Computation and Communication Efficient Federated Learning over Wireless Networks
arXiv (Cornell University)(2023)
摘要
Federated learning (FL) enables distributed learning across edge devices while protecting data privacy. However, the learning accuracy decreases due to the heterogeneity of devices' data, and the computation and communication latency increase when updating large-scale learning models on devices with limited computational capability and wireless resources. We consider a novel FL framework with partial model pruning and personalization to overcome these challenges. This framework splits the learning model into a global part with model pruning shared with all devices to learn data representations and a personalized part to be fine-tuned for a specific device, which adapts the model size during FL to reduce both computation and communication latency and increases the learning accuracy for the device with non-independent and identically distributed (non-IID) data. Then, the computation and communication latency and the convergence analysis of the proposed FL framework are mathematically analyzed. To maximize the convergence rate and guarantee learning accuracy, Karush Kuhn Tucker (KKT) conditions are deployed to jointly optimize the pruning ratio and bandwidth allocation. Finally, experimental results demonstrate that the proposed FL framework achieves a remarkable reduction of approximately 50 percents computation and communication latency compared with the scheme only with model personalization.
更多查看译文
关键词
communication efficient federated learning,networks,wireless
AI 理解论文
溯源树
样例
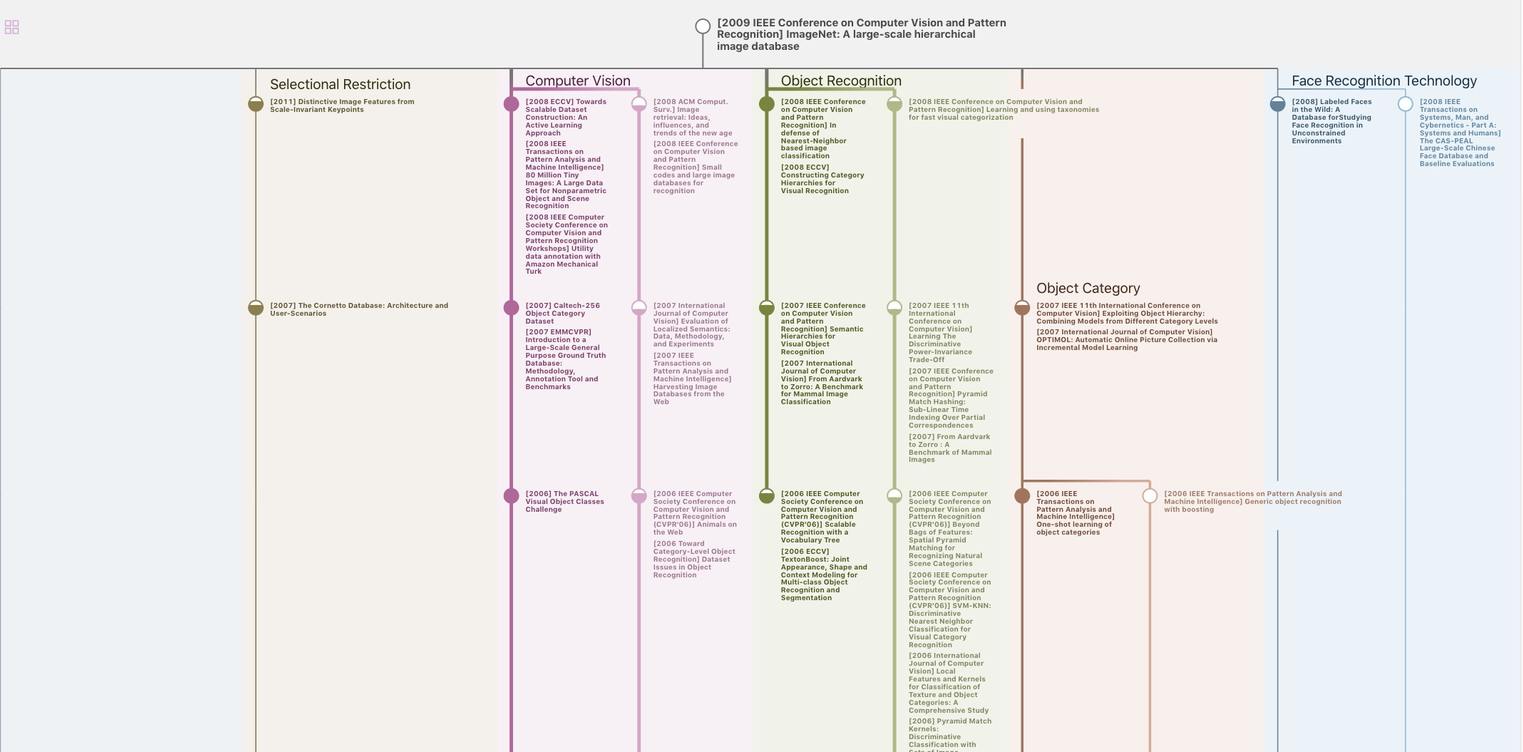
生成溯源树,研究论文发展脉络
Chat Paper
正在生成论文摘要