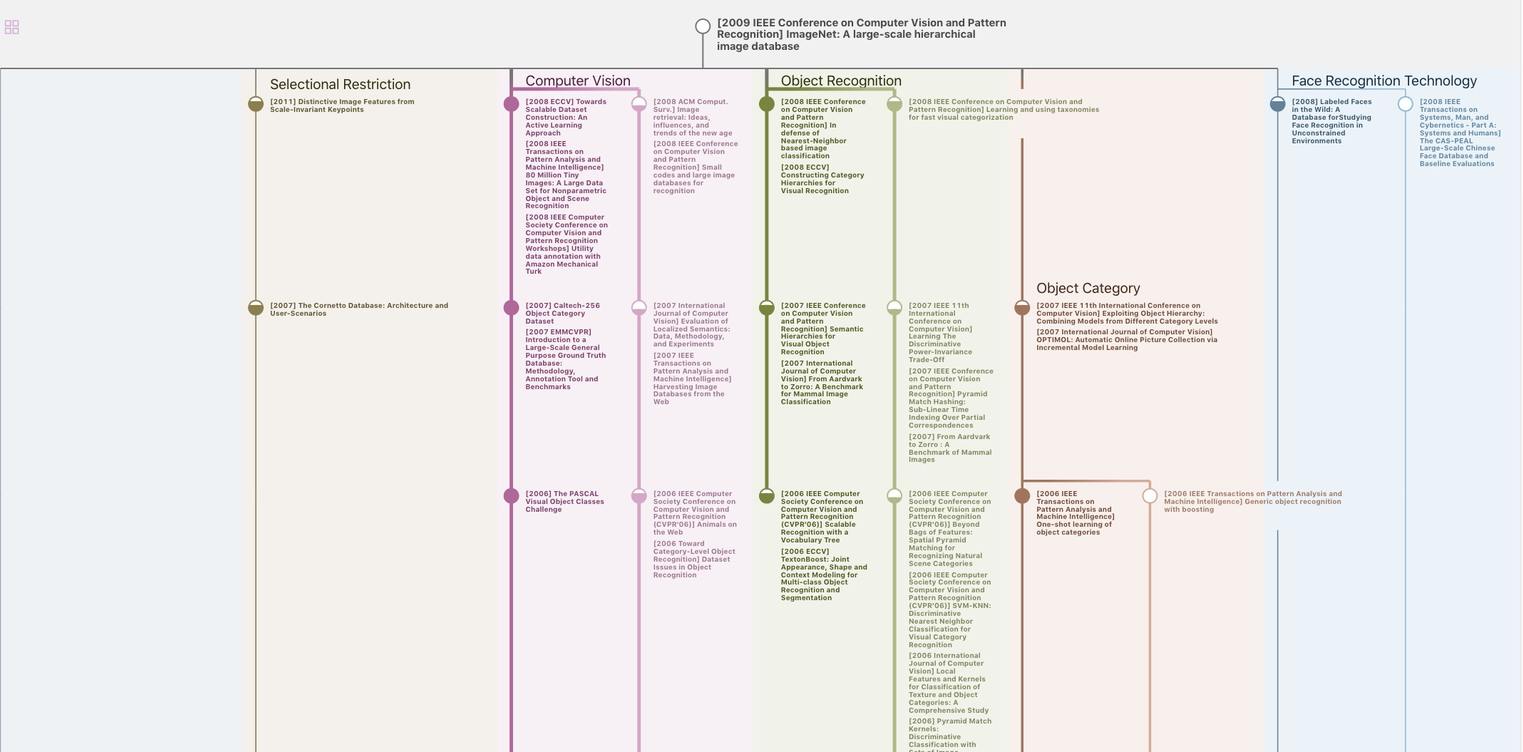
生成溯源树,研究论文发展脉络
Chat Paper
正在生成论文摘要