A Machine Learning Parameterization of Clouds in a Coarse-Resolution Climate Model for Unbiased Radiation
JOURNAL OF ADVANCES IN MODELING EARTH SYSTEMS(2024)
摘要
Coarse-grid weather and climate models rely particularly on parameterizations of cloud fields, and coarse-grained cloud fields from a fine-grid reference model are a natural target for a machine-learned parameterization. We machine-learn the coarsened-fine cloud properties as a function of coarse-grid model state in each grid cell of NOAA's FV3GFS global atmosphere model with 200 km grid spacing, trained using a 3 km fine-grid reference simulation with a modified version of FV3GFS. The ML outputs are coarsened-fine fractional cloud cover and liquid and ice cloud condensate mixing ratios, and the inputs are coarse model temperature, pressure, relative humidity, and ice cloud condensate. The predicted fields are skillful and unbiased, but somewhat under-dispersed, resulting in too many partially cloudy model columns. When the predicted fields are applied diagnostically (offline) in FV3GFS's radiation scheme, they lead to small biases in global-mean top-of-atmosphere (TOA) and surface radiative fluxes. An unbiased global-mean TOA net radiative flux is obtained by setting to zero any predicted cloud with grid-cell mean cloud fraction less than a threshold of 6.5%; this does not significantly degrade the ML prediction of cloud properties. The diagnostic, ML-derived radiative fluxes are far more accurate than those obtained with the existing cloud parameterization in the nudged coarse-grid model, as they leverage the accuracy of the fine-grid reference simulation's cloud properties. Weather and climate models typically use simplified means of predicting clouds, and these methods are particularly important for models that run at coarse resolution (200 km pixels) and relatively quickly. Machine learning is a natural way to improve upon the methods of predicting cloud. We first show that detailed cloud information from an accurate model run at much finer resolution (3 km pixels) can produce more accurate radiative energy fluxes at earth's surface and top of atmosphere when used in the coarse model, instead of its own predicted clouds. We show that by training on the temperature, humidity, and other characteristics of the coarse model, machine learning can replicate much of this skill in predicting clouds, and provide better radiation estimates than traditional methods. Because solar and thermal radiation is sensitive to small amounts of cloud, we find that we need to remove small amounts erroneously predicted by machine learning to achieve the right amounts of radiation at earth's surface and top of atmosphere. The machine-learning based approach could be used as a replacement for the current statistical methods of predicting cloud fields in fast, coarse-resolution weather and climate models. Fine-grid model clouds, when coarsened, can produce more accurate radiative fluxes than a coarse model's own parameterized clouds Machine learning can capture the coarsened fine-grid clouds as functions of coarse model state (temperature, pressure, and humidity) With appropriate post-processing, the machine-learned clouds also outperform the coarse model's clouds in terms of diagnostic radiation
更多查看译文
关键词
global climate models,machine learning,radiation scheme,cloud parameterization,subgrid-scale clouds
AI 理解论文
溯源树
样例
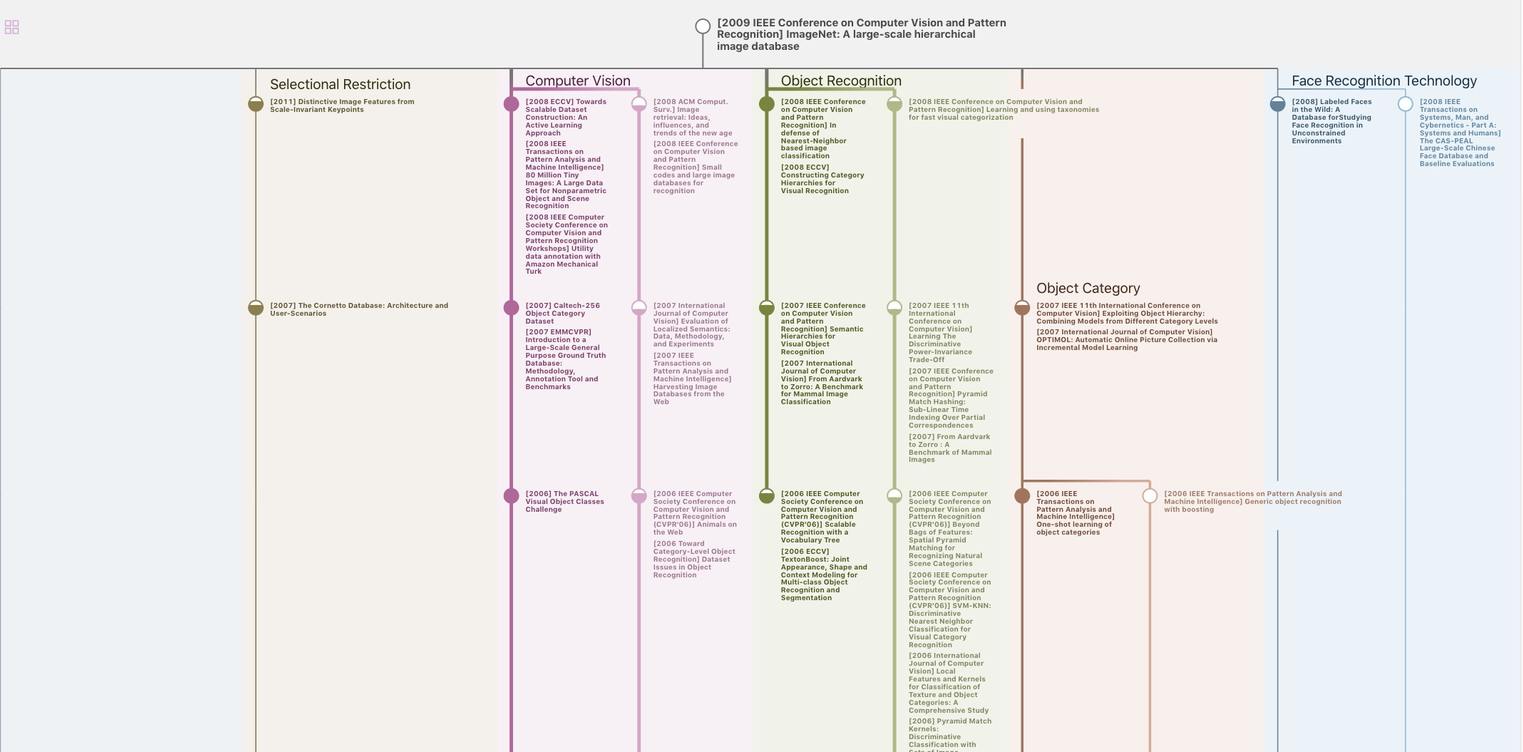
生成溯源树,研究论文发展脉络
Chat Paper
正在生成论文摘要